A Novel Hybrid GOA-XGB Model for Estimating Wheat Aboveground Biomass Using UAV-Based Multispectral Vegetation Indices
REMOTE SENSING(2022)
摘要
The rapid and nondestructive determination of wheat aboveground biomass (AGB) is important for accurate and efficient agricultural management. In this study, we established a novel hybrid model, known as extreme gradient boosting (XGBoost) optimization using the grasshopper optimization algorithm (GOA-XGB), which could accurately determine an ideal combination of vegetation indices (VIs) for simulating wheat AGB. Five multispectral bands of the unmanned aerial vehicle platform and 56 types of VIs obtained based on the five bands were used to drive the new model. The GOA-XGB model was compared with many state-of-the-art models, for example, multiple linear regression (MLR), multilayer perceptron (MLP), gradient boosting decision tree (GBDT), Gaussian process regression (GPR), random forest (RF), support vector machine (SVM), XGBoost, SVM optimization by particle swarm optimization (PSO), SVM optimization by the whale optimization algorithm (WOA), SVM optimization by the GOA (GOA-SVM), XGBoost optimization by PSO, XGBoost optimization by the WOA. The results demonstrated that MLR and GOA-MLR models had poor prediction accuracy for AGB, and the accuracy did not significantly improve when input factors were more than three. Among single-factor-driven machine learning (ML) models, the GPR model had the highest accuracy, followed by the XGBoost model. When the input combinations of multispectral bands and VIs were used, the GOA-XGB model (having 37 input factors) had the highest accuracy, with RMSE = 0.232 kg m(-2), R-2 = 0.847, MAE = 0.178 kg m(-2), and NRMSE = 0.127. When the XGBoost feature selection was used to reduce the input factors to 16, the model accuracy improved further to RMSE = 0.226 kg m(-2), R-2 = 0.855, MAE = 0.172 kg m(-2), and NRMSE = 0.123. Based on the developed model, the average AGB of the plot was 1.49 +/- 0.34 kg.
更多查看译文
关键词
metaheuristic optimization algorithm, feature selection, XGBoost, Gaussian process regression, aboveground biomass mapping
AI 理解论文
溯源树
样例
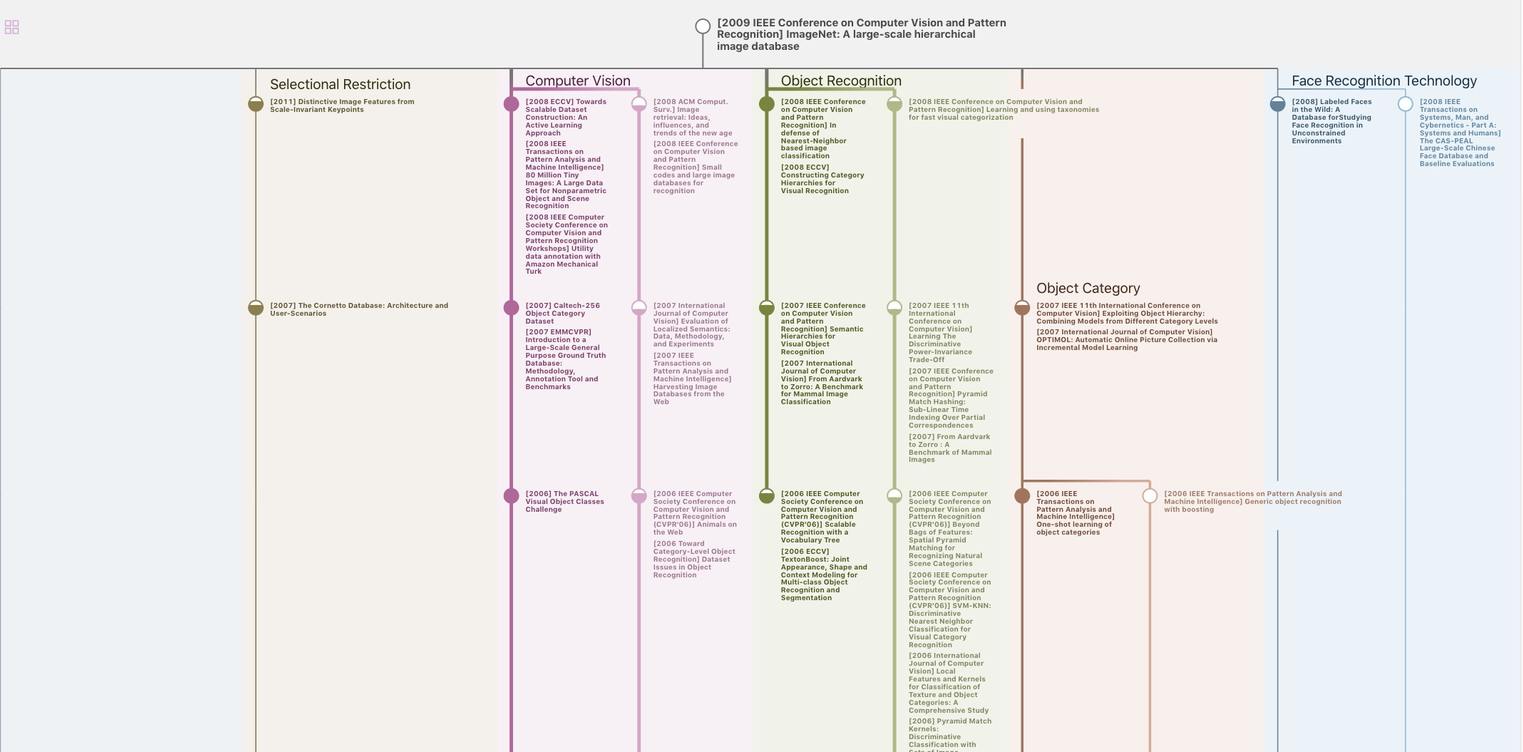
生成溯源树,研究论文发展脉络
Chat Paper
正在生成论文摘要