RGB-D Inertial Odometry for a Resource-Restricted Robot in Dynamic Environments
IEEE ROBOTICS AND AUTOMATION LETTERS(2022)
摘要
Current simultaneous localization and mapping (SLAM) algorithms perform well in static environments but easily fail in dynamic environments. Recent works introduce deep learning-based semantic information to SLAM systems to reduce the influence of dynamic objects. However, it is still challenging to apply a robust localization in dynamic environments for resource-restricted robots. This paper proposes a real-time RGB-D inertial odometry system for resource-restricted robots in dynamic environments named Dynamic-VINS. Three main threads run in parallel: object detection, feature tracking, and state optimization. The proposed Dynamic-VINS combines object detection and depth information for dynamic feature recognition and achieves performance comparable to semantic segmentation. Dynamic-VINS adopts grid-based feature detection and proposes a fast and efficient method to extract high-quality FAST feature points. IMU is applied to predict motion for feature tracking and moving consistency check. The proposed method is evaluated on both public datasets and real-world applications and shows competitive localization accuracy and robustness in dynamic environments. Yet, to the best of our knowledge, it is the best-performance real-time RGB-D inertial odometry for resource-restricted platforms in dynamic environments for now. The proposed system is open source at:
https://github.com/HITSZ-NRSL/Dynamic-VINS.git
更多查看译文
关键词
Localization, visual-inertial SLAM
AI 理解论文
溯源树
样例
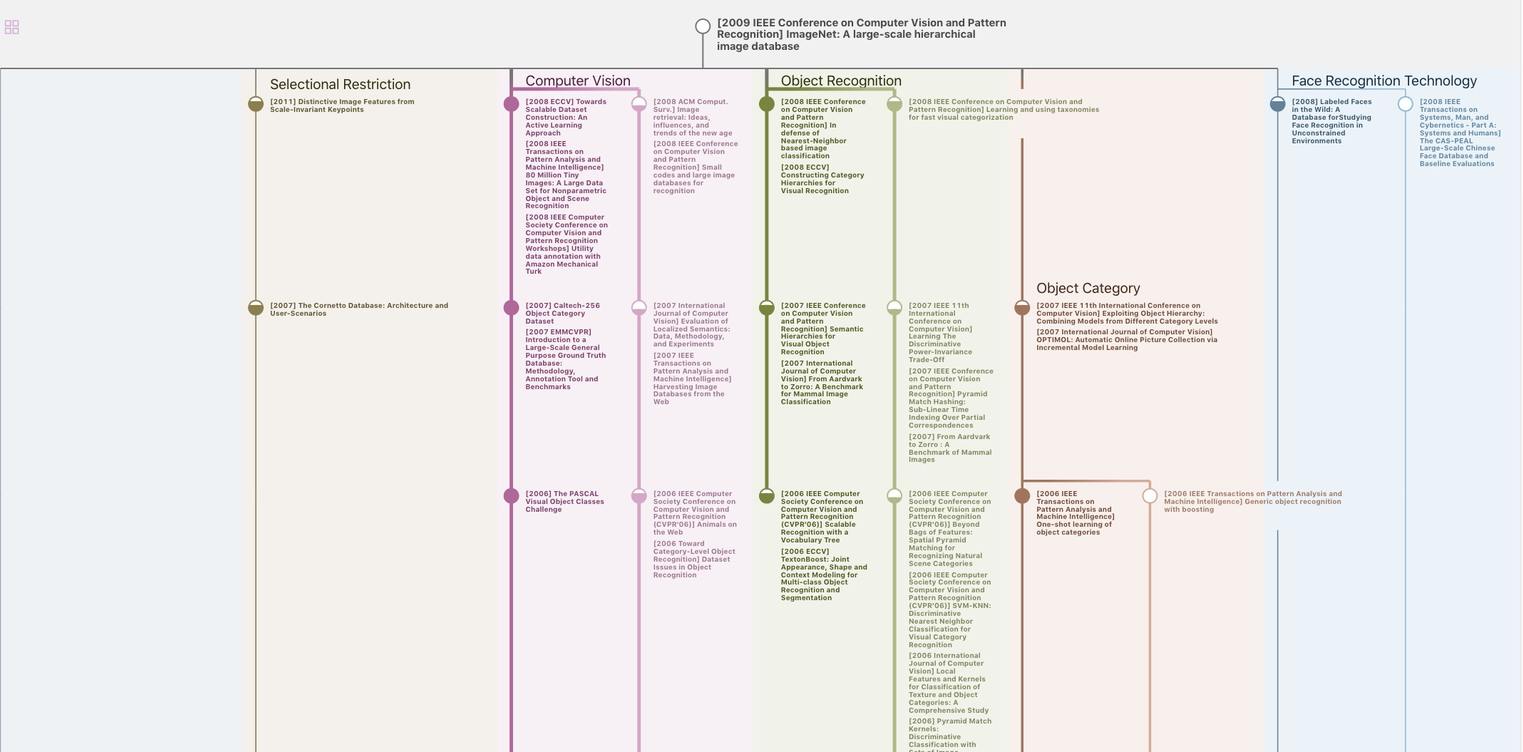
生成溯源树,研究论文发展脉络
Chat Paper
正在生成论文摘要