Automated detection of panic disorder based on multimodal physiological signals using machine learning
ETRI JOURNAL(2023)
摘要
We tested the feasibility of automated discrimination of patients with panic disorder (PD) from healthy controls (HCs) based on multimodal physiological responses using machine learning. Electrocardiogram (ECG), electrodermal activity (EDA), respiration (RESP), and peripheral temperature (PT) of the participants were measured during three experimental phases: rest, stress, and recovery. Eleven physiological features were extracted from each phase and used as input data. Logistic regression (LoR), k-nearest neighbor (KNN), support vector machine (SVM), random forest (RF), and multilayer perceptron (MLP) algorithms were implemented with nested cross-validation. Linear regression analysis showed that ECG and PT features obtained in the stress and recovery phases were significant predictors of PD. We achieved the highest accuracy (75.61%) with MLP using all 33 features. With the exception of MLP, applying the significant predictors led to a higher accuracy than using 24 ECG features. These results suggest that combining multimodal physiological signals measured during various states of autonomic arousal has the potential to differentiate patients with PD from HCs.
更多查看译文
关键词
anxiety disorder, autonomic nervous system (ANS) response, deep learning, electrocardiogram (ECG), heart rate variability (HRV), machine learning, mental stress task, multimodal, panic disorder, physiological signals
AI 理解论文
溯源树
样例
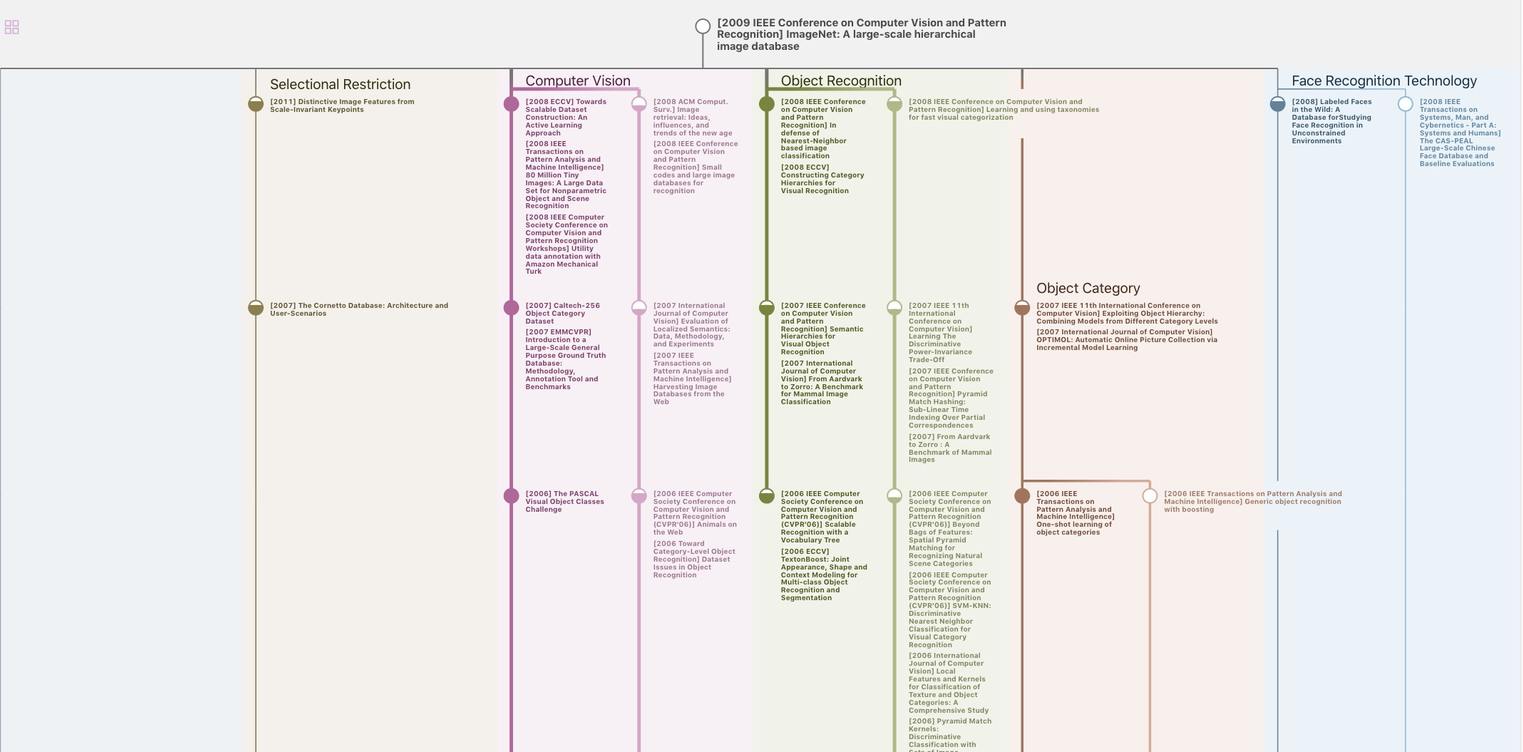
生成溯源树,研究论文发展脉络
Chat Paper
正在生成论文摘要