An Outlier Detection and Feature Ranking based Ensemble Learning for ECG Analysis
INTERNATIONAL JOURNAL OF ADVANCED COMPUTER SCIENCE AND APPLICATIONS(2022)
摘要
Automated classification of each heartbeat class from the ECG signal is important to diagnose cardiovascular diseases (CVDs) more quickly. ECG data acquired from the real -time or clinical databases contains exceptional values or extreme values called outliers. The separation and removal of outliers is very much useful for improving the data quality. The presence of outliers will influence the results of machine learning (ML) methods such as classification and regression. Outlier identification and removal plays a significant role in this area of research and is a part of signal denoising. Also, most of the traditional ECG-signal processing methods are facing the difficulty in finding the essential key features of recorded signal. In this work, an extreme outlier detection technique known as improved inter quartile range (IIQR) filtering method is used to find the outliers of the signal for the feature ranking process. In addition, an optimized random forest (ORF) based heterogenous ensemble classification model is proposed to improve the true positive and runtime on the ECG data. The classification of each heartbeat type is classified with majority voting technique. Ensemble learning and majority voting rule is used to enhance the accuracy of heart disease prediction. The proposed feature ranking based ORF ensemble classification model (LR + SVM + ORF + XGBoost + KNN) is evaluated on the MITBIH arrhythmia database and produces an overall accuracy of 99.45% which significantly outperforms the state-of-the-art methods such as, (LR + SVM + RF + XGBoost + KNN) with 96.17% accuracy, ensemble deep learning accuracy of 95.81% and ensemble SVM accuracy of 94.47%.
更多查看译文
关键词
Feature ranking, improved inter quartile range, majority voting, outlier detection, optimized random forest
AI 理解论文
溯源树
样例
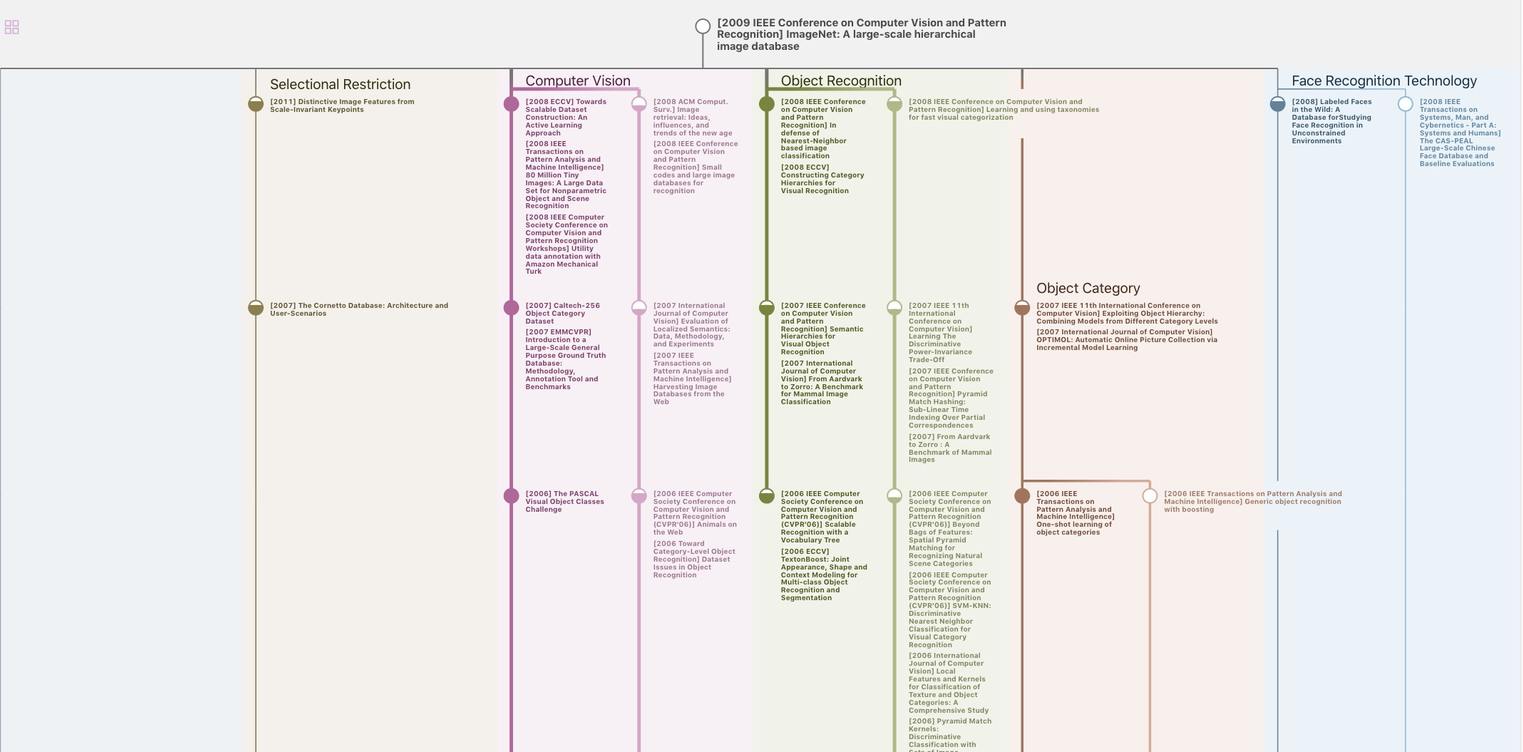
生成溯源树,研究论文发展脉络
Chat Paper
正在生成论文摘要