Abnormal Event Detection using Additive Summarization Model for Intelligent Transportation Systems
INTERNATIONAL JOURNAL OF ADVANCED COMPUTER SCIENCE AND APPLICATIONS(2022)
摘要
Video surveillance is used for capturing the abnormal events on roadsides that are caused due to improper driving, accidents, and hindrances resulting in transportation lags and life-critical issues. It is essential to highlight the accident keyframes in videos to achieve intelligent video surveillance. Video summarization plays a vital role in summarizing the keyframe for an abnormal event from the stacked video surveillance input. The observed video is converted into frames and analyzed for providing an accurate summarization for accident analysis forecast and guiding the users in avoiding such events. The main issues in summarization arise from the inconsistency between the spatiotemporal redundancies and the classification of sequence verification in video surveillance. This article introduces an Additive Event Summarization Method (AESM) for projecting classified events through a gated recurrent unit learning paradigm. In this process, the gates are assigned for unclassified and active frames for sequence verification. Based on the sequence, the abnormality is classified and summarized with higher accuracy than the state of art techniques. This proposed method relies on heterogeneous features for classifying events with better structural indices. The proposed method's performance is analyzed using the metrics accuracy, false rate, analysis time, SSIM, and F1-Score.
更多查看译文
关键词
Event detection, gated recurrent unit, summarization, intelligent transportation system
AI 理解论文
溯源树
样例
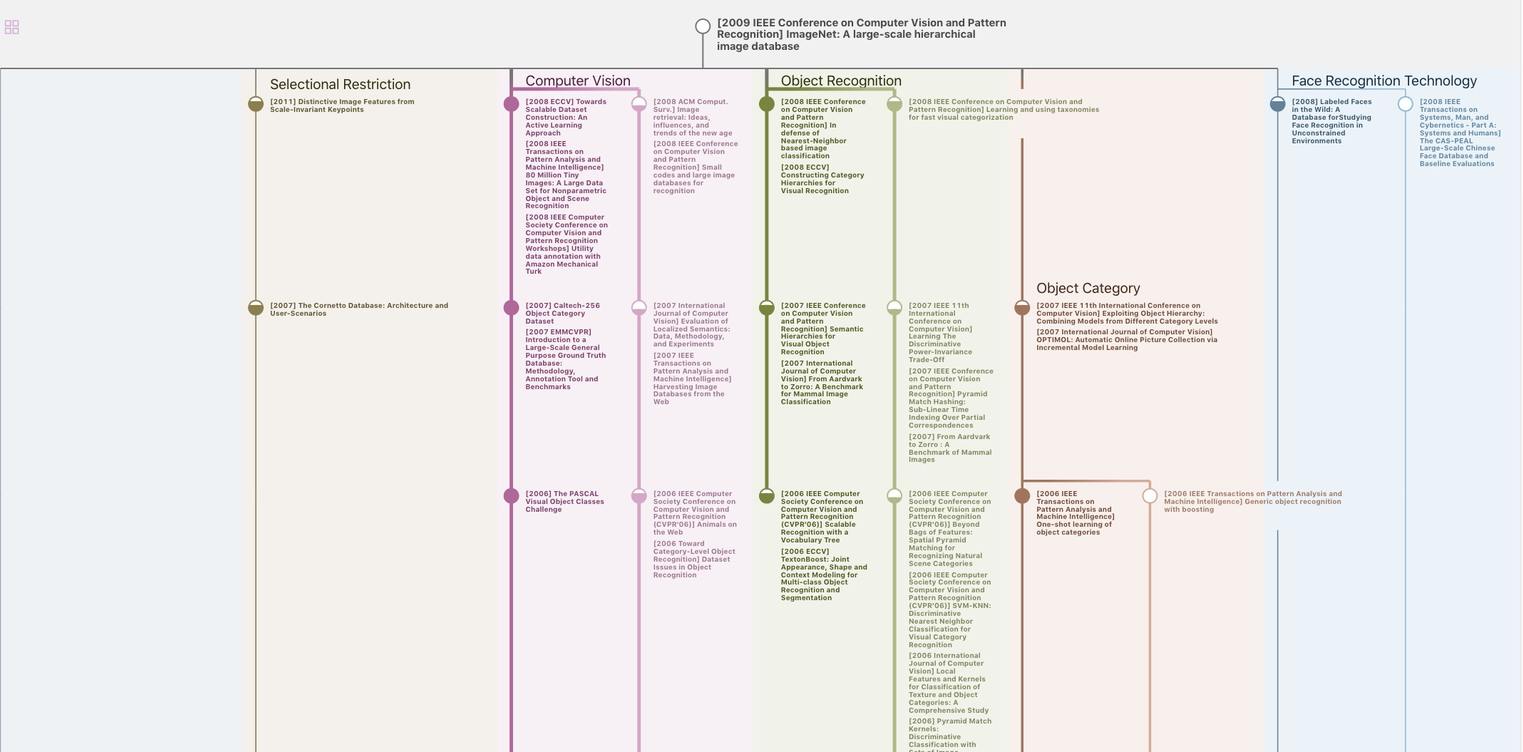
生成溯源树,研究论文发展脉络
Chat Paper
正在生成论文摘要