A Prestudy of Machine Learning in Industrial Quality Control Pipelines
INFORMATICA-AN INTERNATIONAL JOURNAL OF COMPUTING AND INFORMATICS(2022)
摘要
Today???s fast paced industrial production requires automation at multiple steps during its process. Involving humans during the quality control inspection provides high degree of confidence that the end products are with the best quality. Workers involved in the control process may have an impact on production capacity by lowering the throughput, depending on the complexity of the control process at the time the control is carried out, during the process which is a time-critical operation, or after the process is completed. Companies are striving to fully automate their quality control stages of production and it comes naturally to focus on using various machine learning methods to help build a quality control pipeline which will offer high throughput and high degree of quality. In this paper we give an overview of applying several machine learning approaches in order to achieve an autonomous quality control pipeline. The applications for these approaches were used to help improve the quality control pipeline of two of the biggest manufacturing companies in Slovenia. One of the most challenging part of the study was that the tests had to be performed only on a small number of defective products, as is in reality. The motivation was to test several methods to find the most promising one for later actual application. slovenskih podjetjih.
更多查看译文
关键词
machine learning, manufacturing, quality control, home appliances, car industry
AI 理解论文
溯源树
样例
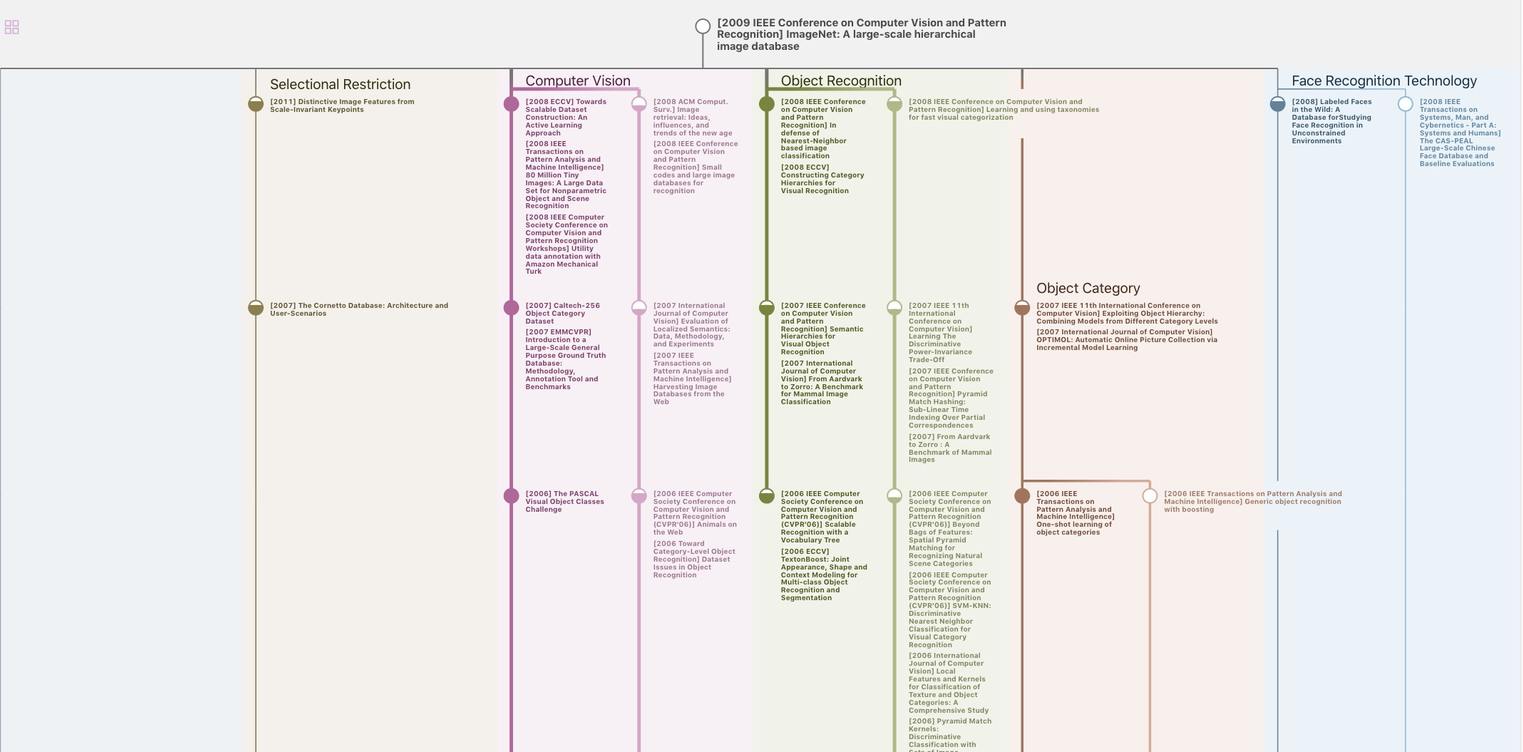
生成溯源树,研究论文发展脉络
Chat Paper
正在生成论文摘要