Transfer Learning from Healthy to Unhealthy Patients for the Automated Classification of Functional Brain Networks in fMRI
APPLIED SCIENCES-BASEL(2022)
摘要
Functional Magnetic Resonance Imaging (fMRI) is an essential tool for the pre-surgical planning of brain tumor removal, which allows the identification of functional brain networks to preserve the patient's neurological functions. One fMRI technique used to identify the functional brain network is the resting-state-fMRI (rs-fMRI). This technique is not routinely available because of the necessity to have an expert reviewer who can manually identify each functional network. The lack of sufficient unhealthy data has so far hindered a data-driven approach based on machine learning tools for full automation of this clinical task. In this article, we investigate the possibility of such an approach via the transfer learning method from healthy control data to unhealthy patient data to boost the detection of functional brain networks in rs-fMRI data. The end-to-end deep learning model implemented in this article distinguishes seven principal functional brain networks using fMRI images. The best performance of a 75% correct recognition rate is obtained from the proposed deep learning architecture, which shows its superiority over other machine learning algorithms that were equally tested for this classification task. Based on this best reference model, we demonstrate the possibility of boosting the results of our algorithm with transfer learning from healthy patients to unhealthy patients. This application of the transfer learning technique opens interesting possibilities because healthy control subjects can be easily enrolled for fMRI data acquisition since it is noninvasive. Consequently, this process helps to compensate for the usual small cohort of unhealthy patient data. This transfer learning approach could be extended to other medical imaging modalities and pathology.
更多查看译文
关键词
deep learning, transfer learning, resting-state fMRI, functional brain networks, image classification
AI 理解论文
溯源树
样例
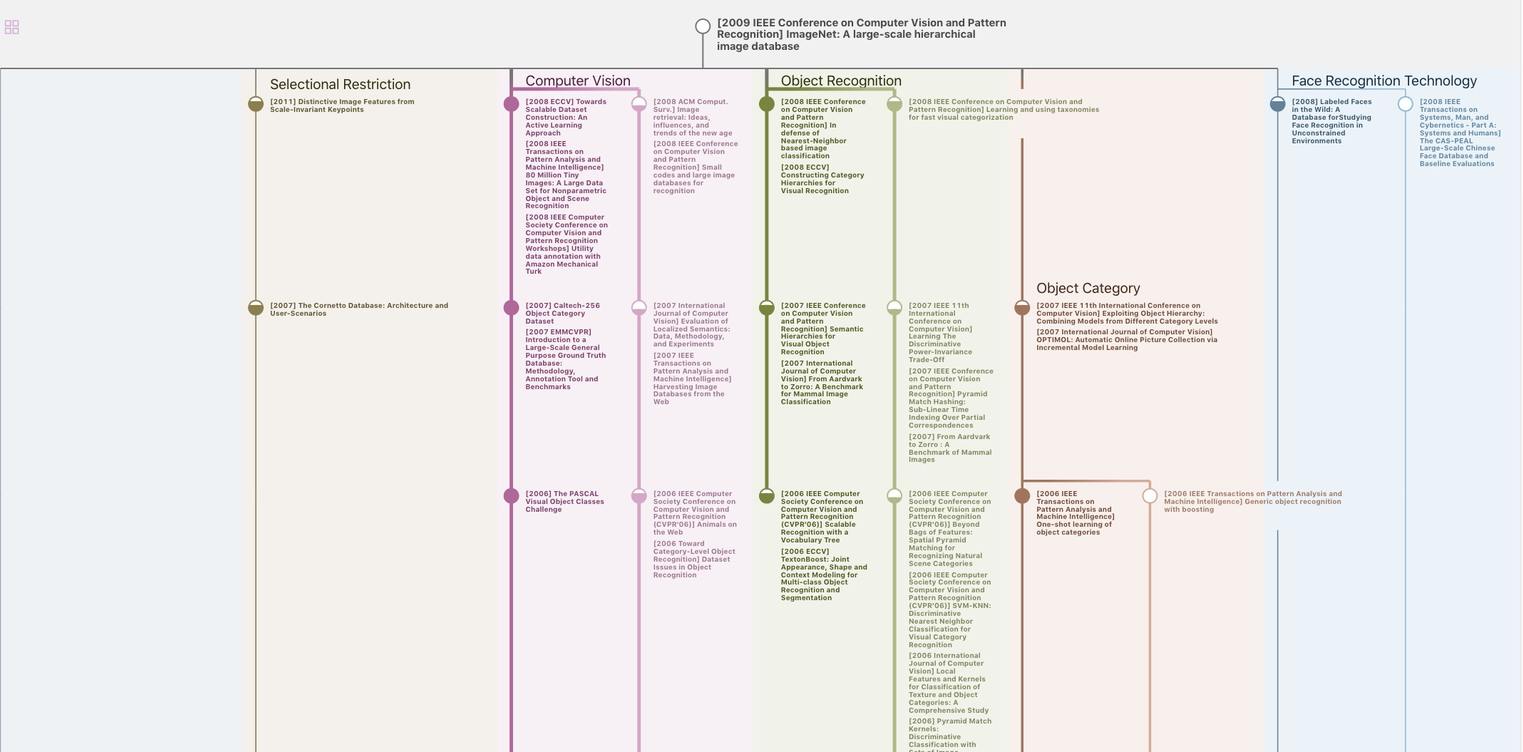
生成溯源树,研究论文发展脉络
Chat Paper
正在生成论文摘要