Predicting lead water service lateral locations: Geospatial data science in support of municipal programming
Socio-Economic Planning Sciences(2022)
摘要
We present and discuss machine learning predictions of customers' service line materials in Pittsburgh, PA and demonstrate the degree to which these predictions and the supporting data can and cannot improve municipal lead programming. Like previous work, predictive features reflect a combination of property characteristics, administrative spatial data, and tap water quality samples. Our work also includes labels of service line materials diagnosed by photographs taken at the curb box, which prove to boost predictions but are imperfect exclusive diagnostic methods. We use sample weighting and spatial cross validation in an effort to overcome the oversampling of lead service line characteristics of data collected for regulatory compliance. Cross-validation demonstrates precise predictions (precision >90%) for only 13% of customers, suggesting that predictions could improve short-term replacement decisions in avoiding unnecessary excavations. However, model precision declines when expanding predictions to more customers, limiting the degree to which predictions can estimate system-wide inventories and inform the regulatory decisions requiring complete inventories. We discuss the necessary trade-offs between biased sampling for regulatory compliance, which favors finding and replacing lead, and predictive modeling, which improves with unbiased sampling. We present a flow diagram that can help municipalities balance biased and unbiased sampling when integrating predictive modeling into compliance with federal regulations.
更多查看译文
关键词
Lead contamination,Drinking water,Machine learning
AI 理解论文
溯源树
样例
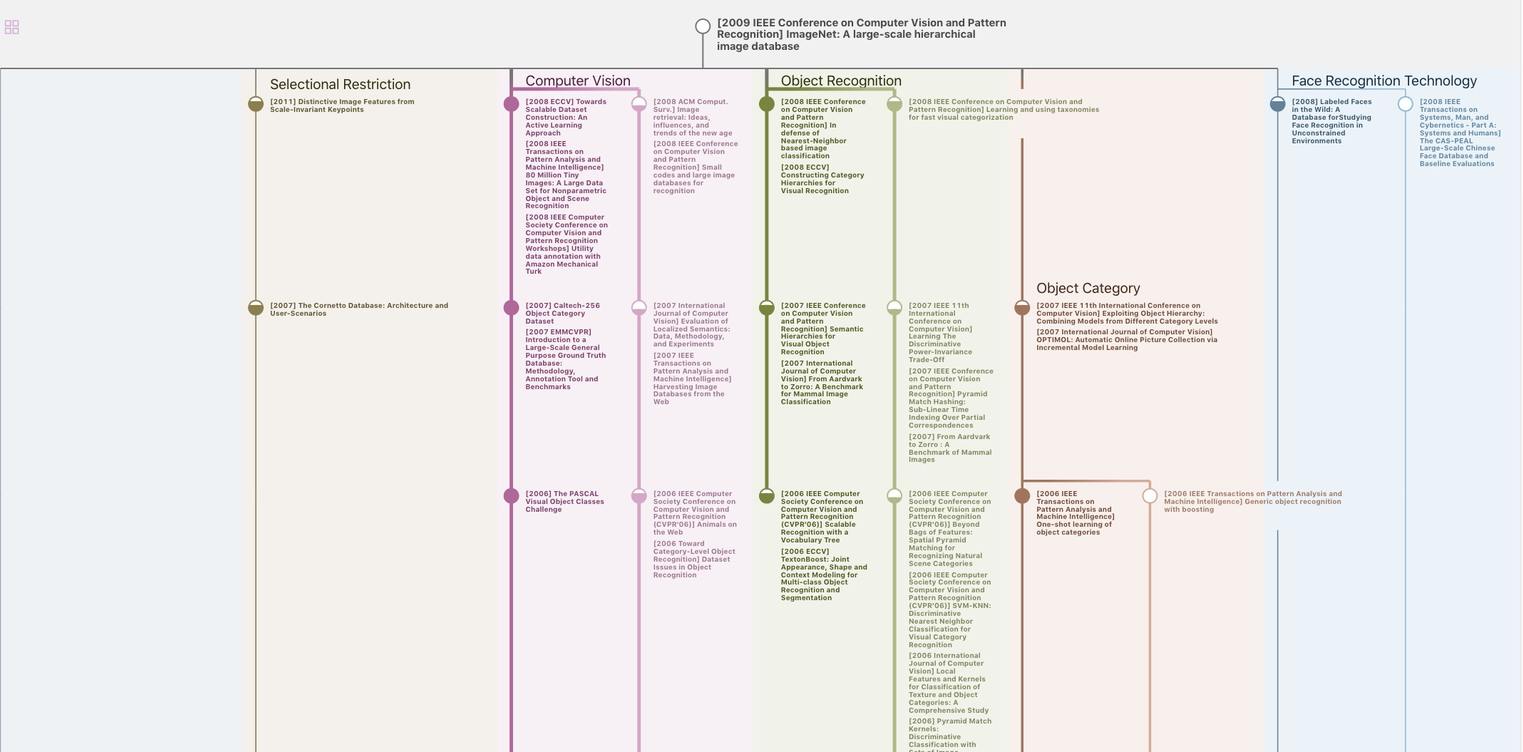
生成溯源树,研究论文发展脉络
Chat Paper
正在生成论文摘要