Optimized building extraction from high-resolution satellite imagery using deep learning
MULTIMEDIA TOOLS AND APPLICATIONS(2022)
摘要
Building extraction is very essential in various urban dynamics like disaster management and change detection, finding the estimated population, and so on. Building extraction from satellite data is a challenging task as the images may be subjected to different illumination or structure due to very large variations of the appearance of buildings which may correspond to the different area/terrain. Although satellite imagery is readily available from various sources, translating the imagery includes intensive effort. Many computer-vision tasks have been carried out successfully but understanding the impact of them on building extraction with remote sensing imagery is a growing need.To overcome this kind of problem, an algorithm is proposed which extends the convolutional neural network for pixel-wise classification of images. Furthermore, to resolve the problem of extraction and masking of images, Mask-RCNN (i.e., Mask Region-based Convolutional Neural Network) algorithm is used which makes this process easier and more efficient.The model is trained on a complex dataset that is significantly larger. Also, to make this algorithm more scalable, an advanced image augmentation technique is used in the pre-processing step.The results show that the algorithm achieves better performance in terms of accuracy.
更多查看译文
关键词
High-resolution remote sensing images,Deep learning,Deep convolutional networks,Building extraction,Mask-RCNN
AI 理解论文
溯源树
样例
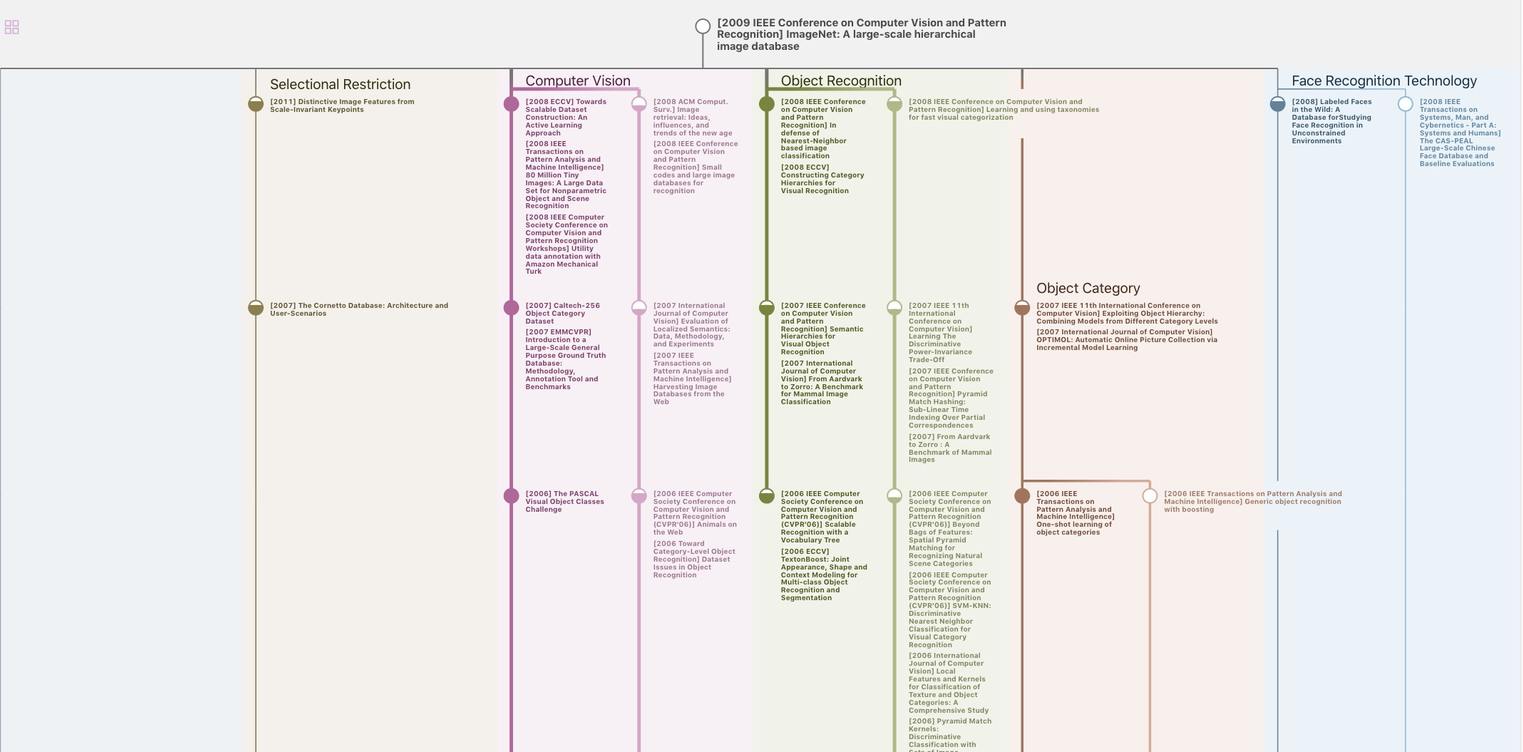
生成溯源树,研究论文发展脉络
Chat Paper
正在生成论文摘要