A neural-embedded discrete choice model: Learning taste representation with strengthened interpretability
Transportation Research Part B: Methodological(2022)
摘要
Discrete choice models (DCMs) require a priori knowledge of the utility functions, especially how tastes vary across individuals. Utility misspecification may lead to biased estimates, inaccurate interpretations and limited predictability. In this paper, we utilize a neural network to learn taste representation. Our formulation consists of two modules: a neural network (TasteNet) that learns taste parameters (e.g., time coefficient) as flexible functions of individual characteristics; and a multinomial logit (MNL) model with utility functions defined with expert knowledge. Taste parameters learned by the neural network are fed into the choice model and link the two modules.
更多查看译文
关键词
Discrete choice models,Neural networks,Taste heterogeneity,Interpretability,Utility specification,Machine learning,Deep learning
AI 理解论文
溯源树
样例
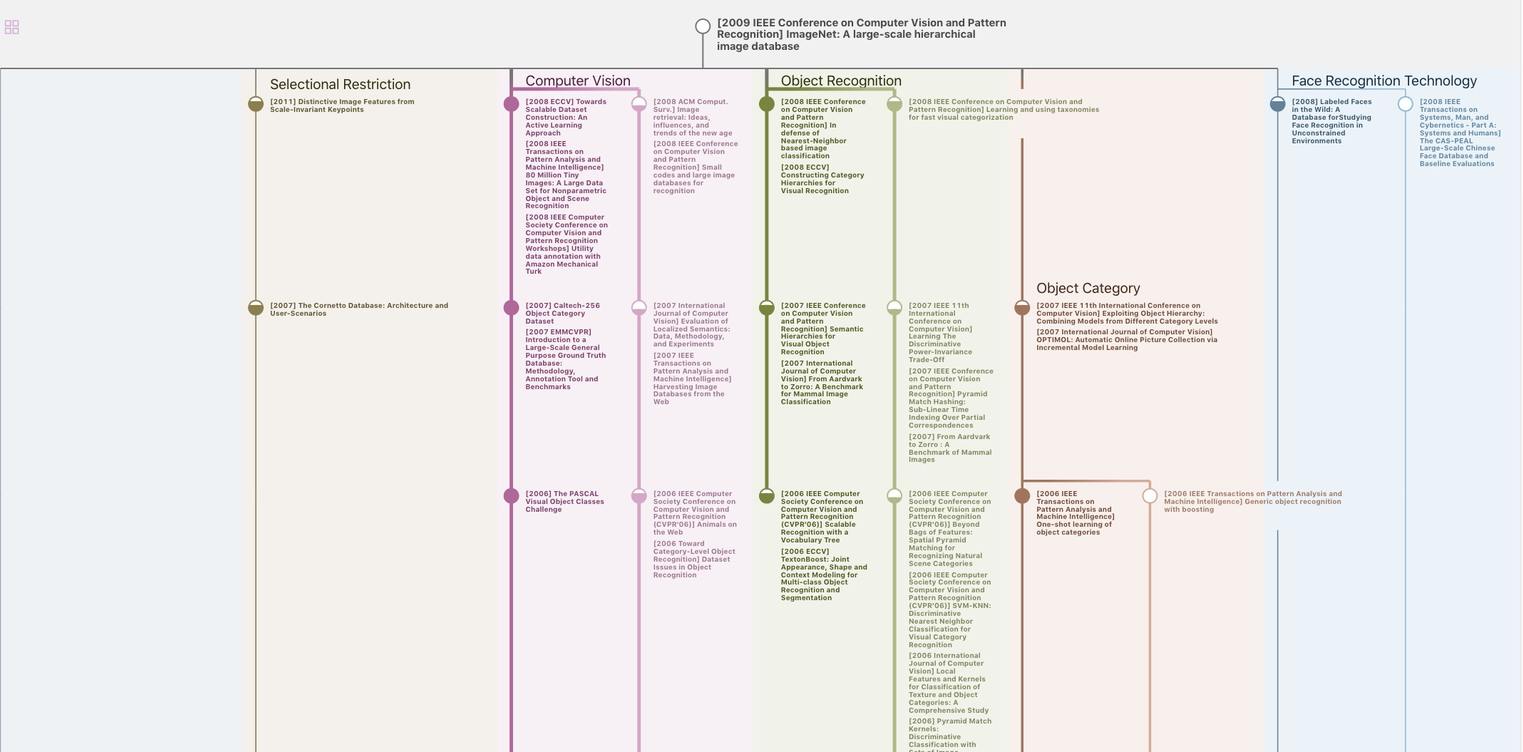
生成溯源树,研究论文发展脉络
Chat Paper
正在生成论文摘要