A Prior Level Fusion Approach for the Semantic Segmentation of 3D Point Clouds Using Deep Learning
REMOTE SENSING(2022)
摘要
Three-dimensional digital models play a pivotal role in city planning, monitoring, and sustainable management of smart and Digital Twin Cities (DTCs). In this context, semantic segmentation of airborne 3D point clouds is crucial for modeling, simulating, and understanding large-scale urban environments. Previous research studies have demonstrated that the performance of 3D semantic segmentation can be improved by fusing 3D point clouds and other data sources. In this paper, a new prior-level fusion approach is proposed for semantic segmentation of large-scale urban areas using optical images and point clouds. The proposed approach uses image classification obtained by the Maximum Likelihood Classifier as the prior knowledge for 3D semantic segmentation. Afterwards, the raster values from classified images are assigned to Lidar point clouds at the data preparation step. Finally, an advanced Deep Learning model (RandLaNet) is adopted to perform the 3D semantic segmentation. The results show that the proposed approach provides good results in terms of both evaluation metrics and visual examination with a higher Intersection over Union (96%) on the created dataset, compared with (92%) for the non-fusion approach.
更多查看译文
关键词
3D point cloud, aerial images, semantic segmentation, data fusion, deep learning
AI 理解论文
溯源树
样例
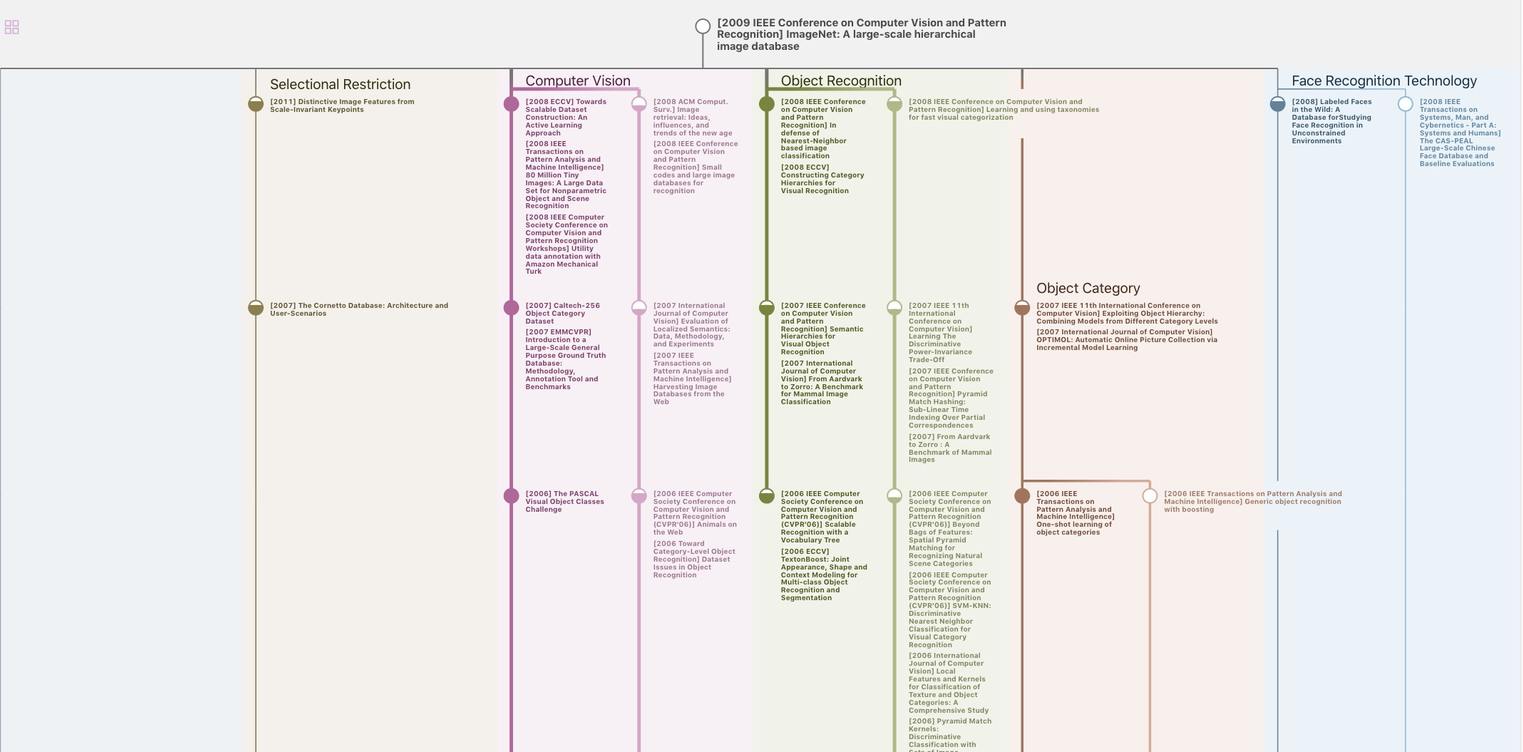
生成溯源树,研究论文发展脉络
Chat Paper
正在生成论文摘要