A Grant-Free Random Access Process for Low-End Distribution System Using Deep Neural Network
APPLIED SCIENCES-BASEL(2022)
摘要
With the rising number of Internet of Things (IoT) devices joining the communication network, data exchange is increased tremendously resulting in network congestion. This paper deals with the optimal transmission of IoT devices to maximize the chances of success in random access procedures. With every machine trying to use the network for the transfer of data, IoT devices pose serious challenges to the already deployed infrastructure network. With a huge number of IoT devices and fixed limited resources, the existing handshaking-based random access process is not effective. To address this research gap, we propose a grant-free procedure while considering orthogonal transmission and devise a strategy to minimize collisions and idle events and maximize success. We use deep neural networks (DNN) that take channel conditions as an input to predict the device's transmission for a successful maximization. In order to evaluate the performance of our proposed algorithm, we calculated the average delay with respect to channel coefficient and arrival rate in addition to the number of successes against the channel coefficient. Simulation results show that the proposed algorithm performs well and conforms with the claim of a successful maximization.
更多查看译文
关键词
IoT, random access channel, grant-free transmission, machine learning, deep neural network
AI 理解论文
溯源树
样例
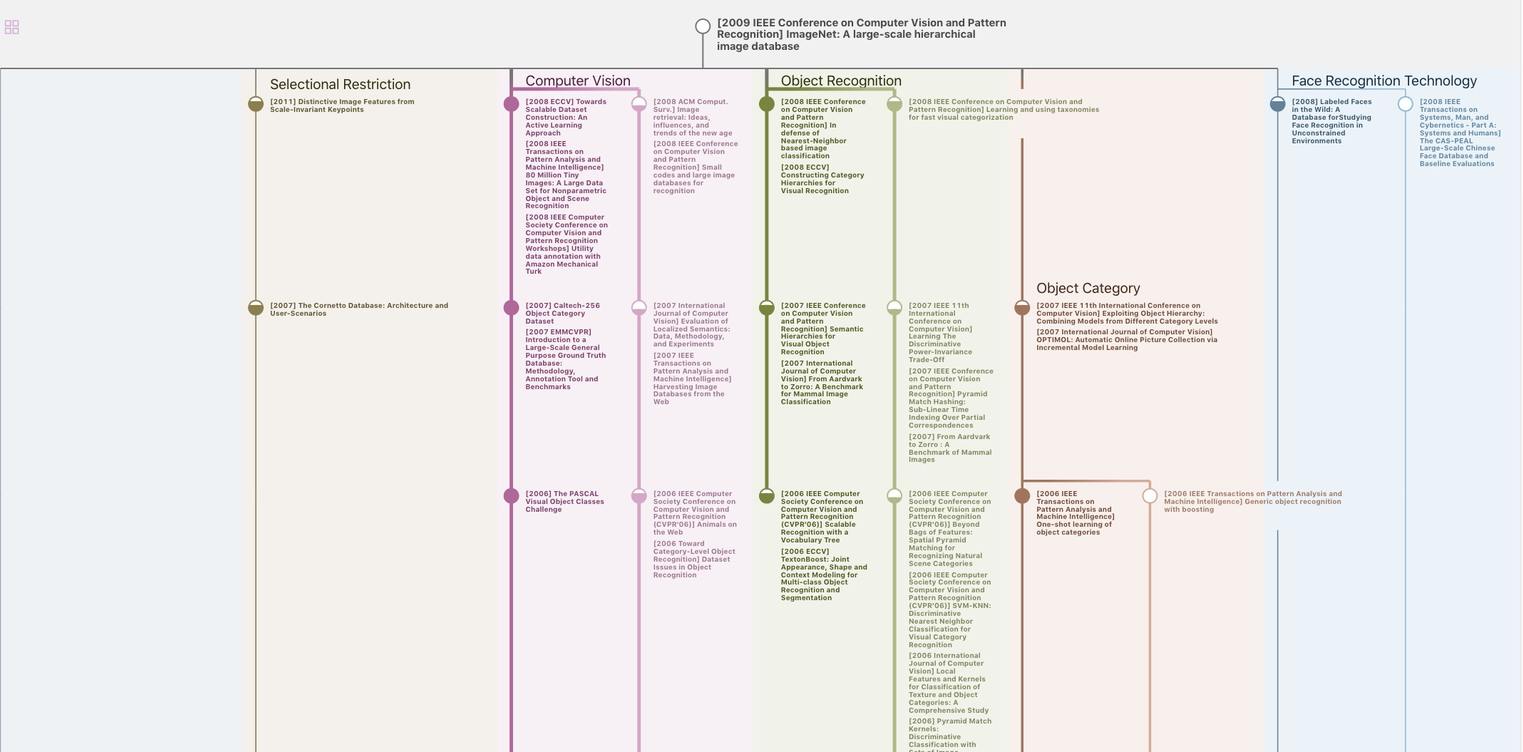
生成溯源树,研究论文发展脉络
Chat Paper
正在生成论文摘要