Optimization-enabled deep convolutional network for the generation of normal speech from non-audible murmur based on multi-kernel-based features
INTERNATIONAL JOURNAL OF WAVELETS MULTIRESOLUTION AND INFORMATION PROCESSING(2022)
摘要
Automatic Speech Recognition schemes are the alternating modes in which individuals interrelate with various mobile application applications. The user interactivity needs are a huge vocabulary identification system, high accuracy, energy-efficient solution and time. Although the automatic Speech Recognition system requires power budget and huge memory bandwidth, it is not applicable for many tiny forms of battery-controlled devices. Hence, an effective method is developed using the proposed Multi-kernel Perceptual linear predictive and Stochastic Biogeography-based whale optimization algorithm optimization to adapt non-audible mutter to regular speech. First, the input speech signal is initially given to the pre-processed module. Then, the features, such as spectral centroid, pitch chroma, Taylor amplitude modulation spectrogram (AMS), spectral skewness, and the developed Multi-kernel Perceptual linear predictive, are extracted to determine the appropriate features. After extracting features, the speech recognition is performed based on Deep Convolutional Neural Network, which is trained by the proposed Stochastic Biogeography whale optimization algorithm. The Stochastic Biogeography whale optimization algorithm combines the stochastic gradient descent method, whale optimization algorithm, and biogeography-based optimization. The developed model showed improved results with maximum accuracy of 0.985, minimal FPR of 0.001, maximal TPR of 1, respectively.
更多查看译文
关键词
Speech recognition, Multi-kernel PLP, stochastic gradient descent approach, deep convolutional neural network, biogeography-based optimization
AI 理解论文
溯源树
样例
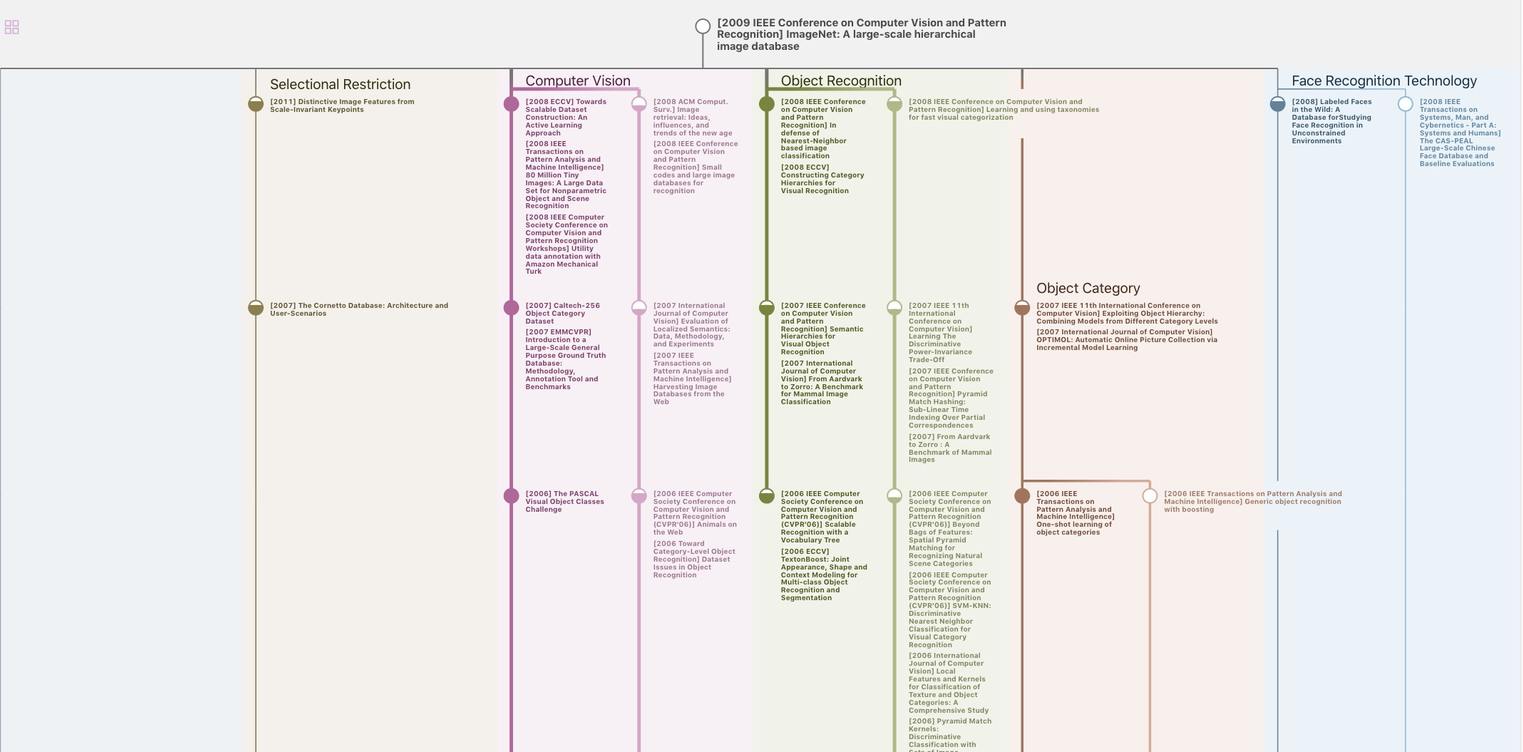
生成溯源树,研究论文发展脉络
Chat Paper
正在生成论文摘要