Electromyography-Based, Robust Hand Motion Classification Employing Temporal Multi-Channel Vision Transformers
IEEE ROBOTICS AND AUTOMATION LETTERS(2022)
摘要
With an increasing use of robotic and bionic devices for the execution of everyday life, complex tasks, Electromyography (EMG) based interfaces are being explored as candidate technologies for facilitating an intuitive interaction with such devices. However, EMG-based interfaces typically require appropriate features to be extracted from the raw EMG signals using a plethora of feature extraction methods to achieve excellent performance in practical applications. To select an appropriate feature set that will lead to significant EMG-based decoding performance, a deep understanding of available methods and the human musculoskeletal system is needed. To overcome this issue, researchers have proposed the use of deep learning methods for automatically extracting complex features directly from the raw EMG data. In this work, we propose Temporal Multi-Channel Vision Transformers as a deep learning technique that has the potential to achieve dexterous control of robots and bionic hands. The performance of this method is evaluated and compared with other well-known methods, employing the open-access Ninapro dataset.
更多查看译文
关键词
Deep learning methods, deep learning in grasping and manipulation, in-hand manipulation, prosthetics and exoskeletons
AI 理解论文
溯源树
样例
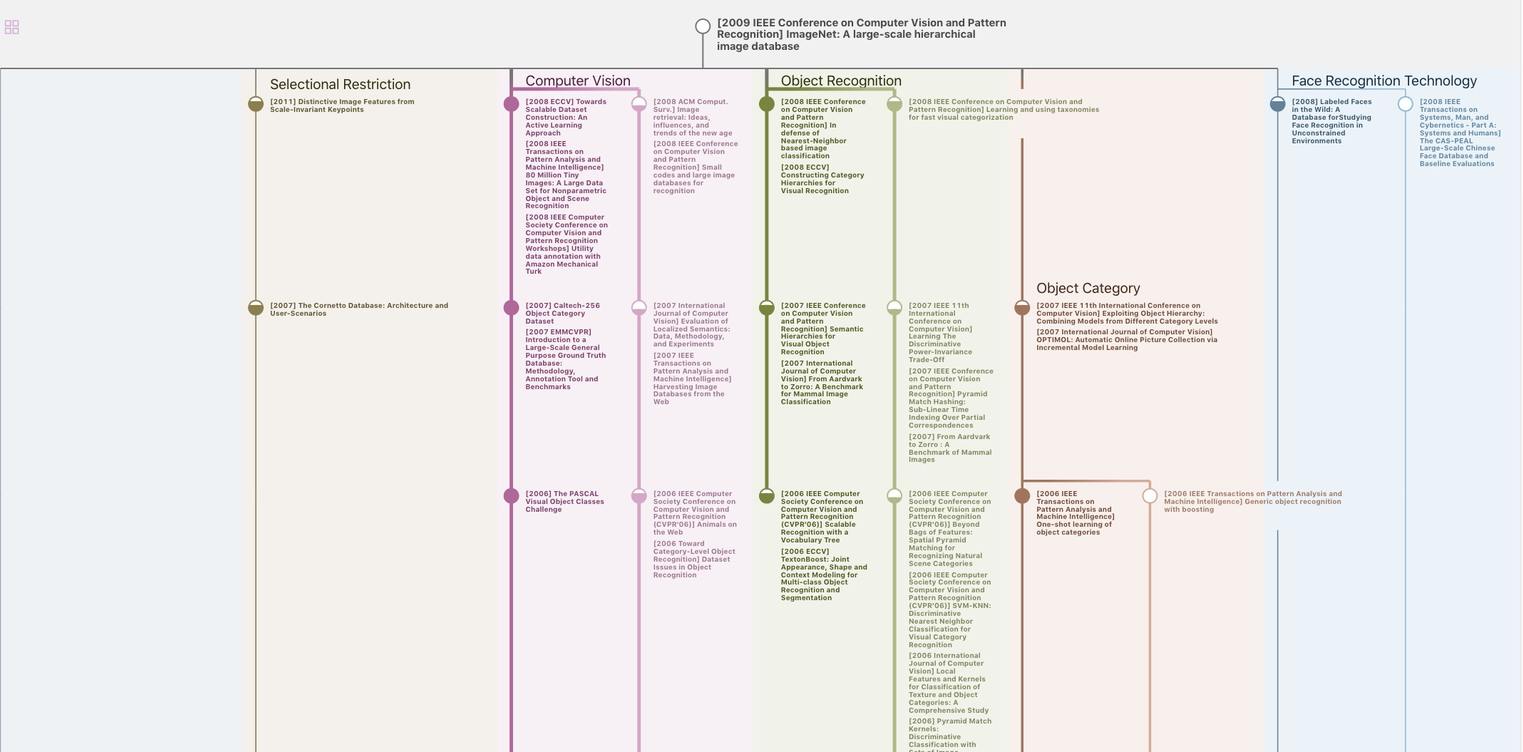
生成溯源树,研究论文发展脉络
Chat Paper
正在生成论文摘要