Multicalibrated Regression for Downstream Fairness
PROCEEDINGS OF THE 2023 AAAI/ACM CONFERENCE ON AI, ETHICS, AND SOCIETY, AIES 2023(2023)
摘要
We show how to take a regression function (f) over cap that is appropriately multicalibrated and efficiently post-process it into an approximately error minimizing classifier satisfying a large variety of fairness constraints. The post-processing requires no labeled data, and only a modest amount of unlabeled data and computation. The computational and sample complexity requirements of computing (f) over cap are comparable to the requirements for solving a single fair learning task optimally, but it can in fact be used to solve many different downstream fairness-constrained learning problems efficiently. Our post-processing method easily handles intersecting groups, generalizing prior work on post-processing regression functions to satisfy fairness constraints that only applied to disjoint groups. Our work extends recent work showing that multicalibrated regression functions are omnipredictors (i.e. can be post-processed to optimally solve unconstrained ERM problems) to constrained optimization problems.
更多查看译文
AI 理解论文
溯源树
样例
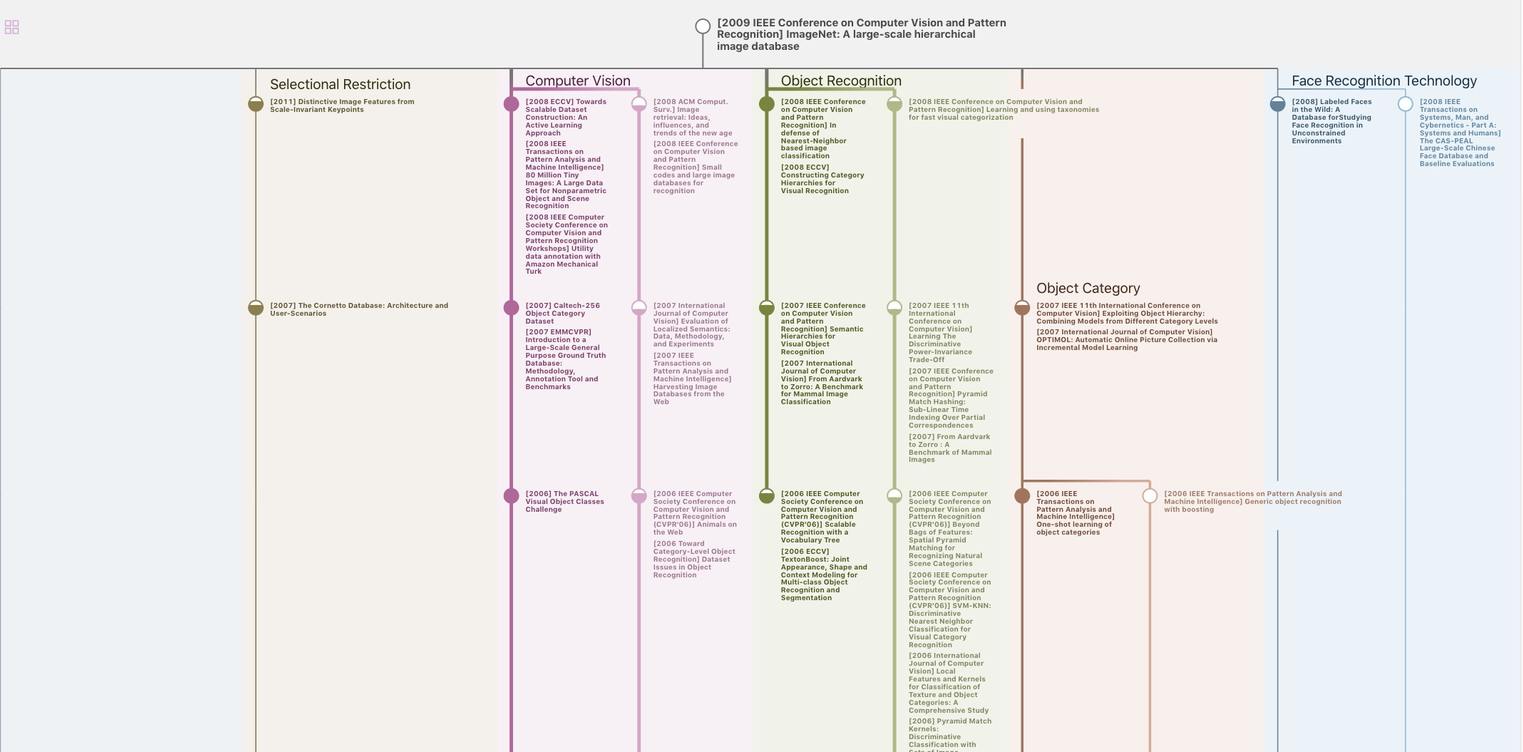
生成溯源树,研究论文发展脉络
Chat Paper
正在生成论文摘要