Structure preservation via the Wasserstein distance
arxiv(2022)
摘要
We show that under minimal assumptions on a random vector $X\in\mathbb{R}^d$, and with high probability, given $m$ independent copies of $X$, the coordinate distribution of each vector $(\langle X_i,\theta \rangle)_{i=1}^m$ is dictated by the distribution of the true marginal $\langle X,\theta \rangle$. Formally, we show that with high probability, \[\sup_{\theta \in S^{d-1}} \left( \frac{1}{m}\sum_{i=1}^m \left|\langle X_i,\theta \rangle^\sharp - \lambda^\theta_i \right|^2 \right)^{1/2} \leq c \left( \frac{d}{m} \right)^{1/4},\] where $\lambda^{\theta}_i = m\int_{(\frac{i-1}{m}, \frac{i}{m}]} F_{ \langle X,\theta \rangle }^{-1}(u)^2 \,du$ and $a^\sharp$ denotes the monotone non-decreasing rearrangement of $a$. The proof follows from the optimal estimate on the worst Wasserstein distance between a marginal of $X$ and its empirical counterpart, $\frac{1}{m} \sum_{i=1}^m \delta_{\langle X_i, \theta \rangle}$. We then use the accurate information on the structures of the vectors $(\langle X_i,\theta \rangle)_{i=1}^m$ to construct the first non-gaussian ensemble that yields the optimal estimate in the Dvoretzky-Milman Theorem: the ensemble exhibits almost Euclidean sections in arbitrary normed spaces of the same dimension as the gaussian embedding -- despite being very far from gaussian (in fact, it happens to be heavy-tailed).
更多查看译文
关键词
structure,preservation
AI 理解论文
溯源树
样例
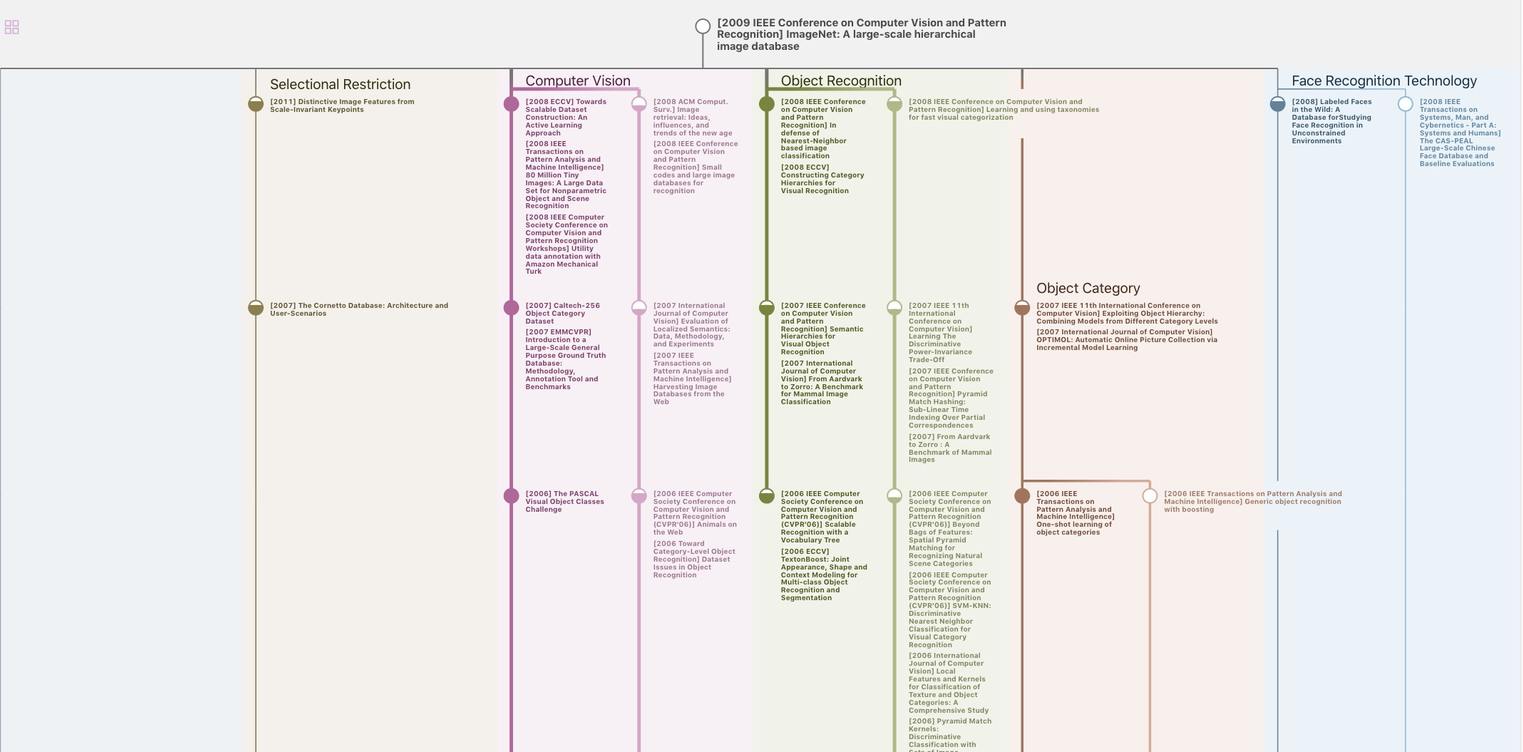
生成溯源树,研究论文发展脉络
Chat Paper
正在生成论文摘要