COMPASS: a formal framework and aggregate dataset for generalized surgical procedure modeling
arxiv(2023)
摘要
Purpose We propose a formal framework for the modeling and segmentation of minimally invasive surgical tasks using a unified set of motion primitives (MPs) to enable more objective labeling and the aggregation of different datasets. Methods We model dry-lab surgical tasks as finite state machines, representing how the execution of MPs as the basic surgical actions results in the change of surgical context, which characterizes the physical interactions among tools and objects in the surgical environment. We develop methods for labeling surgical context based on video data and for automatic translation of context to MP labels. We then use our framework to create the COntext and Motion Primitive Aggregate Surgical Set (COMPASS), including six dry-lab surgical tasks from three publicly available datasets (JIGSAWS, DESK, and ROSMA), with kinematic and video data and context and MP labels. Results Our context labeling method achieves near-perfect agreement between consensus labels from crowd-sourcing and expert surgeons. Segmentation of tasks to MPs results in the creation of the COMPASS dataset that nearly triples the amount of data for modeling and analysis and enables the generation of separate transcripts for the left and right tools. Conclusion The proposed framework results in high quality labeling of surgical data based on context and fine-grained MPs. Modeling surgical tasks with MPs enables the aggregation of different datasets and the separate analysis of left and right hands for bimanual coordination assessment. Our formal framework and aggregate dataset can support the development of explainable and multi-granularity models for improved surgical process analysis, skill assessment, error detection, and autonomy.
更多查看译文
关键词
Minimally invasive surgery,Robotic surgery,Surgical context,Surgical gesture recognition,Surgical process modeling
AI 理解论文
溯源树
样例
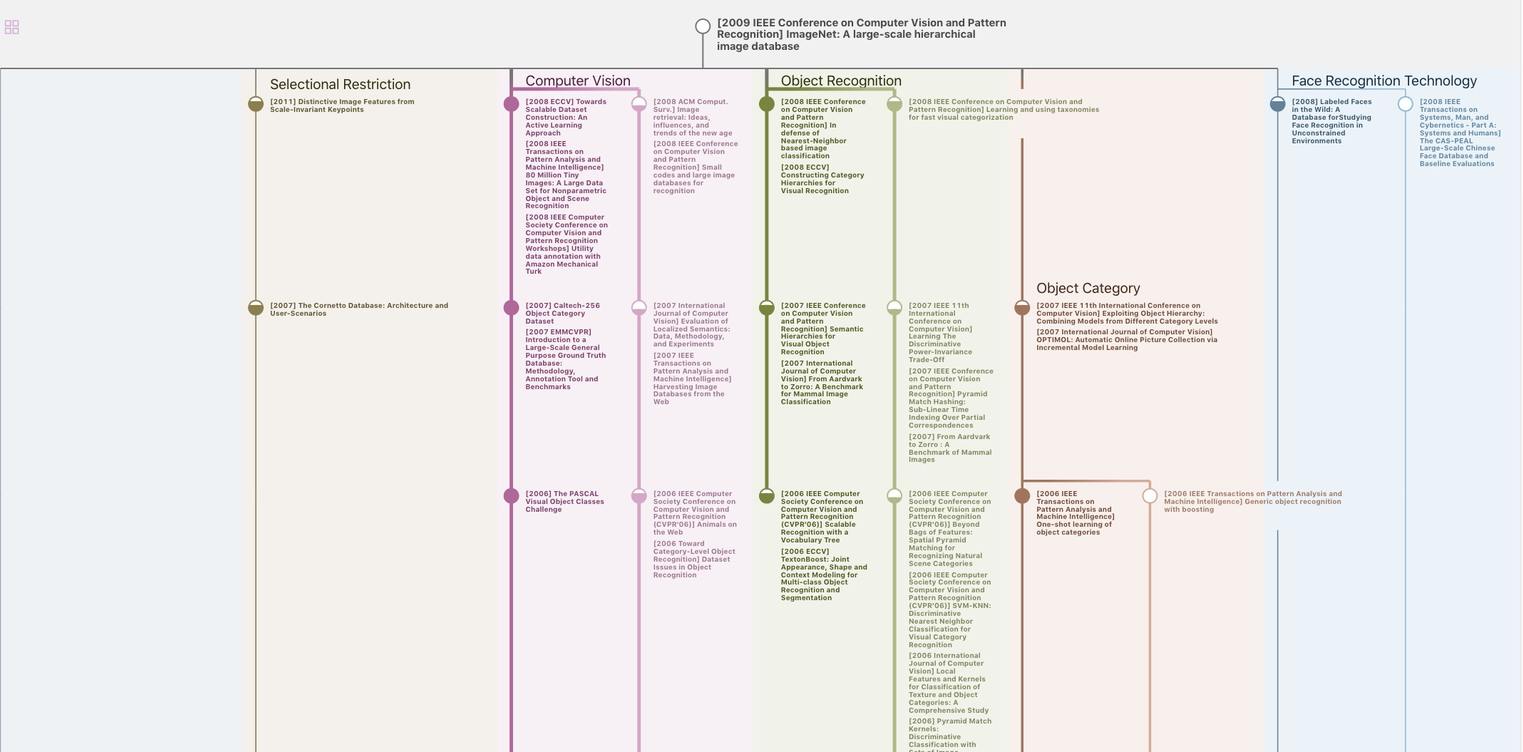
生成溯源树,研究论文发展脉络
Chat Paper
正在生成论文摘要