Joint User and Data Detection in Grant-Free NOMA With Attention-Based BiLSTM Network
IEEE OPEN JOURNAL OF THE COMMUNICATIONS SOCIETY(2023)
摘要
We consider the multi-user detection (MUD) problem in uplink grant-free non-orthogonal multiple access (NOMA), where the access point has to identify the total number and correct identity of the active Internet of Things (IoT) devices and decode their transmitted data. We assume that IoT devices use complex spreading sequences and transmit information in a random-access manner following the burst-sparsity model, where some IoT devices transmit their data in multiple adjacent time slots with a high probability, while others transmit only once during a frame. Exploiting the temporal correlation, we propose an attention-based bidirectional long short-term memory (BiLSTM) network to solve the MUD problem. The BiLSTM network creates a pattern of the device activation history using forward and reverse pass LSTMs, whereas the attention mechanism provides essential context to the device activation points. By doing so, a hierarchical pathway is followed for detecting active devices in a grant-free scenario. Then, by utilising the complex spreading sequences, blind data detection for the estimated active devices is performed. The proposed framework does not require prior knowledge of device sparsity levels and channels for performing MUD. The results show that the proposed network achieves better performance compared to existing benchmark schemes.
更多查看译文
关键词
Non-orthogonal multiple access,grant-free,deep learning,multi-user detection,Internet of Things
AI 理解论文
溯源树
样例
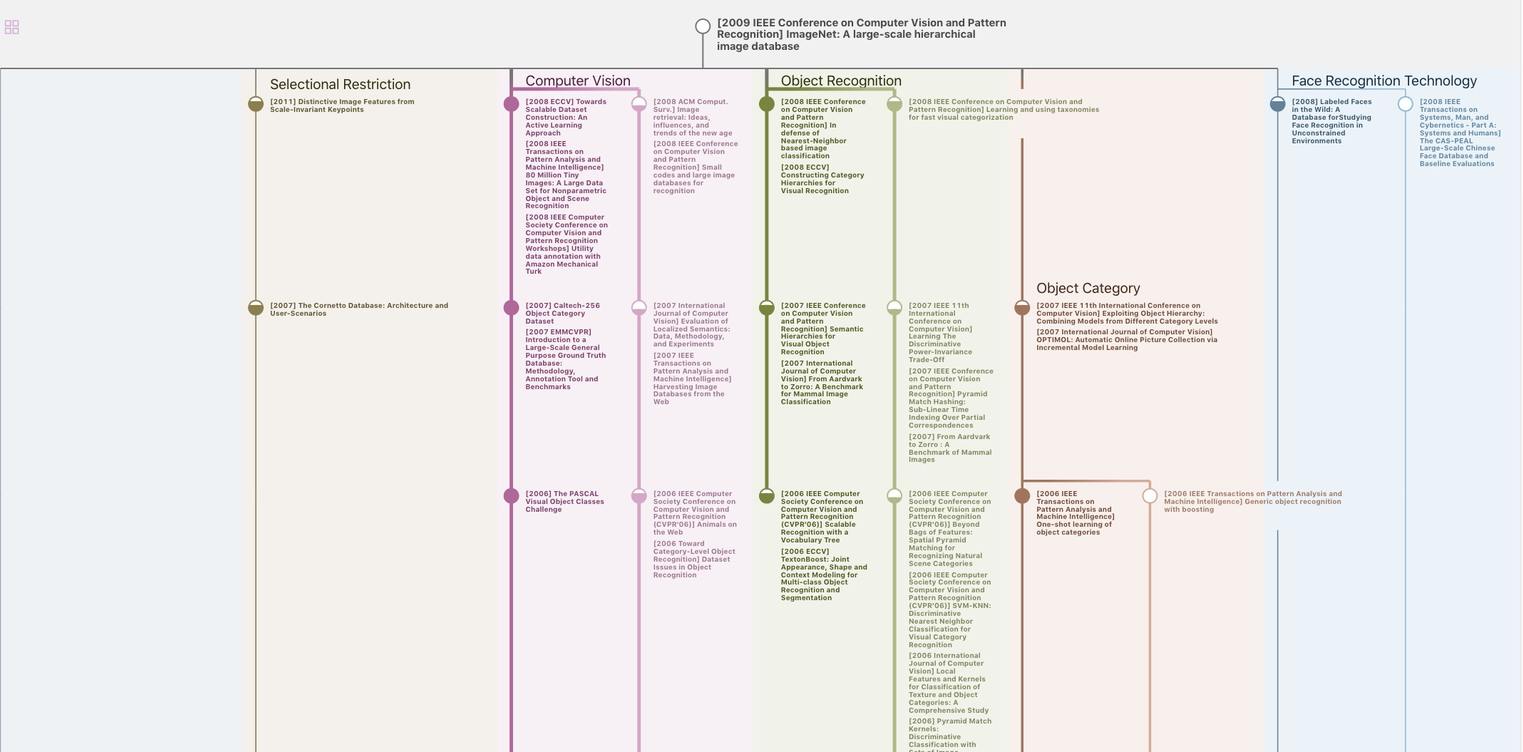
生成溯源树,研究论文发展脉络
Chat Paper
正在生成论文摘要