Heterogeneous attention based transformer for sign language translation
APPLIED SOFT COMPUTING(2023)
摘要
Sign language translation (SLT) has attracted significant interest both from research and industry, enabling convenient communications with the deaf-mute community. While recent transformer-based models have shown improved sign translation performance, it is still under-explored how to design an efficient transformer-based deep network architecture that effectively extracts joint visual-text features by exploiting multi-level spatial and temporal contextual information. In this paper, we propose heterogeneous attention based transformer(HAT), a novel SLT model to generate attentions from diverse spatial and temporal contextual levels. Specifically, the proposed light dual-stream sparse attention-based module yields more effective visual-text representations compared to conventional transformers. Extensive experiments demonstrate that our HAT achieves state-of-the-art performance on the challenging PHOENIX2014T benchmark dataset with a BLEU-4 score of 25.33 on the test set.(c) 2023 The Author(s). Published by Elsevier B.V. This is an open access article under the CC BY license (http://creativecommons.org/licenses/by/4.0/).
更多查看译文
关键词
Sign language translation,Transformer,Attention-based models,Dual-sparse attention,PHOENIX2014T
AI 理解论文
溯源树
样例
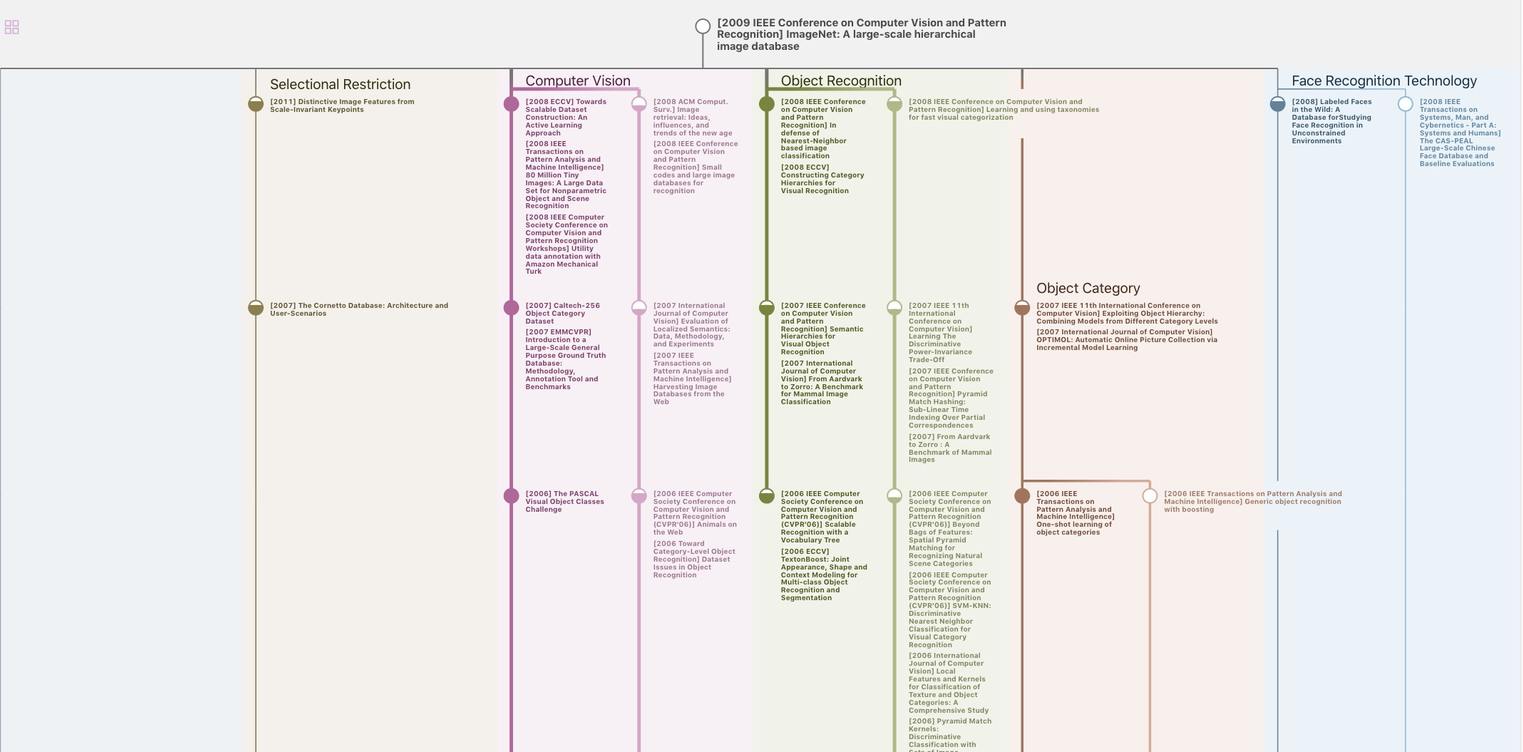
生成溯源树,研究论文发展脉络
Chat Paper
正在生成论文摘要