Sample Complexity Bounds for Learning High-dimensional Simplices in Noisy Regimes
arxiv(2022)
摘要
In this paper, we propose a sample complexity bound for learning a simplex from noisy samples. A dataset of size $n$ is given which includes i.i.d. samples drawn from a uniform distribution over an unknown arbitrary simplex in $\mathbb{R}^K$, where samples are assumed to be corrupted by an additive Gaussian noise of an arbitrary magnitude. We propose a strategy which outputs a simplex having, with high probability, a total variation distance of $\epsilon + O\left(\mathrm{SNR}^{-1}\right)$ from the true simplex, for any $\epsilon>0$. We prove that to arrive this close to the true simplex, it is sufficient to have $n\ge\tilde{O}\left(K^2/\epsilon^2\right)$ samples. Here, SNR stands for the signal-to-noise ratio which can be viewed as the ratio of the diameter of the simplex to the standard deviation of the noise. Our proofs are based on recent advancements in sample compression techniques, which have already shown promises in deriving tight bounds for density estimation in high-dimensional Gaussian mixture models.
更多查看译文
AI 理解论文
溯源树
样例
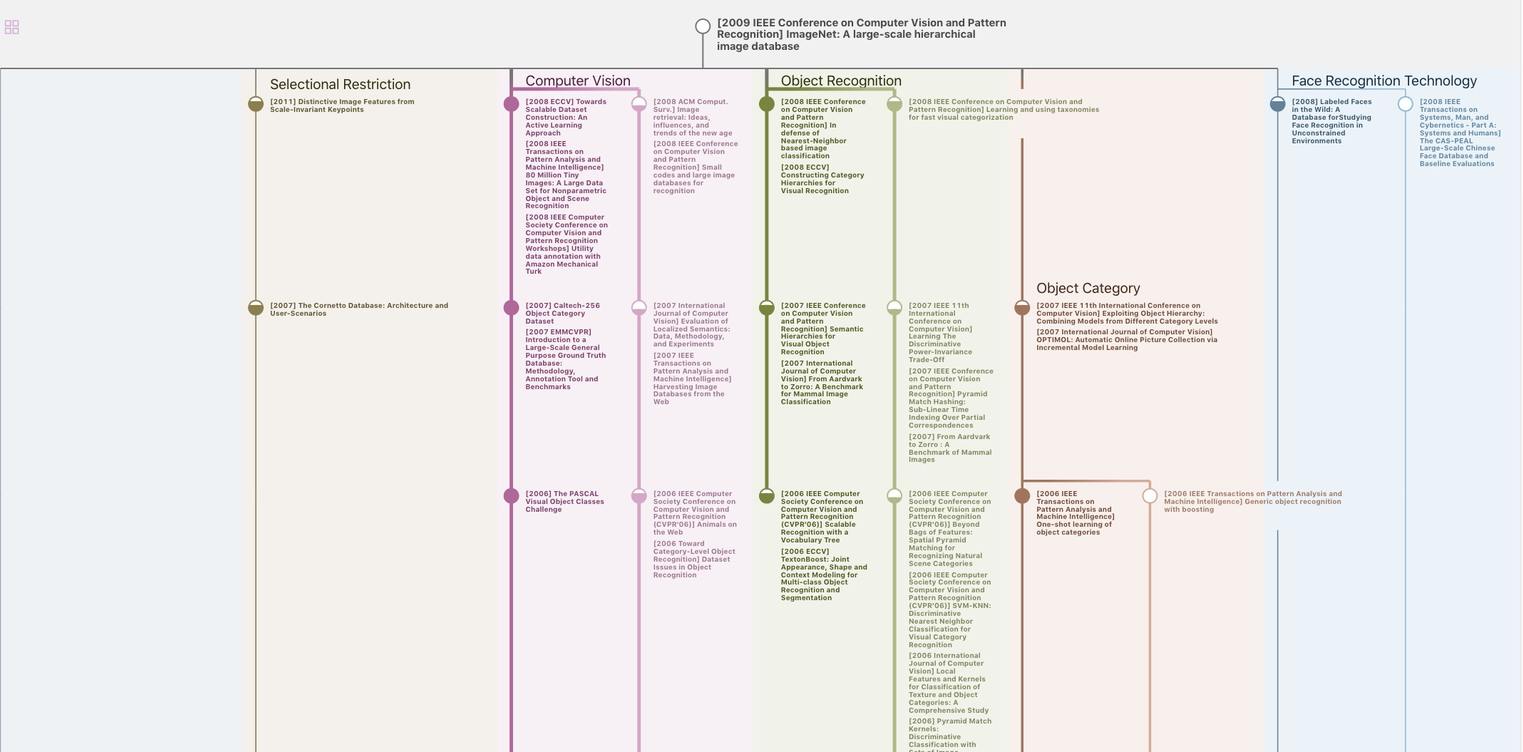
生成溯源树,研究论文发展脉络
Chat Paper
正在生成论文摘要