Federated Meta-Learning for Traffic Steering in O-RAN
2022 IEEE 96th Vehicular Technology Conference (VTC2022-Fall)(2022)
摘要
The vision of 5G lies in providing high data rates, low latency (for the aim of near-real-time applications), significantly increased base station capacity, and near-perfect quality of service (QoS) for users, compared to LTE networks. In order to provide such services, 5G systems will support various combinations of access technologies such as LTE, NR, NR-U and Wi-Fi. Each radio access technology (RAT) provides different types of access, and these should be allocated and managed optimally among the users. Besides resource management, 5G systems will also support a dual connectivity service. The orchestration of the network therefore becomes a more difficult problem for system managers with respect to legacy access technologies. In this paper, we propose an algorithm for RAT allocation based on federated meta-learning (FML), which enables RAN intelligent controllers (RICs) to adapt more quickly to dynamically changing environments. We have designed a simulation environment which contains LTE and 5G NR service technologies. In the simulation, our objective is to fulfil UE demands within the deadline of transmission to provide higher QoS values. We compared our proposed algorithm with a single RL agent, the Reptile algorithm and a rule-based heuristic method. Simulation results show that the proposed FML method achieves higher caching rates at first deployment round 21% and 12% respectively. Moreover, proposed approach adapts to new tasks and environments most quickly amongst the compared methods.
更多查看译文
关键词
Federated Meta Learning,Reinforcement learning,Traffic steering,5G,O-RAN,Resource management
AI 理解论文
溯源树
样例
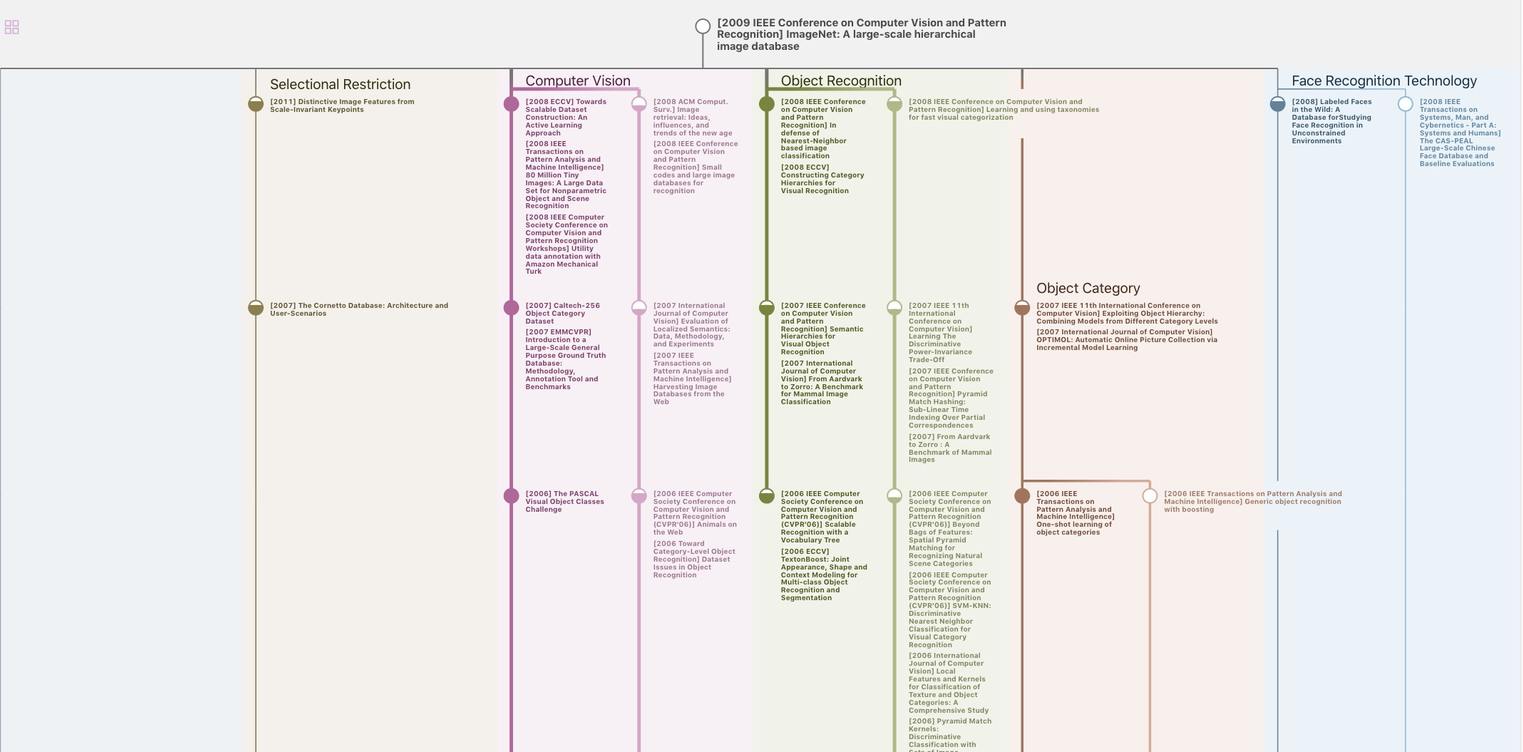
生成溯源树,研究论文发展脉络
Chat Paper
正在生成论文摘要