Model-based Reinforcement Learning with Multi-step Plan Value Estimation
arxiv(2022)
摘要
A promising way to improve the sample efficiency of reinforcement learning is model-based methods, in which many explorations and evaluations can happen in the learned models to save real-world samples. However, when the learned model has a non-negligible model error, sequential steps in the model are hard to be accurately evaluated, limiting the model's utilization. This paper proposes to alleviate this issue by introducing multi-step plans to replace multi-step actions for model-based RL. We employ the multi-step plan value estimation, which evaluates the expected discounted return after executing a sequence of action plans at a given state, and updates the policy by directly computing the multi-step policy gradient via plan value estimation. The new model-based reinforcement learning algorithm MPPVE (Model-based Planning Policy Learning with Multi-step Plan Value Estimation) shows a better utilization of the learned model and achieves a better sample efficiency than state-of-the-art model-based RL approaches.
更多查看译文
关键词
reinforcement,plan,learning,model-based,multi-step
AI 理解论文
溯源树
样例
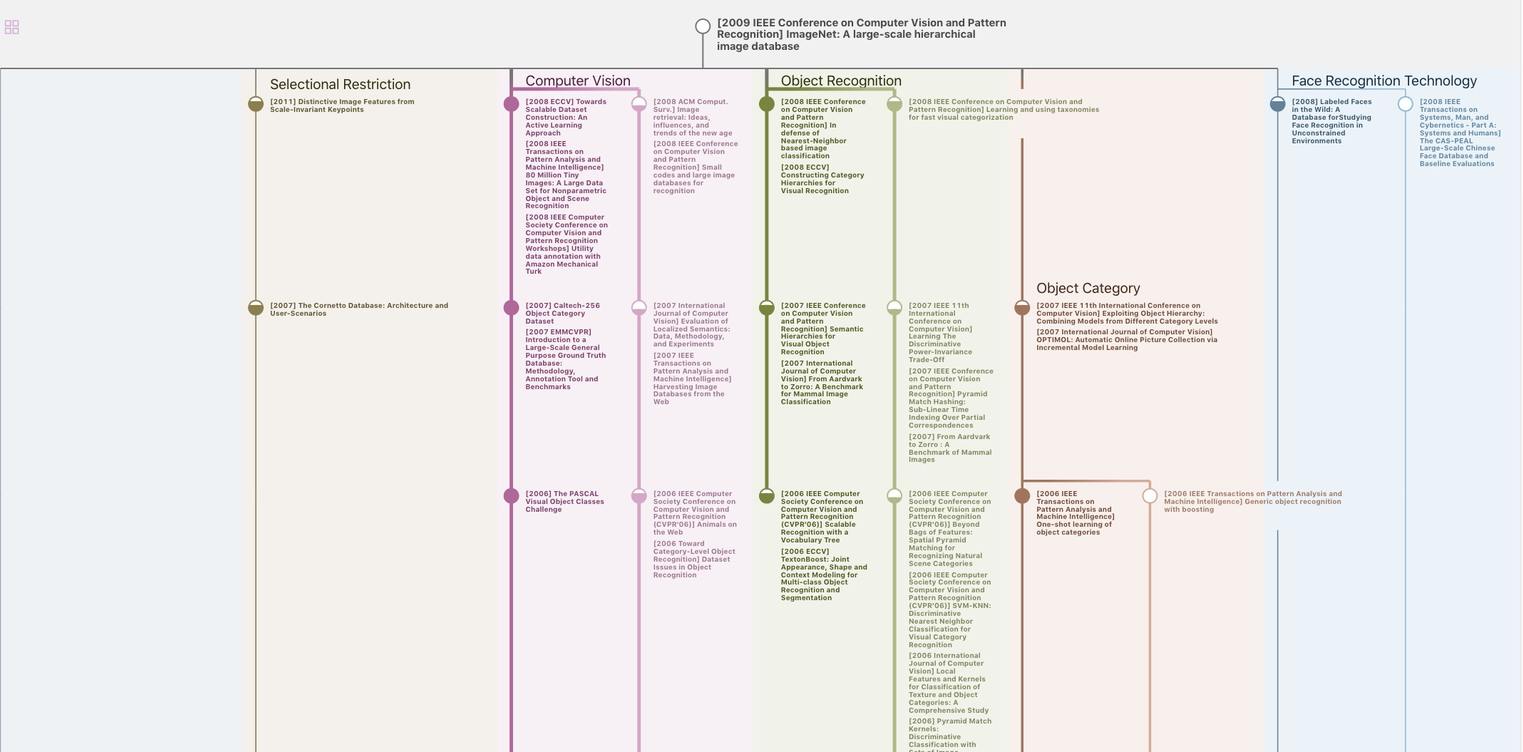
生成溯源树,研究论文发展脉络
Chat Paper
正在生成论文摘要