TEDL: A Two-stage Evidential Deep Learning Method for Classification Uncertainty Quantification
arxiv(2022)
摘要
In this paper, we propose TEDL, a two-stage learning approach to quantify uncertainty for deep learning models in classification tasks, inspired by our findings in experimenting with Evidential Deep Learning (EDL) method, a recently proposed uncertainty quantification approach based on the Dempster-Shafer theory. More specifically, we observe that EDL tends to yield inferior AUC compared with models learnt by cross-entropy loss and is highly sensitive in training. Such sensitivity is likely to cause unreliable uncertainty estimation, making it risky for practical applications. To mitigate both limitations, we propose a simple yet effective two-stage learning approach based on our analysis on the likely reasons causing such sensitivity, with the first stage learning from cross-entropy loss, followed by a second stage learning from EDL loss. We also re-formulate the EDL loss by replacing ReLU with ELU to avoid the Dying ReLU issue. Extensive experiments are carried out on varied sized training corpus collected from a large-scale commercial search engine, demonstrating that the proposed two-stage learning framework can increase AUC significantly and greatly improve training robustness.
更多查看译文
关键词
deep learning,classification
AI 理解论文
溯源树
样例
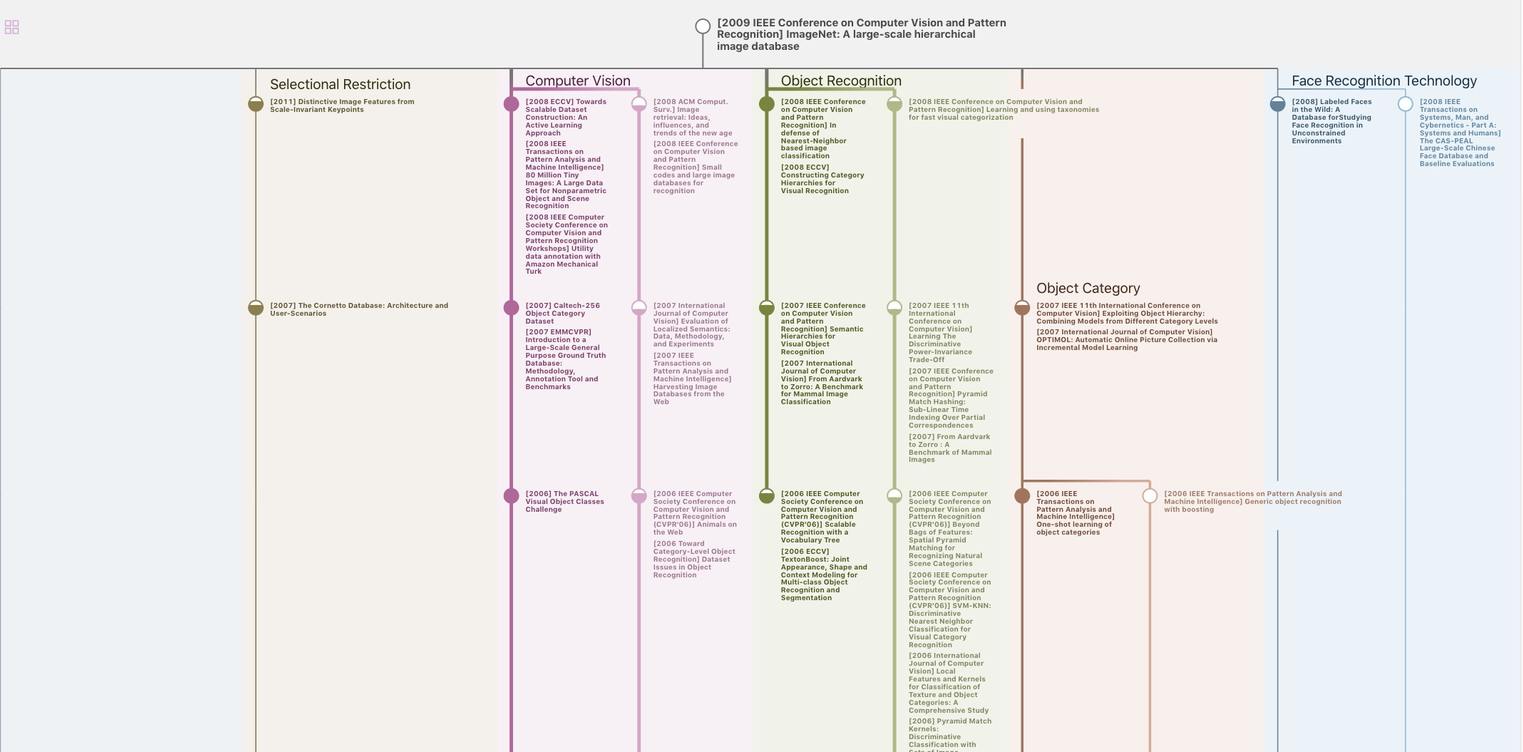
生成溯源树,研究论文发展脉络
Chat Paper
正在生成论文摘要