Early detection of myocardial ischemia in 12-lead ECG using deterministic learning and ensemble learning
COMPUTER METHODS AND PROGRAMS IN BIOMEDICINE(2022)
摘要
Background and objective: Early detection of myocardial ischemia is a necessary but difficult problem in cardiovascular diseases. Approaches that exclusively rely on classical ST and T wave changes on the standard 12-lead electrocardiogram (ECG) lack sufficient accuracy in detecting myocardial ischemia. This study aims to construct generalizable models for the detection of myocardial ischemia in patients with subtle ECG waveform changes (namely non-diagnostic ECG) using ensemble learning to integrate ECG dy-namic features acquired via deterministic learning. Methods: First, cardiodynamicsgram (CDG), a noninva-sive spatiotemporal electrocardiographic method, is generated through dynamic modeling of ECG signals using the deterministic learning algorithm. Then, the spectral fitting exponent, Lyapunov exponent, and Lempel-Ziv complexity are extracted from CDG. Subsequently, the bagging-based heterogeneous ensem-ble algorithm is applied on CDG features to generate diverse base classifiers and aggregate them with weighted voting to obtain an ensemble model for myocardial ischemia detection. Finally, we train and test the proposed heterogeneous ensemble model on a real-world clinical dataset. This dataset consists of 499 non-diagnostic 12-lead ECG records from 499 patients collected from three independent medi-cal centers, including 383 patients with myocardial ischemia and 116 patients without ischemia. Results: With 10-times 5-fold cross-validation technology, our proposed method achieves an average accuracy of 89.10%, sensitivity of 91.72%, and specificity of 82.69% using the heterogeneous ensemble algorithm on the real-world clinical dataset. On three independent medical centers, our ensemble model also achieves accuracy performance over 82% for patients with non-diagnostic ECG. Furthermore, our ensemble model trained with real-world clinical data yields promising results of 91.11% accuracy, 90.49% sensitivity, and 92.88% specificity on the external test set of the public PTB dataset. Conclusion: The experimental results demonstrate that the proposed model combining ensemble learning and deterministic learning presents excellent diagnostic accuracy and generalization in clinical practice, and could be implemented as a com-plement to the standard ECG in the clinical diagnosis of myocardial ischemia. (c) 2022 Published by Elsevier B.V.
更多查看译文
关键词
Electrocardiogram,Cardiodynamicsgram,Deterministic learning,Ensemble learning
AI 理解论文
溯源树
样例
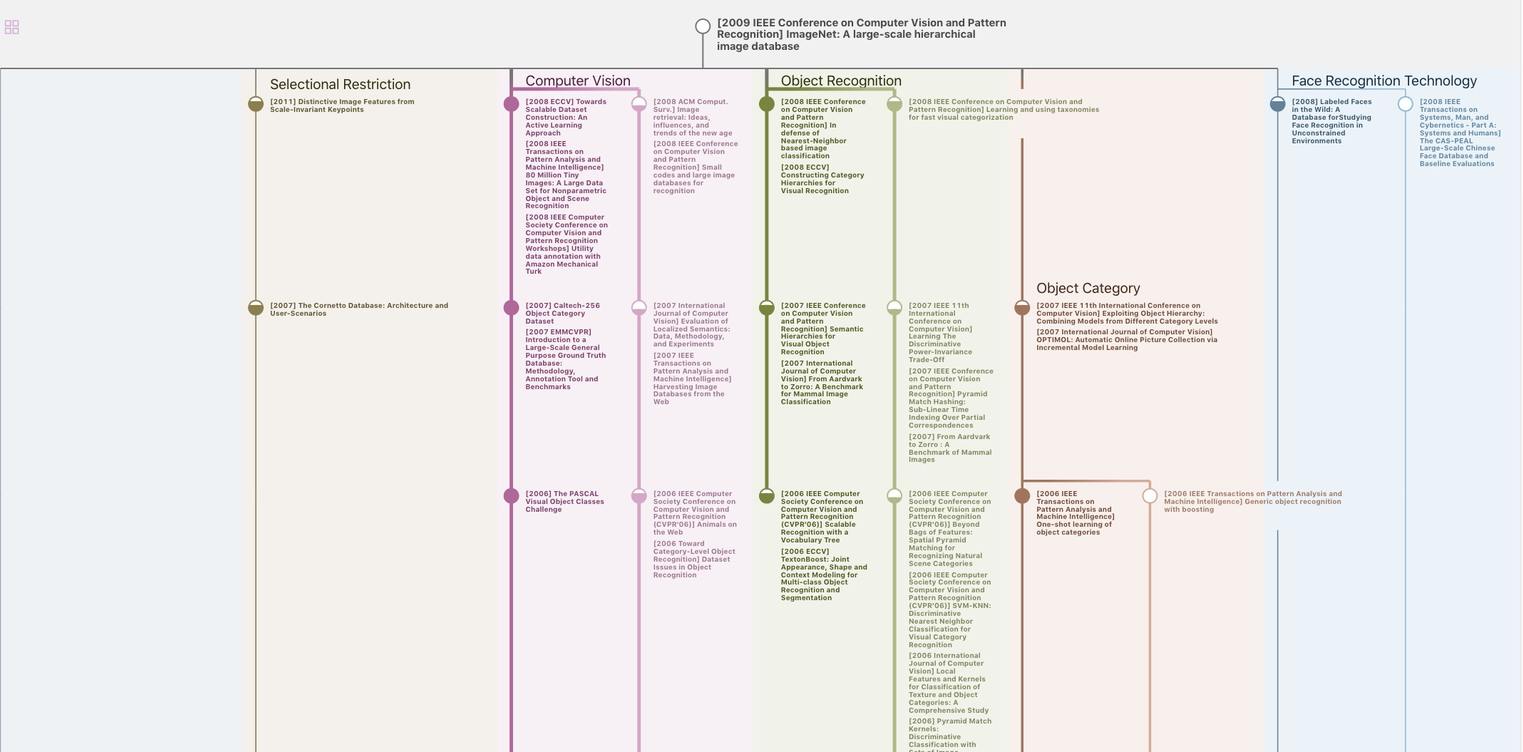
生成溯源树,研究论文发展脉络
Chat Paper
正在生成论文摘要