On the Factory Floor: ML Engineering for Industrial-Scale Ads Recommendation Models
Workshop on Online Recommender Systems and User Modeling(2022)
摘要
For industrial-scale advertising systems, prediction of ad click-through rate (CTR) is a central problem. Ad clicks constitute a significant class of user engagements and are often used as the primary signal for the usefulness of ads to users. Additionally, in cost-per-click advertising systems where advertisers are charged per click, click rate expectations feed directly into value estimation. Accordingly, CTR model development is a significant investment for most Internet advertising companies. Engineering for such problems requires many machine learning (ML) techniques suited to online learning that go well beyond traditional accuracy improvements, especially concerning efficiency, reproducibility, calibration, credit attribution. We present a case study of practical techniques deployed in Google's search ads CTR model. This paper provides an industry case study highlighting important areas of current ML research and illustrating how impactful new ML methods are evaluated and made useful in a large-scale industrial setting.
更多查看译文
AI 理解论文
溯源树
样例
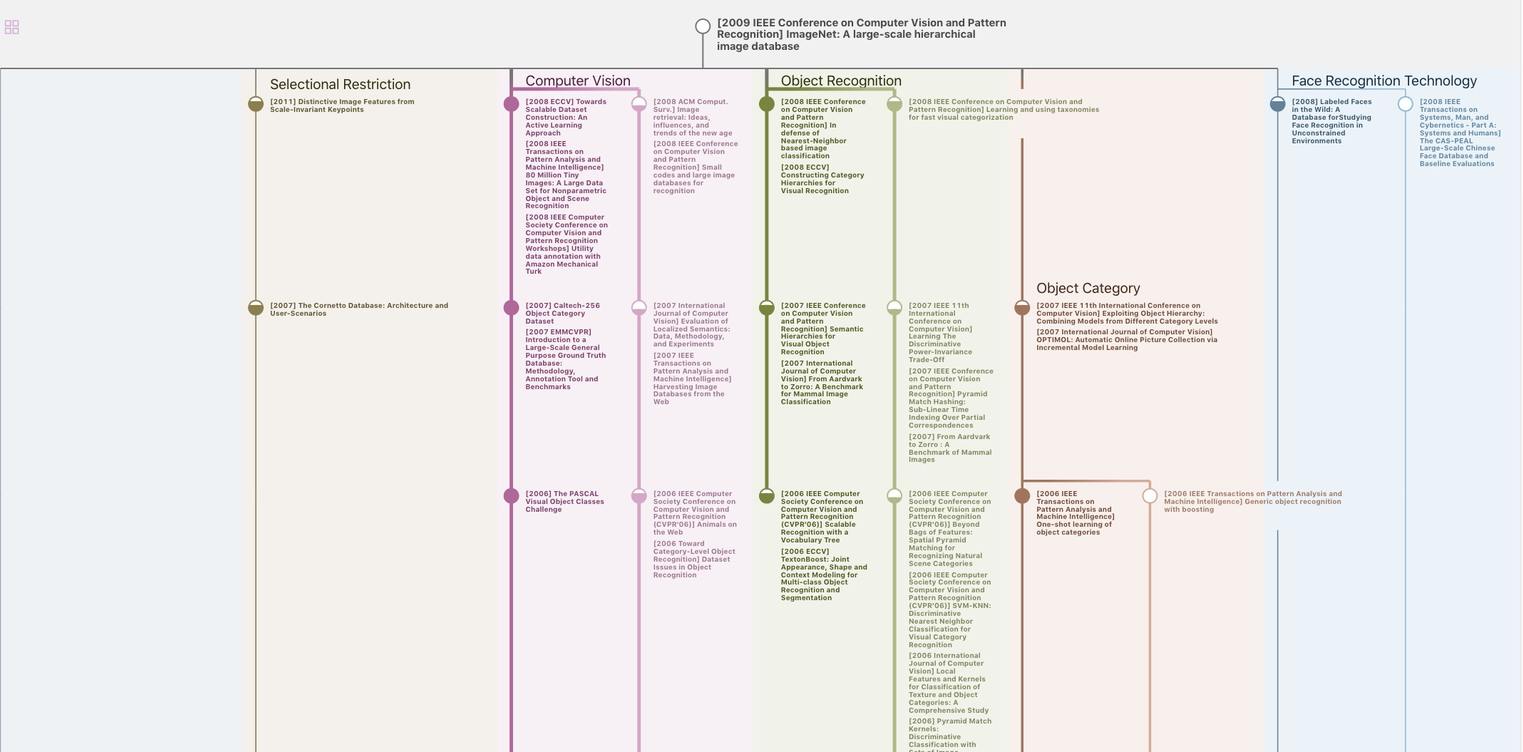
生成溯源树,研究论文发展脉络
Chat Paper
正在生成论文摘要