A new hazard event classification model via deep learning and multifractal
arxiv(2023)
摘要
Hazard and operability analysis (HAZOP) is the paradigm of industrial safety that can reveal the hazards of process from its node deviations, consequences, causes, measures and suggestions, and such hazards can be considered as hazard events (HaE). The classification research on HaE has much irreplaceable pragmatic values. In this paper, we present a novel deep learning model termed DLF through multifractal to explore HaE classification where the motivation is that HaE can be naturally regarded as a kind of time series. Specifically, first HaE is vectorized to get HaE time series by employing BERT. Then, a new multifractal analysis method termed HmF-DFA is proposed to win HaE fractal series by analyzing HaE time series. Finally, a new hierarchical gating neural network (HGNN) is designed to process HaE fractal series to accomplish the classification of HaE from three aspects: severity, possibility and risk. We take HAZOP reports of 18 processes as cases, and launch the experiments on this basis. Results demonstrate that compared with other classifiers, DLF classifier performs better under metrics of precision, recall and F1-score, especially for the severity aspect. Also, HmF-DFA and HGNN effectively promote HaE classification. Our HaE classification system can serve application incentives to experts, engineers, employees, and other enterprises. We hope our research can contribute added support to the daily practice in industrial safety.
更多查看译文
关键词
Hazard event classification,Multifractal analysis,Deep learning,Hierarchical gating neural network,Hazard and operability analysis
AI 理解论文
溯源树
样例
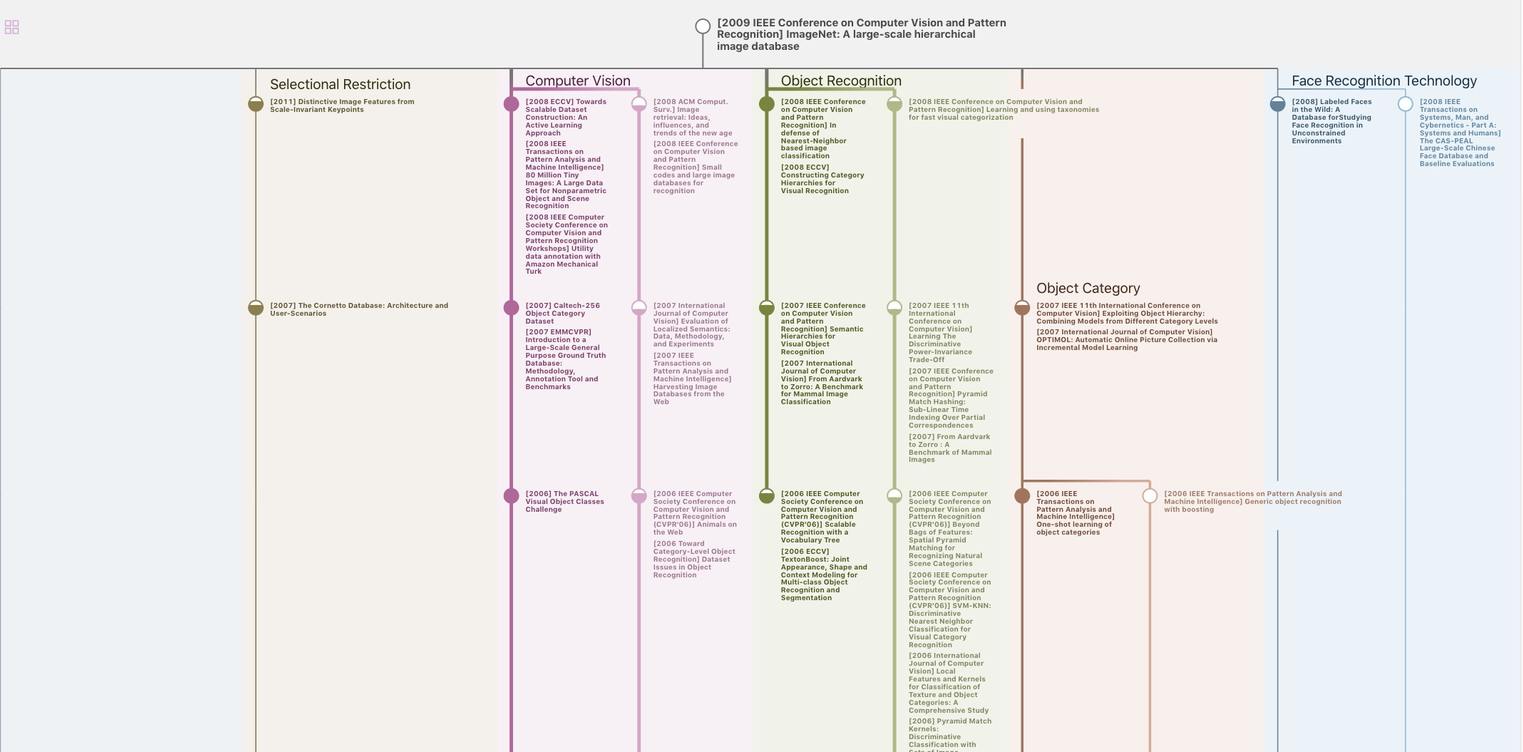
生成溯源树,研究论文发展脉络
Chat Paper
正在生成论文摘要