Partial Observability during DRL for Robot Control
arxiv(2022)
摘要
Deep Reinforcement Learning (DRL) has made tremendous advances in both simulated and real-world robot control tasks in recent years. Nevertheless, applying DRL to novel robot control tasks is still challenging, especially when researchers have to design the action and observation space and the reward function. In this paper, we investigate partial observability as a potential failure source of applying DRL to robot control tasks, which can occur when researchers are not confident whether the observation space fully represents the underlying state. We compare the performance of three common DRL algorithms, TD3, SAC and PPO under various partial observability conditions. We find that TD3 and SAC become easily stuck in local optima and underperform PPO. We propose multi-step versions of the vanilla TD3 and SAC to improve robustness to partial observability based on one-step bootstrapping.
更多查看译文
关键词
robot control,drl
AI 理解论文
溯源树
样例
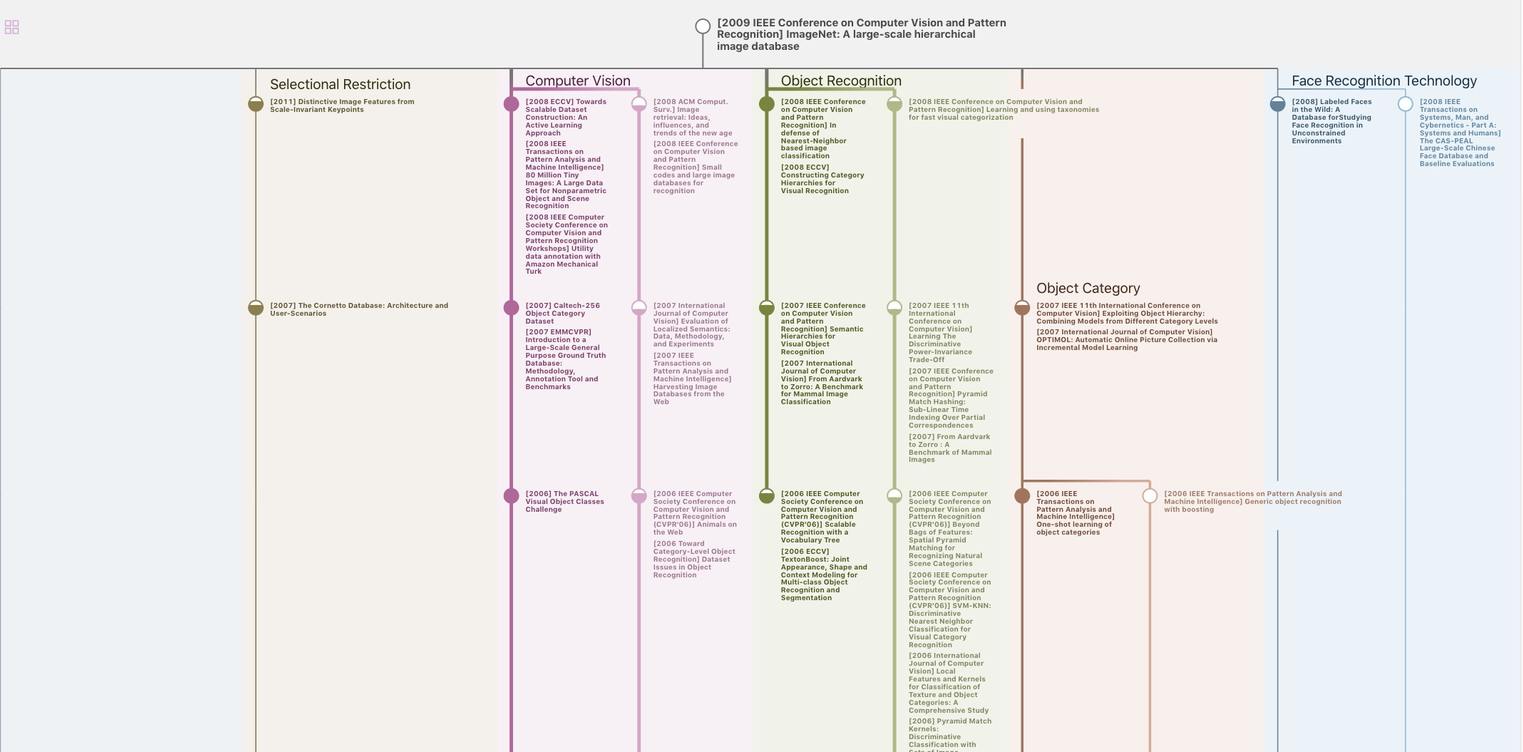
生成溯源树,研究论文发展脉络
Chat Paper
正在生成论文摘要