Generation of Turbulent States using Physics-Informed Neural Networks
arxiv(2022)
摘要
When modelling turbulent flows, it is often the case that information on the forcing terms or the boundary conditions is either not available or overly complicated and expensive to implement. Instead, some flow features, such as the mean velocity profile or its statistical moments, may be accessible through experiments or observations. We present a method based on physics-informed neural networks to generate turbulent states subject to a set of given conditions. The physics-informed method ensures the final state approximates a valid flow. We show examples of different statistical conditions that can be used to prepare states, motivated by experimental and atmospheric problems. Lastly, we show two ways of scaling the resolution of the prepared states. One is through the use of multiple and parallel neural networks. The other uses nudging, a synchronization-based data assimilation technique that leverages the power of specialized numerical solvers.
更多查看译文
关键词
turbulent states,neural networks,physics-informed
AI 理解论文
溯源树
样例
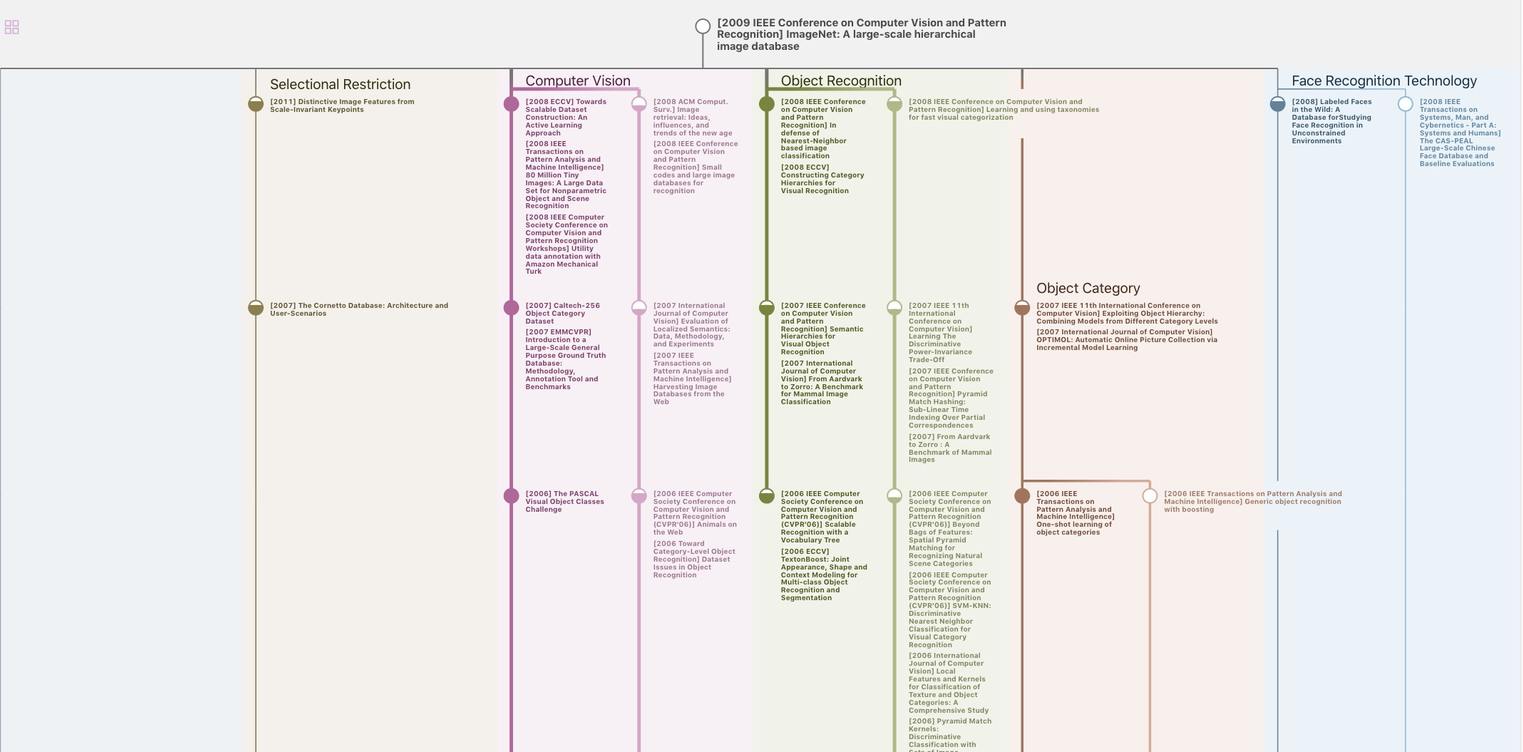
生成溯源树,研究论文发展脉络
Chat Paper
正在生成论文摘要