Boosting Lying Posture Classification with Transfer Learning
2022 44th Annual International Conference of the IEEE Engineering in Medicine & Biology Society (EMBC)(2022)
摘要
Automatic lying posture tracking is an important factor in human health monitoring. The increasing popularity of the wrist-based trackers provides the means for unobtrusive, affordable, and long-term monitoring with minimized privacy concerns for the end-users and promising results in detecting the type of physical activity, step counting, and sleep quality assessment. However, there is limited research on development of accurate and efficient lying posture tracking models using wrist-based sensor. Our experiments demonstrate a major drop in the accuracy of the lying posture tracking using wrist-based accelerometer sensor due to the unpredictable noise from arbitrary wrist movements and rotations while sleeping. In this paper, we develop a deep transfer learning method that improves performance of lying posture tracking using noisy data from wrist sensor by transferring the knowledge from an initial setting which contains both clean and noisy data. The proposed solution develops an optimal mapping model from the noisy data to the clean data in the initial setting using LSTM sequence regression, and reconstruct clean synthesized data in another setting where no noisy sensor data is available. This increases the lying posture tracking F1-Score by 24.9% for ‘left-wrist’ and by 18.1% for ‘right-wrist’ sensors comparing to the case without mapping.
更多查看译文
关键词
Humans,Learning,Machine Learning,Movement,Posture,Wrist
AI 理解论文
溯源树
样例
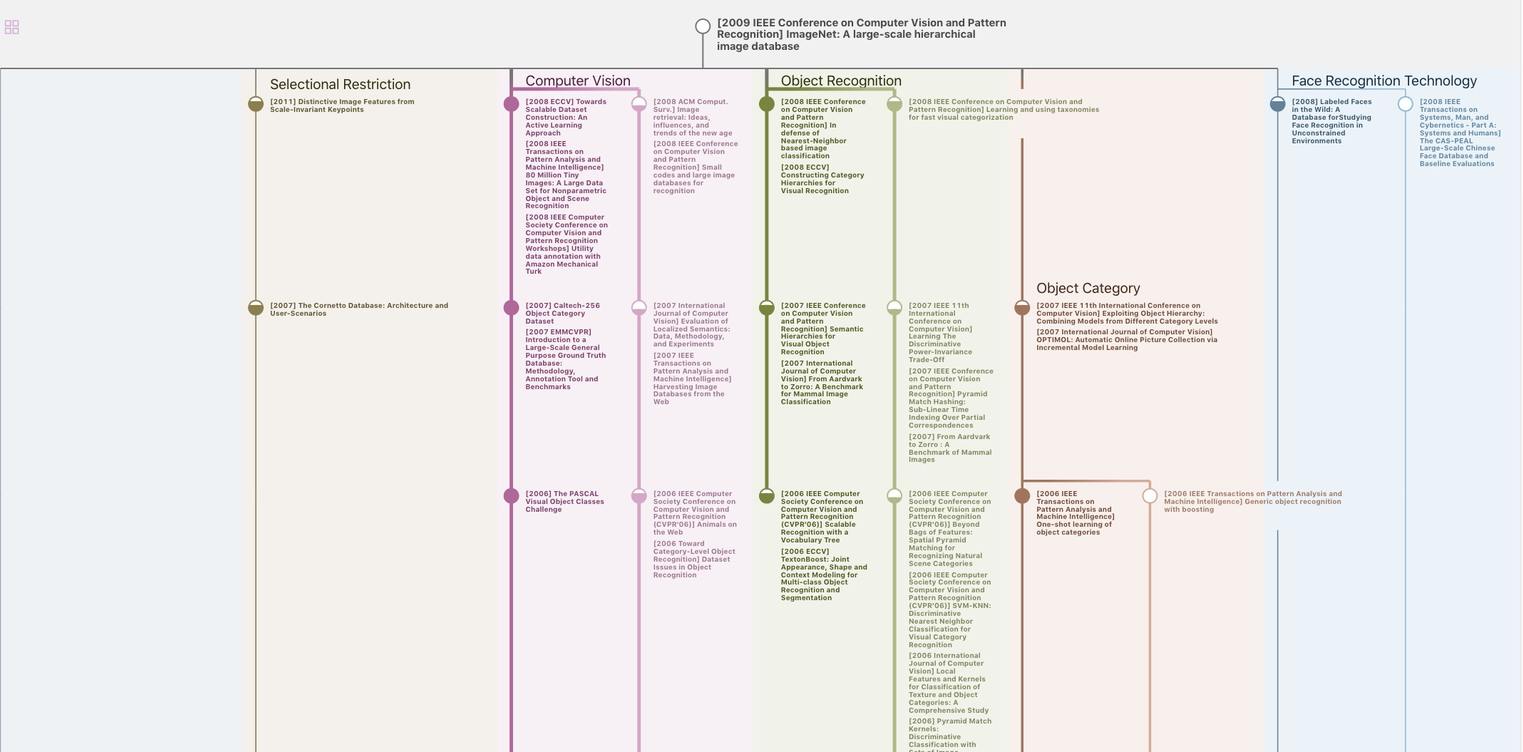
生成溯源树,研究论文发展脉络
Chat Paper
正在生成论文摘要