Methods Used to Estimate EEG Source-Space Networks: A Comparative Simulation-Based Study.
Annual International Conference of the IEEE Engineering in Medicine and Biology Society (EMBC)(2022)
摘要
Along with the study of the brain activity evoked by external stimuli, an important advance in current neuroscience involves understanding the spontaneous brain activity that occurs during resting conditions. Interestingly, the identification of the connectivity patterns in "resting-state" has been the subject of a great number of electrophysiology-based studies. In this context, the Electroencephalography (EEG) source connectivity method enables estimating resting-state cortical networks from scalp-EEG recordings. However, there is still no consensus over a unified pipeline adapted in all cases (e.g., type of task, a priori on studied networks) and numerous methodological questions remain unanswered. In order to address this problem, we simulated, using neural mass models, EEG data corresponding to the default mode network (DMN), the most widely studied resting-state network, and tested the effect of different channel densities, two inverse solutions and two functional connectivity measures on the correspondence between the reconstructed networks and the reference networks. Results showed that increasing the number of electrodes enhances the accuracy of the network reconstruction, and that eLORETA/PLV led to better accuracy than other inverse solution/connectivity measure combinations in terms of the correlation between reconstructed and reference connectivity matrices. This work has a wide range of implications in the field of electrophysiology connectomics, and is a step towards a convergence and standardization of approaches in this emerging field.
更多查看译文
关键词
Brain,Computer Simulation,Connectome,Electroencephalography,Rest
AI 理解论文
溯源树
样例
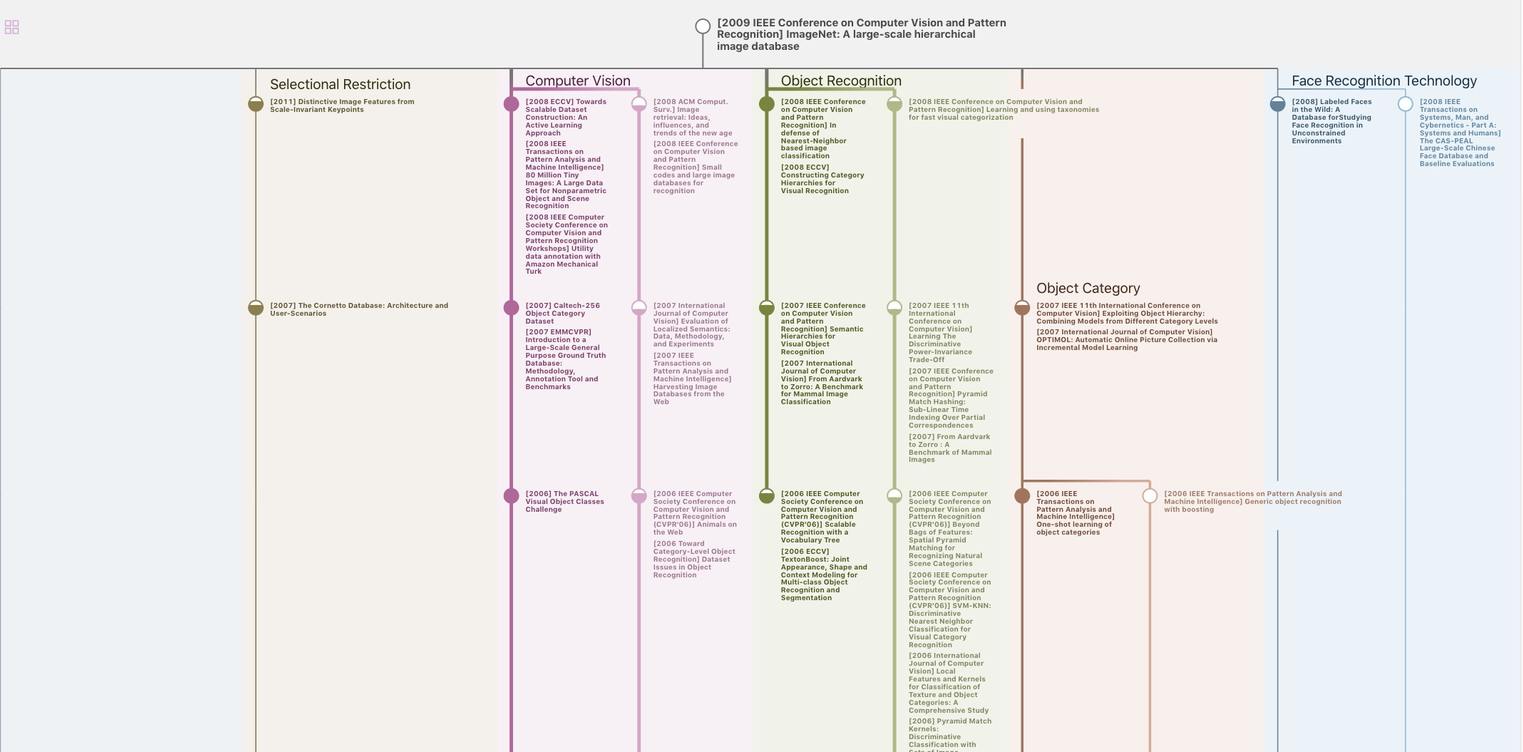
生成溯源树,研究论文发展脉络
Chat Paper
正在生成论文摘要