A "smart" Imputation Approach for Effective Quality Control Across Complex Clinical Data Structures.
Annual International Conference of the IEEE Engineering in Medicine and Biology Society (EMBC)(2022)
摘要
The overwhelming need to improve the quality of complex data structures in healthcare is more important than ever. Although data quality has been the point of interest in many studies, none of them has focused on the development of quantitative and explainable methods for data imputation. In this work, we propose a "smart" imputation workflow to address missing data across complex data structures in the context of in silico clinical trials. AI algorithms were utilized to produce high-quality virtual patient profiles. A search algorithm was then developed to extract the best virtual patient profiles through the definition of a profile matching score (PMS). A case study was conducted, where the real dataset was randomly contaminated with multiple missing values (e.g., 10 to 50%). In total, 10000 virtual patient profiles with less than 0.02 Kullback-Leibler (KL) divergence were produced to estimate the PMS distribution. The best generator achieved the lowest average squared absolute difference (0.4) and average correlation difference (0.02) with the real dataset highlighting its increased effectiveness for data imputation across complex clinical data structures.
更多查看译文
关键词
Algorithms,Humans,Quality Control
AI 理解论文
溯源树
样例
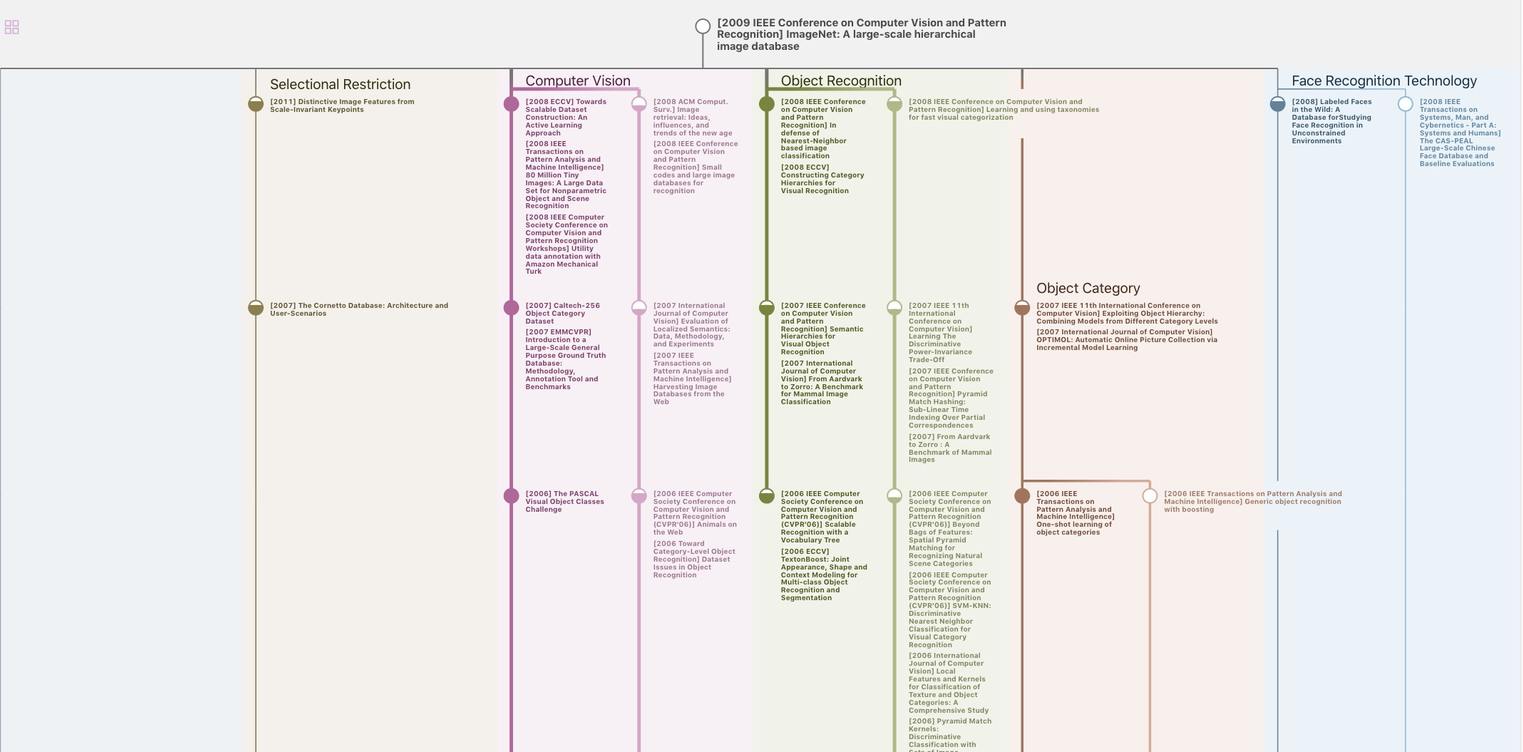
生成溯源树,研究论文发展脉络
Chat Paper
正在生成论文摘要