A Comparative Study between Image- and Projection-Domain Self-Supervised Learning for Ultra Low-Dose CBCT.
Annual International Conference of the IEEE Engineering in Medicine and Biology Society (EMBC)(2022)
摘要
We compare image domain and projection domain denoising approaches with self-supervised learning for ultra low-dose cone-beam CT (CBCT), where number of detected x-ray photons is significantly low. For image-domain self-supervised denoising, we first reconstruct CBCT images with the standard filtered backprojection. For model training, we use blind-spot filtering to partially blind images and recover the blind spots. For projection-domain self-supervised denoising, we regard the post-log projections as training examples of convolutional neural network. From experimental results with various low-dose CBCT settings, the projection-domain denoiser outperforms the image-domain denoiser both in image quality and accuracy for ultra low-dose CBCT.
更多查看译文
关键词
learning,projection-domain,self-supervised,low-dose
AI 理解论文
溯源树
样例
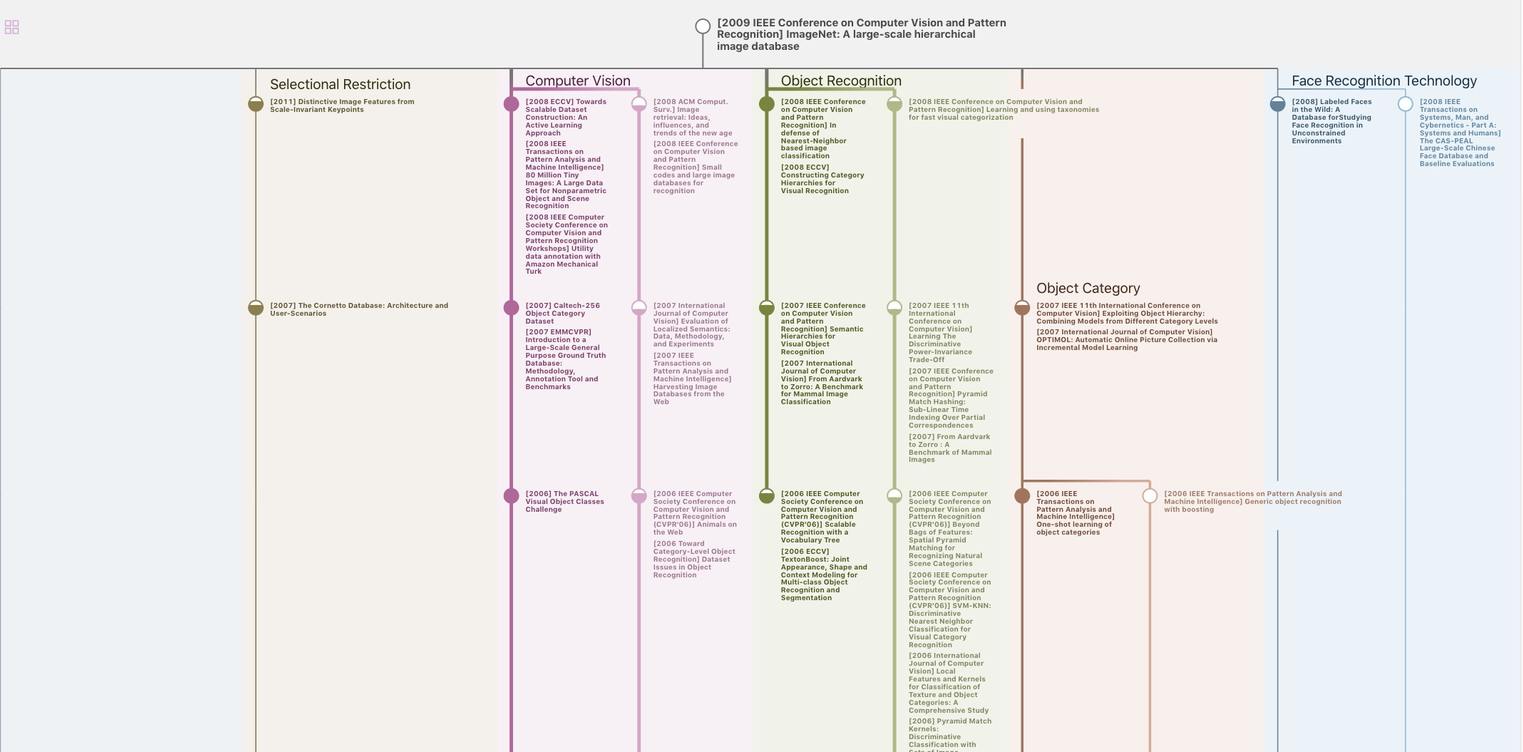
生成溯源树,研究论文发展脉络
Chat Paper
正在生成论文摘要