Interpretable Dimension Reduction for MRI Channel Suppression.
Annual International Conference of the IEEE Engineering in Medicine and Biology Society (EMBC)(2022)
摘要
Channel suppression can reduce the redundant information in multiple channel receiver coils and accelerate reconstruction speed to meet real-time imaging requirements. The principal component analysis has been used for channel suppression, but it is difficult to be interpreted because all channels contribute to principal components. Furthermore, the importance of interpretability in machine learning has recently attracted increasing attention in radiology. To improve the interpretability of PCA-based channel suppression, a sparse PCA method is proposed to reduce the most coils' loadings to be zero. Channel suppression is formulated as solving a nonlinear eigenvalue problem using the inverse power method instead of the direct matrix decomposition. Experimental results of in vivo data show that the sparse PCA-based channel suppression not only improves the interpretability with sparse channels, but also improves reconstruction quality compared to the standard PCA-based reconstruction with the similar reconstruction time.
更多查看译文
关键词
Algorithms,Magnetic Resonance Imaging,Principal Component Analysis,Reconstructive Surgical Procedures,Records
AI 理解论文
溯源树
样例
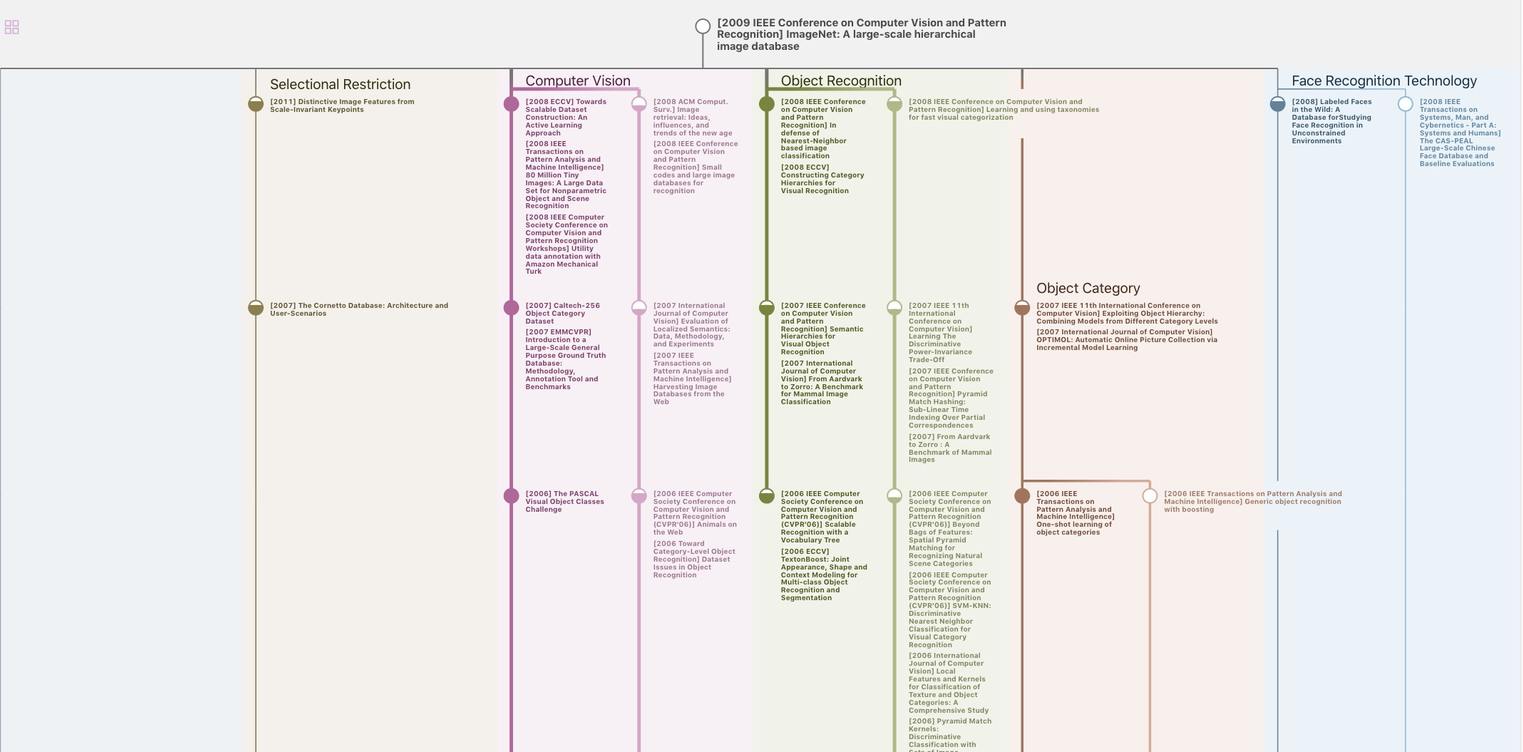
生成溯源树,研究论文发展脉络
Chat Paper
正在生成论文摘要