CNN-Based Classification of Craniosynostosis Using 2D Distance Maps.
Annual International Conference of the IEEE Engineering in Medicine and Biology Society (EMBC)(2022)
摘要
Craniosynostosis is a condition associated with the premature fusion of skull sutures affecting infants. 3D photogrammetric scans are a promising alternative to computed tomography scans in cases of single suture or nonsyndromic synostosis for diagnostic imaging, but oftentimes diagnosis is not automated and relies on additional cephalometric measure-ments and the experience of the surgeon. We propose an alternative representation of the infant's head shape created from 3D photogrammetric surface scans as 2D distance maps. Those 2D distance maps rely on ray casting to extract distances from a center point to the head surface, arranging them into a 2D image grid. We use the distance map for an original convolutional neural network (CNN)-based classification approach, which is evaluated on a publicly available synthetic dataset for benchmarking and also tested on clinical data. Qualitative differences of different head shapes can be ob-served in the distance maps. The CNN-based classifier achieves accuracies of 100 % on the publicly available synthetic dataset and 98.86 % on the clinical test set. Our distance map approach demonstrates the diagnostic value of 3D photogrammetry and the possibility of automatic, CNN-based diagnosis. Future steps include the improvement of the mapping method and testing the CNN on more pathologies.
更多查看译文
关键词
Bone and Bones,Craniosynostoses,Humans,Infant,Neural Networks, Computer,Tomography, X-Ray Computed
AI 理解论文
溯源树
样例
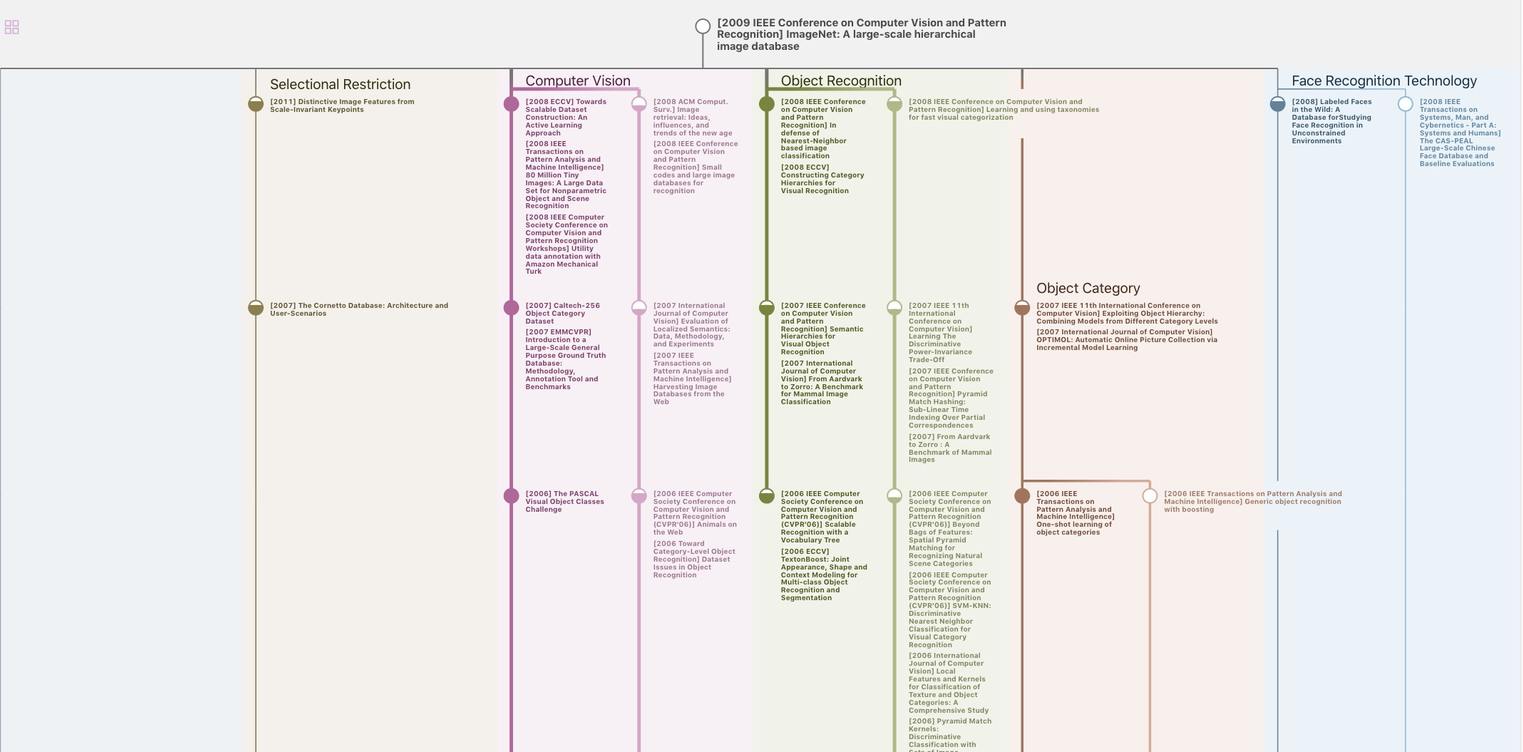
生成溯源树,研究论文发展脉络
Chat Paper
正在生成论文摘要