Classification-guided Neural Network-based Correction of Magnetic Resonance-related Gradient Artifact Residuals in Simultaneously Recorded Surface Electromyography.
Annual International Conference of the IEEE Engineering in Medicine and Biology Society (EMBC)(2022)
摘要
Spontaneous muscular activities can be studied by simultaneous recordings of surface electromyography (sEMG) and diffusion-weighted magnetic resonance imaging (DW-MRI). For reliable assessment of the spontaneous activity rate in sEMG data during active MR imaging, it is necessary to have a decent gradient artifact (GA) correction algorithm enabling the detection of small spontaneous activities with an amplitude of few microvolts. In this work, a neural network with weak label annotations during the training process is utilized for enhanced correction of GA residuals in the sEMG recordings. Based on sEMG signal decomposition and class-activation maps from the neural network classification, the amount of GA residuals is iteratively decreased in the sEMG signal. This leads to a reduction of the false-positive rate in automated spontaneous activity detection. Quality of GA residual correction is therefore estimated by using a specialized second neural network model. Clinical relevance- This work establishes an improved GA residual correction for simultaneously recorded sEMG data during MRI to enhance the ability for small spontaneous activity detection.
更多查看译文
关键词
Artifacts,Diffusion Magnetic Resonance Imaging,Electromyography,Magnetic Resonance Imaging,Magnetic Resonance Spectroscopy,Neural Networks, Computer,Signal Processing, Computer-Assisted
AI 理解论文
溯源树
样例
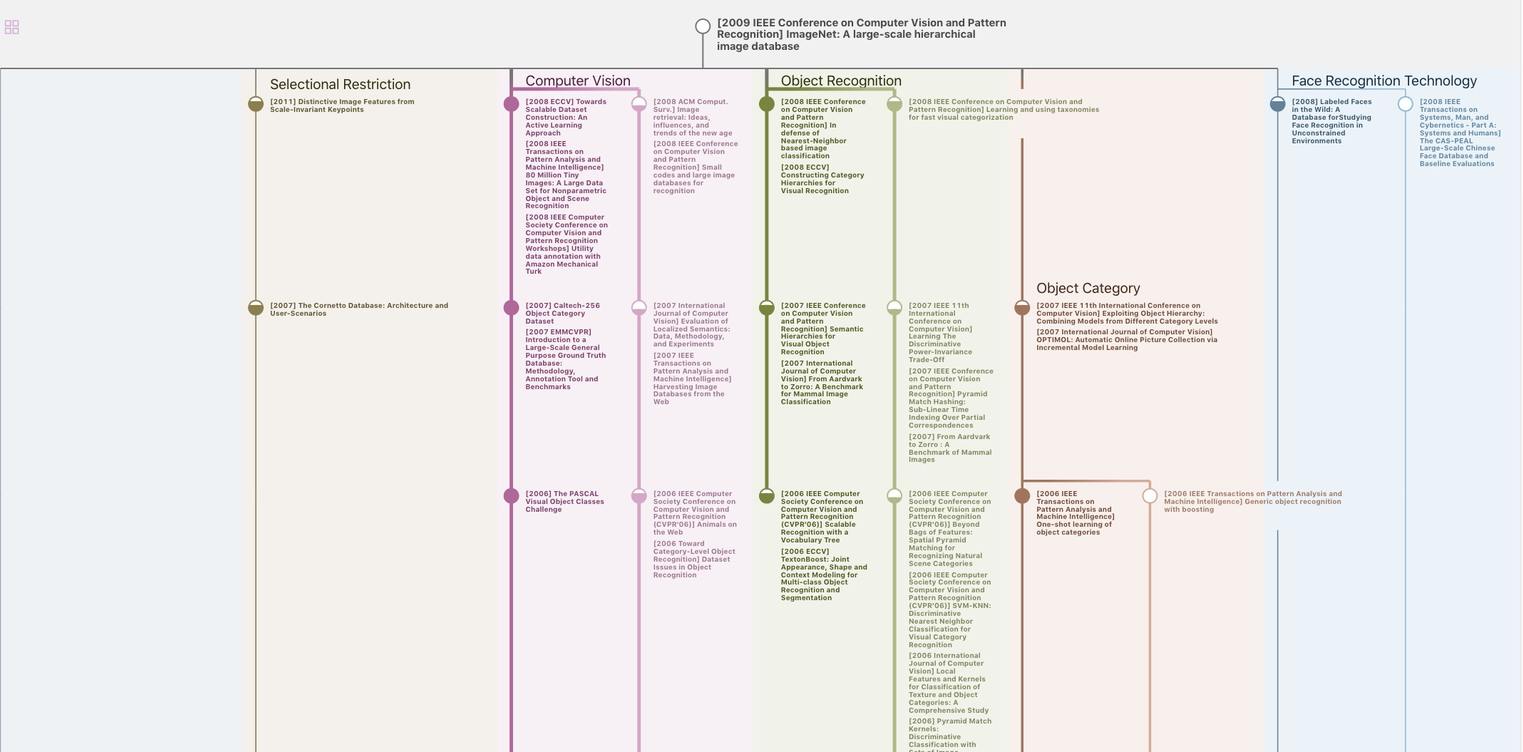
生成溯源树,研究论文发展脉络
Chat Paper
正在生成论文摘要