Continual Learning for Activity Recognition.
Annual International Conference of the IEEE Engineering in Medicine and Biology Society (EMBC)(2022)
摘要
The recent success of deep neural networks in prediction tasks on wearable sensor data is evident. However, in more practical online learning scenarios, where new data arrive sequentially, neural networks suffer severely from the "catastrophic forgetting" problem. In real-world settings, given a pre-trained model on the old data, when we collect new data, it is practically infeasible to re-train the model on both old and new data because the computational costs will increase dramatically as more and more data arrive in time. However, if we fine-tune the model only with the new data because the new data might be different from the old data, the neural network parameters will change to fit the new data. As a result, the new parameters are no longer suitable for the old data. This phenomenon is known as catastrophic forgetting, and continual learning research aims to overcome this problem with minimal computational costs. While most of the continual learning research focuses on computer vision tasks, implications of catastrophic forgetting in wearable computing research and potential avenues to address this problem have remained unexplored. To address this knowledge gap, we study continual learning for activity recognition using wearable sensor data. We show that the catastrophic forgetting problem is a critical challenge for real-world deployment of machine learning models for wearables. Moreover, we show that the catastrophic forgetting problem can be alleviated by employing various training techniques.
更多查看译文
关键词
Education, Distance,Knowledge,Machine Learning,Neural Networks, Computer,Recognition, Psychology
AI 理解论文
溯源树
样例
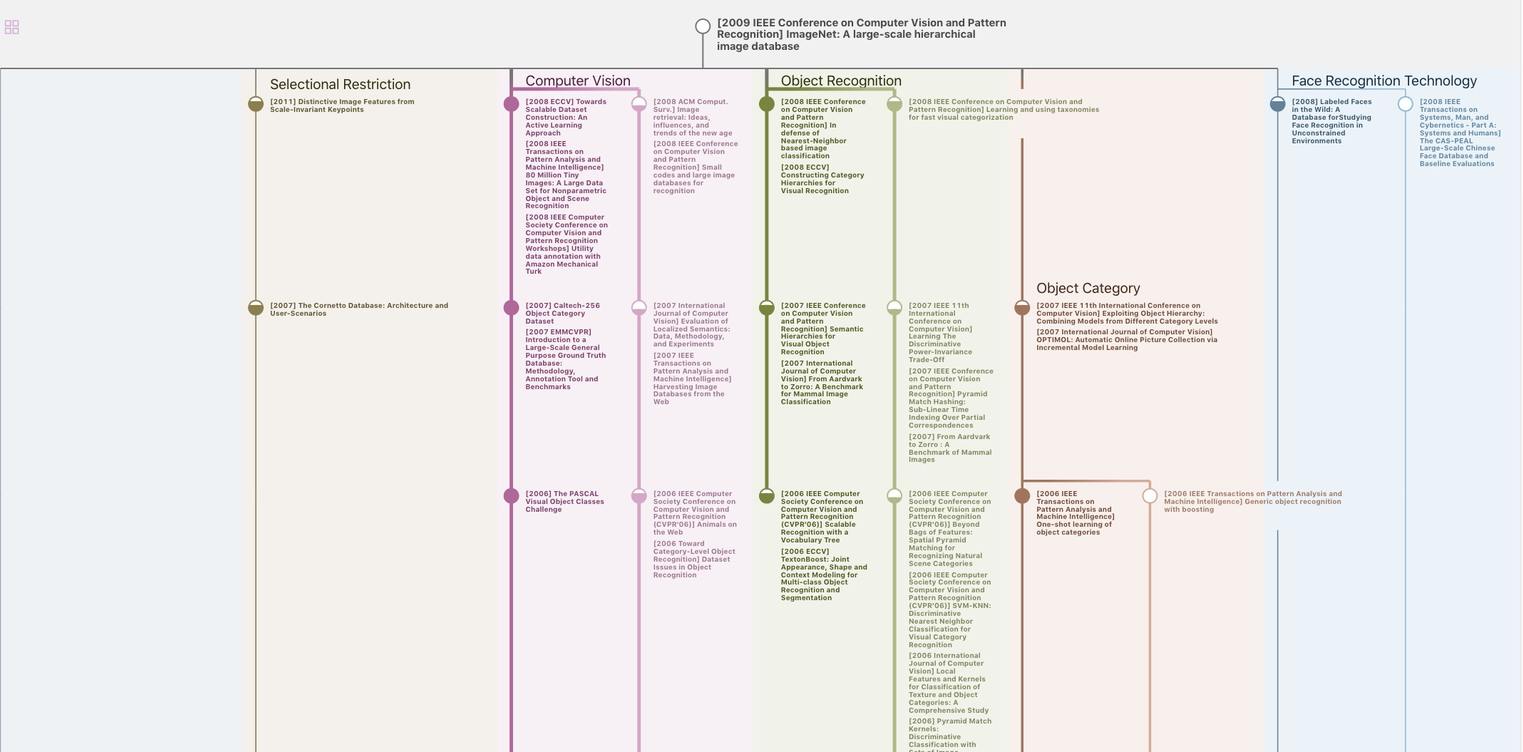
生成溯源树,研究论文发展脉络
Chat Paper
正在生成论文摘要