Detecting Obstructive Apnea Episodes using Dynamic Bayesian Networks and ECG-based Time-Series.
Annual International Conference of the IEEE Engineering in Medicine and Biology Society (EMBC)(2022)
摘要
In this study, we proposed an automatic detector for obstructive apnea episodes using only ECG-based time-series from a single-ECG channel. Several obstructive apnea episodes were provoked for different separated sequences of 15 minutes in anesthetized Sprague-Dawley rats. In this recurrent obstructive sleep apnea (OSA) model, each episode lasted 15s, while the number of total events per sequence was randomly selected. The beat-to-beat interval ( RR) and the R-wave amplitude ( Ra) time-series were extracted and processed for each sequence, and used to train Dynamic Bayesian Networks with different lags. An optimal trade-off between the lag ( L) and RMSE values was considered to select the best model to be used when detecting apnea episodes. The selected models were then used to estimate the occurrence probability of apnea episodes, p(A), by using a filtering approach. Finally, the time-series of the estimated probabilities were post-processed using non-overlapped 15-s epochs, to determine whether they are classified as apneic or non-apneic segments. Results showed that those lagged models with orders greater than 5, presented suitable RMSE values and become more sensitive as the order increased. A detection threshold of 0.2 seems to provide the best apnea detection performance overall, with Acc=0.81, Se=0.83 and Sp=0.79, using two ECG parameters and L=10. Clinical relevance- Dynamic Bayesian Networks represent a powerful tool to develop personalized models for apnea detection and diagnosis in OSA patients.
更多查看译文
关键词
Airway Obstruction,Animals,Bayes Theorem,Electrocardiography,Rats,Rats, Sprague-Dawley,Sleep Apnea Syndromes,Sleep Apnea, Obstructive
AI 理解论文
溯源树
样例
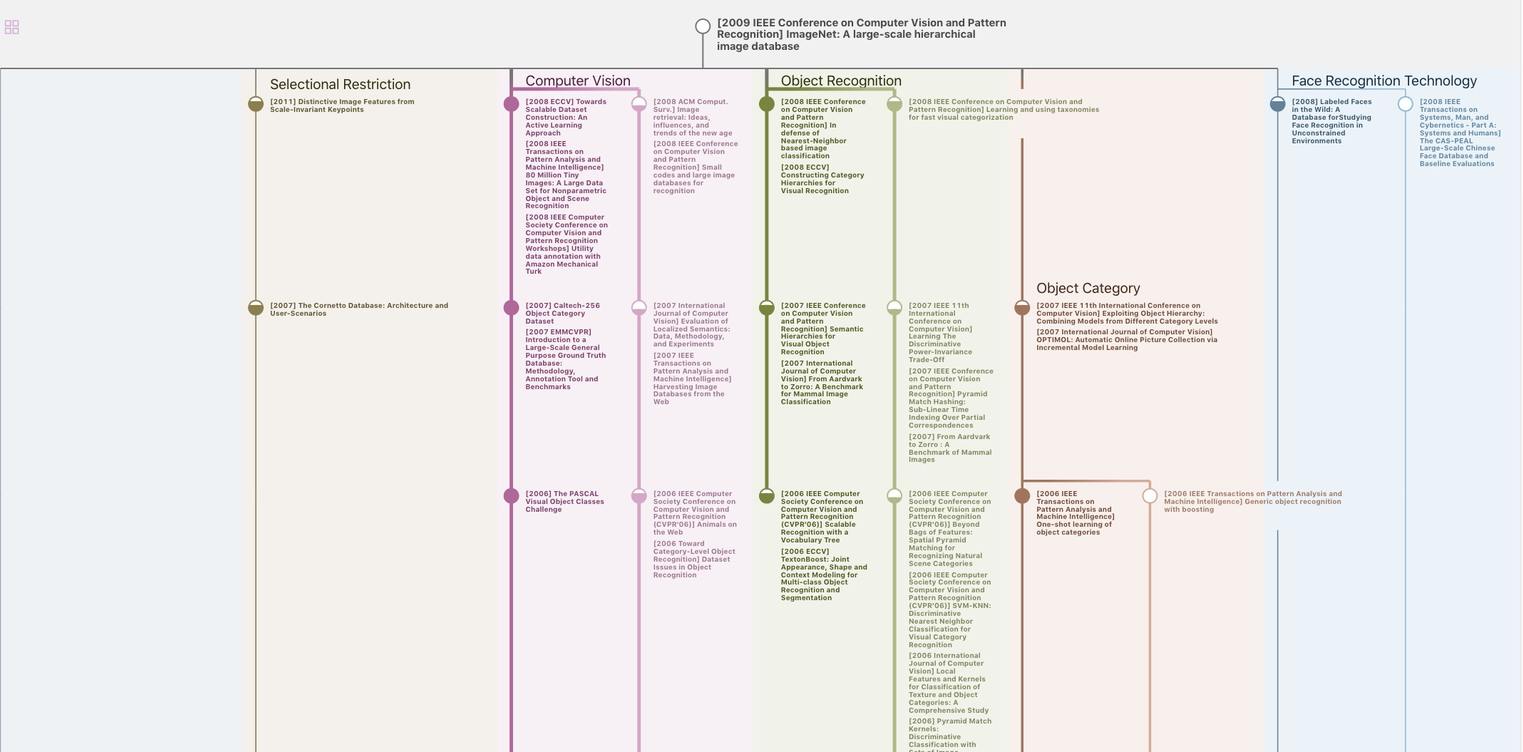
生成溯源树,研究论文发展脉络
Chat Paper
正在生成论文摘要