Improving Autism Spectrum Disorder Prediction by Fusion of Multiple Measures of Resting-State Functional MRI Data
Annual International Conference of the IEEE Engineering in Medicine and Biology Society (EMBC)(2022)
摘要
Autism spectrum disorder (ASD) is a lifelong neurodevelopmental condition characterized by social communication, language and behavior impairments. Leveraging deep learning to automatically predict ASD has attracted more and more attention in the medical and machine learning communities. However, how to select effective measure signals for deep learning prediction is still a challenging problem. In this paper, we studied two kinds of measure signals, i.e., regional homogeneity (ReHo) and Craddock 200 (CC200), which both represents homogeneous functional activity, in the framework of deep learning, and designed a new mechanism to effectively joint them for deep learning based ASD prediction. Extensive experiments on the ABIDE dataset provide empirical evidence in support of effectiveness of our method. In particular, we obtained 79% in terms of accuracy by effectively fusing these two kinds of signals, much better than any single-measure model (ReHo SM-model: ∼69% and CC200 SM-model: ∼70%). These results suggest that leveraging multi-measure signals together are effective for ASD prediction.
更多查看译文
关键词
autism spectrum disorder prediction,mri,multiple measures,resting-state
AI 理解论文
溯源树
样例
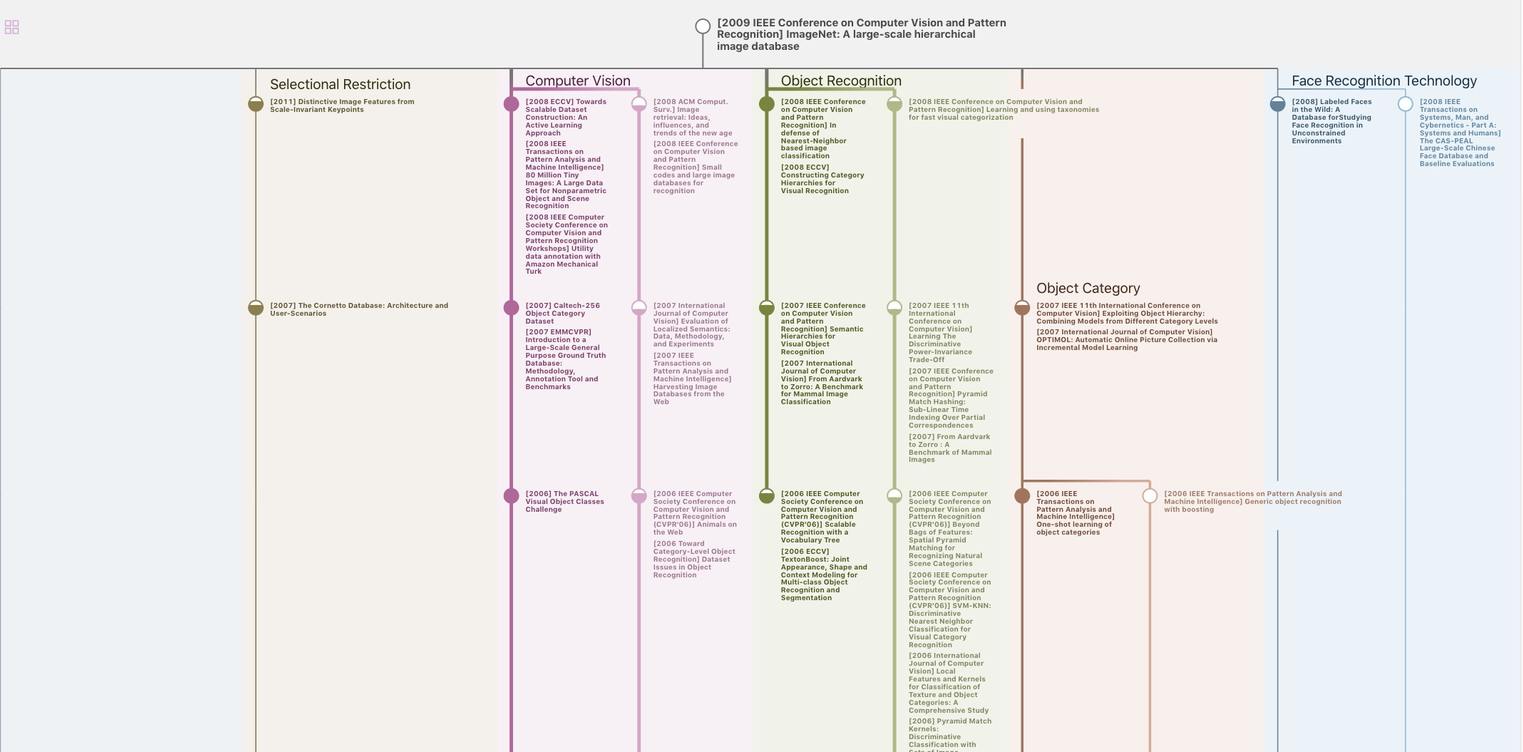
生成溯源树,研究论文发展脉络
Chat Paper
正在生成论文摘要