Toward data lakes as central building blocks for data management and analysis
FRONTIERS IN BIG DATA(2022)
摘要
Data lakes are a fundamental building block for many industrial data analysis solutions and becoming increasingly popular in research. Often associated with big data use cases, data lakes are, for example, used as central data management systems of research institutions or as the core entity of machine learning pipelines. The basic underlying idea of retaining data in its native format within a data lake facilitates a large range of use cases and improves data reusability, especially when compared to the schema-on-write approach applied in data warehouses, where data is transformed prior to the actual storage to fit a predefined schema. Storing such massive amounts of raw data, however, has its very own challenges, spanning from the general data modeling, and indexing for concise querying to the integration of suitable and scalable compute capabilities. In this contribution, influential papers of the last decade have been selected to provide a comprehensive overview of developments and obtained results. The papers are analyzed with regard to the applicability of their input to data lakes that serve as central data management systems of research institutions. To achieve this, contributions to data lake architectures, metadata models, data provenance, workflow support, and FAIR principles are investigated. Last, but not least, these capabilities are mapped onto the requirements of two common research personae to identify open challenges. With that, potential research topics are determined, which have to be tackled toward the applicability of data lakes as central building blocks for research data management.
更多查看译文
关键词
data lake, data analytics, research data management, provenance, big data, FAIR
AI 理解论文
溯源树
样例
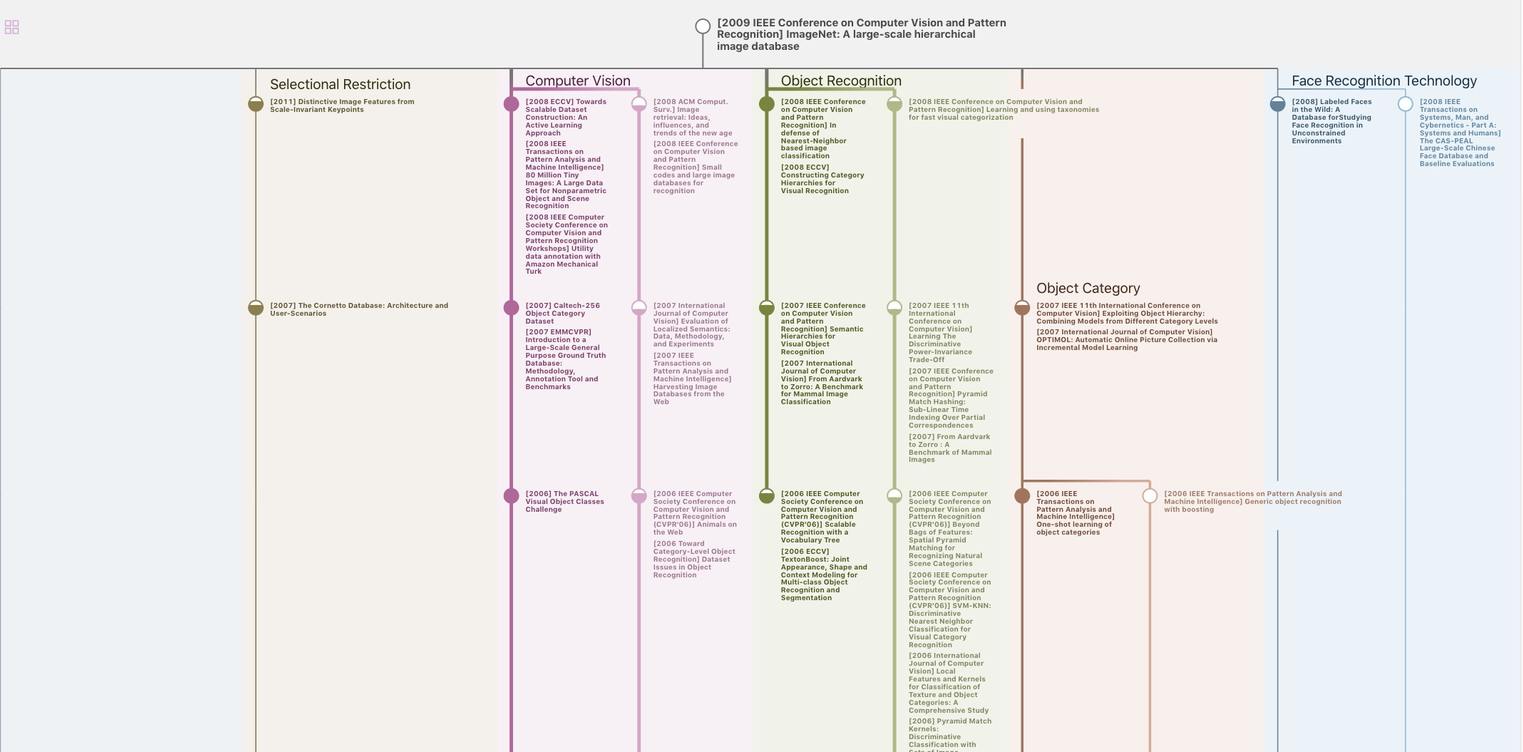
生成溯源树,研究论文发展脉络
Chat Paper
正在生成论文摘要