Efficient Approach for Anomaly Detection in Internet of Things Traffic Using Deep Learning
Wireless Communications and Mobile Computing(2022)
摘要
The network intrusion detection system (NIDs) is a significant research milestone in information security. NIDs can scan and analyze the network to detect an attack or anomaly, which may be a continuing intrusion or perhaps an intrusion that has just occurred. During the pandemic, cybercriminals realized that home networks lurked with vulnerabilities due to a lack of security and computational limitations. A fundamental difficulty in NIDs is providing an effective, robust, lightweight, and rapid framework to perform real-time intrusion detection. This research proposes an efficient, functional cybersecurity approach based on machine/deep learning algorithms to detect anomalies using lightweight network-based IDs. A lightweight, real-time, network-based anomaly detection system can be used to secure connected IoT devices. The UNSW-NB15 dataset is used to evaluate the proposed approach DeepNet and compare results alongside other state-of-the-art existing techniques. For the classification of network-based anomalies, the proposed model achieves 99.16% accuracy by using all features and 99.14% accuracy after feature reduction. The experimental results show that the network anomalies depend exceptionally on features selected after selection.
更多查看译文
关键词
anomaly detection,deep learning,things traffic
AI 理解论文
溯源树
样例
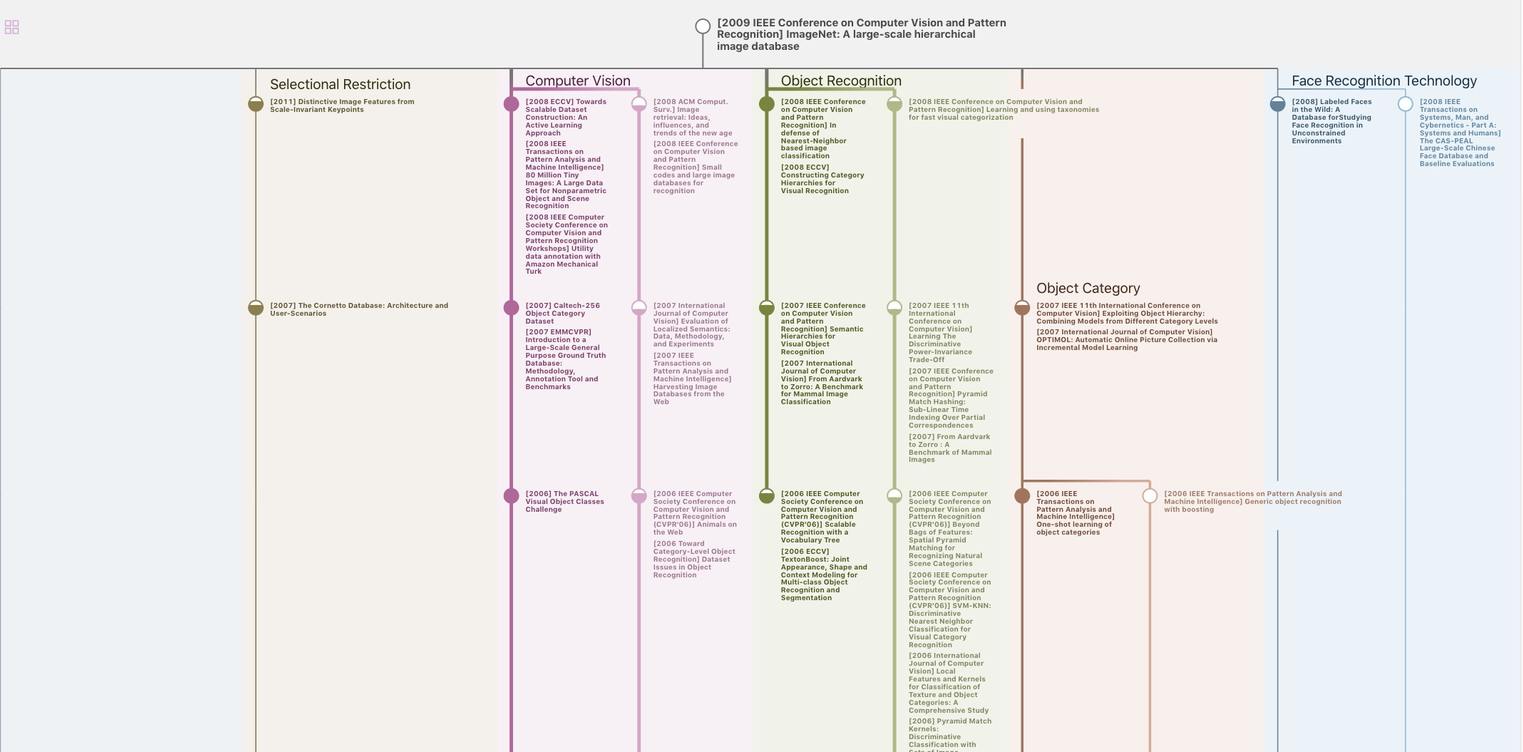
生成溯源树,研究论文发展脉络
Chat Paper
正在生成论文摘要