End-to-End Learning of User Equilibrium with Implicit Neural Networks
SSRN Electronic Journal(2022)
摘要
This paper intends to transform the transportation network equilibrium modeling paradigm via an “end-to-end” framework that directly learns travel choice preferences and the equilibrium state from multi-day link flow observations. The centerpiece of the proposed framework is to use deep neural networks to represent travelers’ route choice preferences and then encapsulate the neural networks in a variational inequality that prescribes the user equilibrium flow distribution. The proposed neural network architecture ensures the existence of equilibrium and accommodates future changes in road network topology. The variational inequality is then embedded as an implicit layer in a learning framework, which takes the context features (e.g., road network and traveler characteristics) as input and outputs the user equilibrium flow distribution. By comparing computed equilibrium flows with observed flows, the neural networks can be trained. The proposed end-to-end framework is demonstrated and validated using synthesized data for the Sioux Falls network.
更多查看译文
关键词
Network equilibrium,Neural-network-based variational inequality,End-to-end learning,Implicit layer
AI 理解论文
溯源树
样例
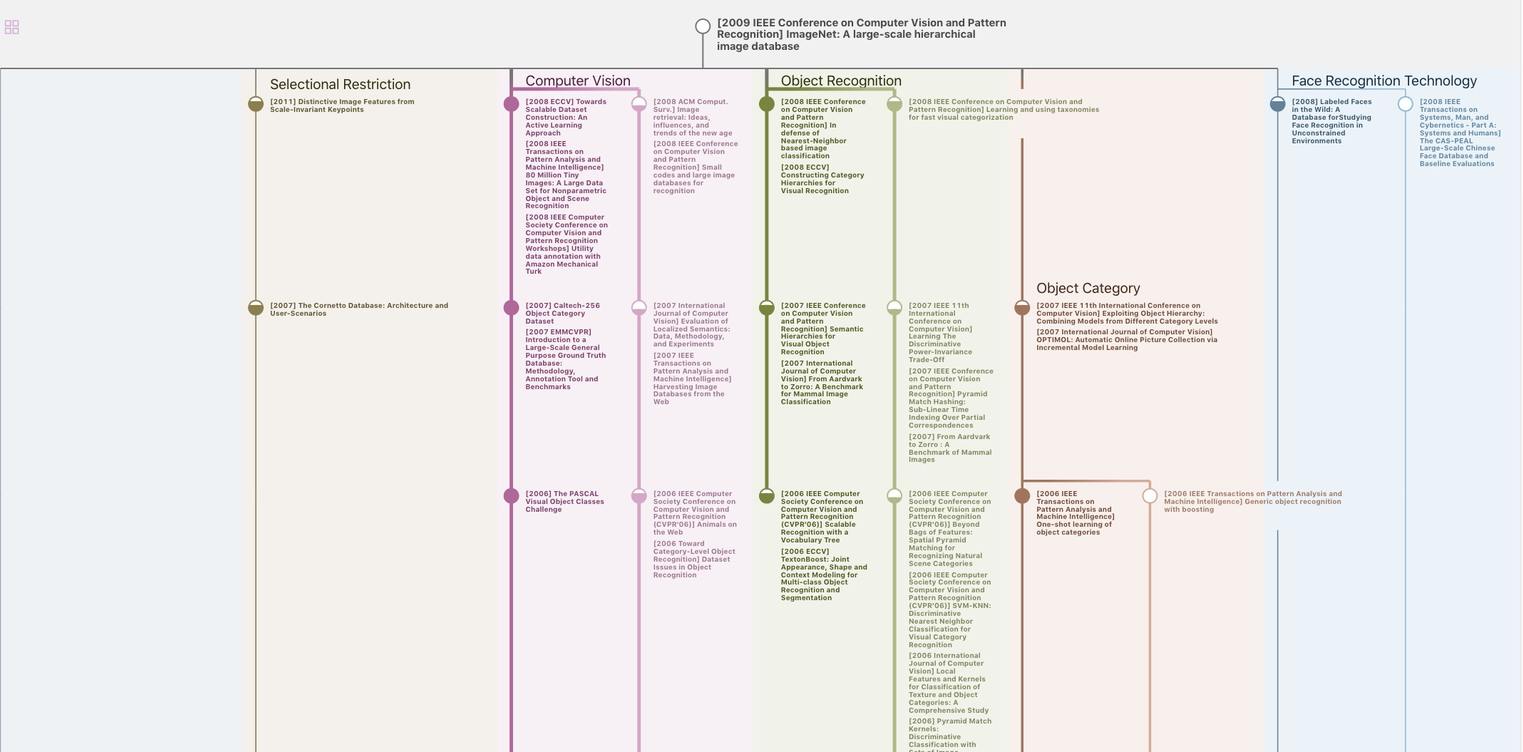
生成溯源树,研究论文发展脉络
Chat Paper
正在生成论文摘要