k-outlier removal based on contextual label information and cluster purity for continuous data classification
EXPERT SYSTEMS WITH APPLICATIONS(2024)
摘要
Outlier detection is extensively adopted in machine learning applications to identify rare but significant objects in a data distribution that deviate from the majority of objects. However, none of the existing outlier definitions use the available contextual label information in a dataset to improve the identification of outliers, though it could improve the performance of data analysis. In this study, we propose a novel definition for outliers considering the available contextual label information along with a method to remove outliers based on the proposed definition to improve classification performance and granulation purity. The experimental results on eight public datasets show that the removal of outliers using the novel method highly improves the classification accuracy with Support Vector Machine, k-Nearest Neighbors, and Classification and Regression Tree algorithms while identifying 100% pure subclasses of the labeled major classes. This method is beneficial in identifying the outliers automatically when the data contains classification information about a specific application instead of outlierness information.
更多查看译文
关键词
Outlier removal,Contextual information,Purity,Continuous data,Neighborhood rough set,Granules
AI 理解论文
溯源树
样例
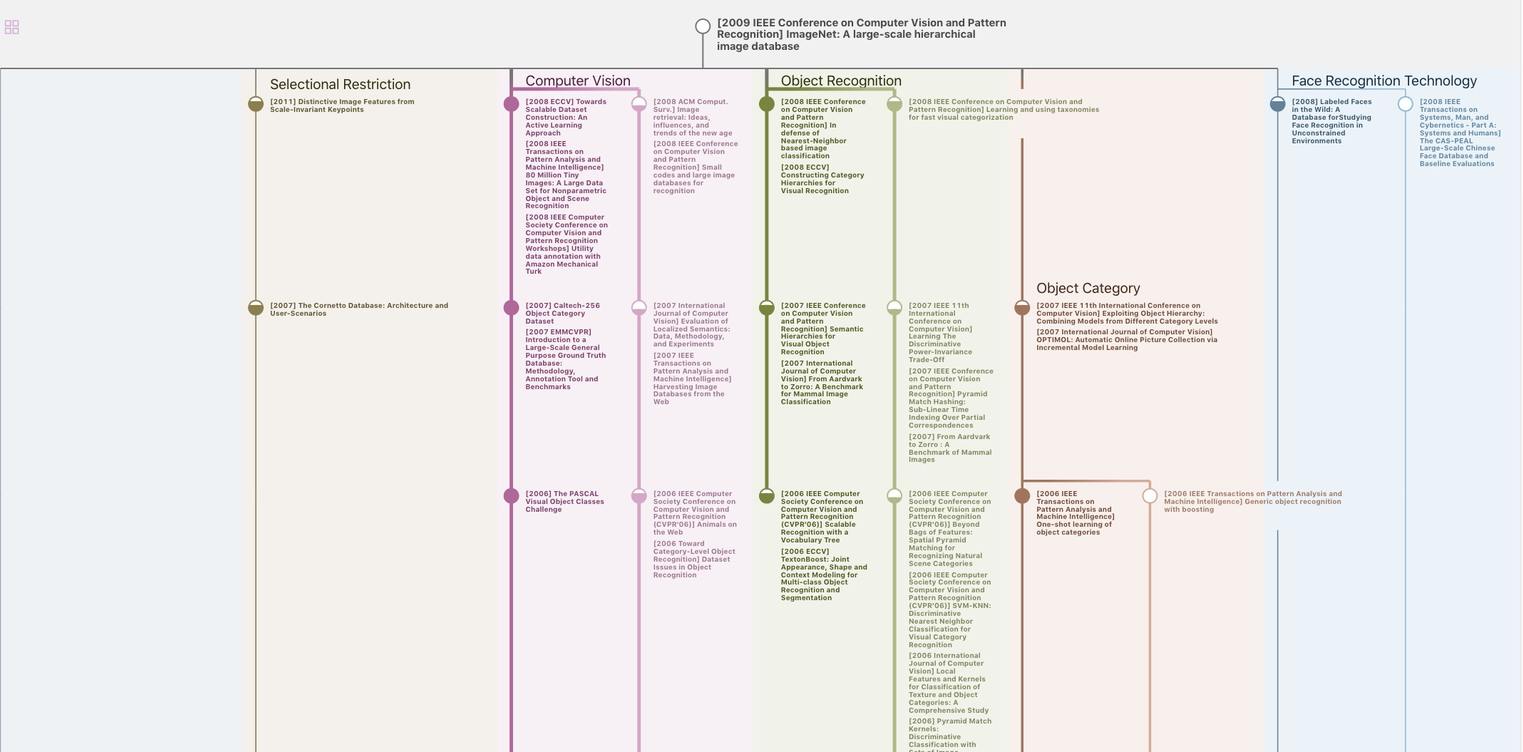
生成溯源树,研究论文发展脉络
Chat Paper
正在生成论文摘要