DRT-Unet: A Segmentation Network for Aiding Brain Tumor Diagnosis
Security and Communication Networks(2022)
摘要
Using image segmentation techniques to assist physicians in brain tumor diagnosis is a hot issue in computer technology research. Although most brain tumor segmentation networks to date have been based on U-Net, the prediction results are depending on which are not well generalized and need to be further improved. As the depth of the network increases, the gradients of the network vanish together with the decrease of the accuracy; meanwhile, the large number of parameters in the network will cause data redundancy. Moreover, a single modality of MRI images cannot adequately segment tumor details. Therefore, a segmentation network with an improved U-Net model is proposed in this paper, which combines Dilated Convolution-Dense Block-Transformation Convolution-Unet (hereafter referred to as DRT-Unet). The network adopts the combination of dilated convolution, dense residual block, and transposed convolution. In the coding process, a dilated convolution block and a local feature residual for fusing dense block are adopted to replace the 3 × 3 convolution layers on each layer in U-Net, and a transition layer is used for down-sampling. In the decoding process, a local feature residual is adopted for fusing dense blocks; meanwhile, a deconvolution structure with up-pooling and transposed convolution cascade is used. By connecting the decoded output features with the encoded low-level visual features, the information on transition layer loss is obtained. The experiments in this paper are carried out on BraTs2018 and BraTs2019 datasets; as a result, the DRT-Unet network can effectively segment tumor lesion regions.
更多查看译文
关键词
segmentation network,tumor,drt-unet
AI 理解论文
溯源树
样例
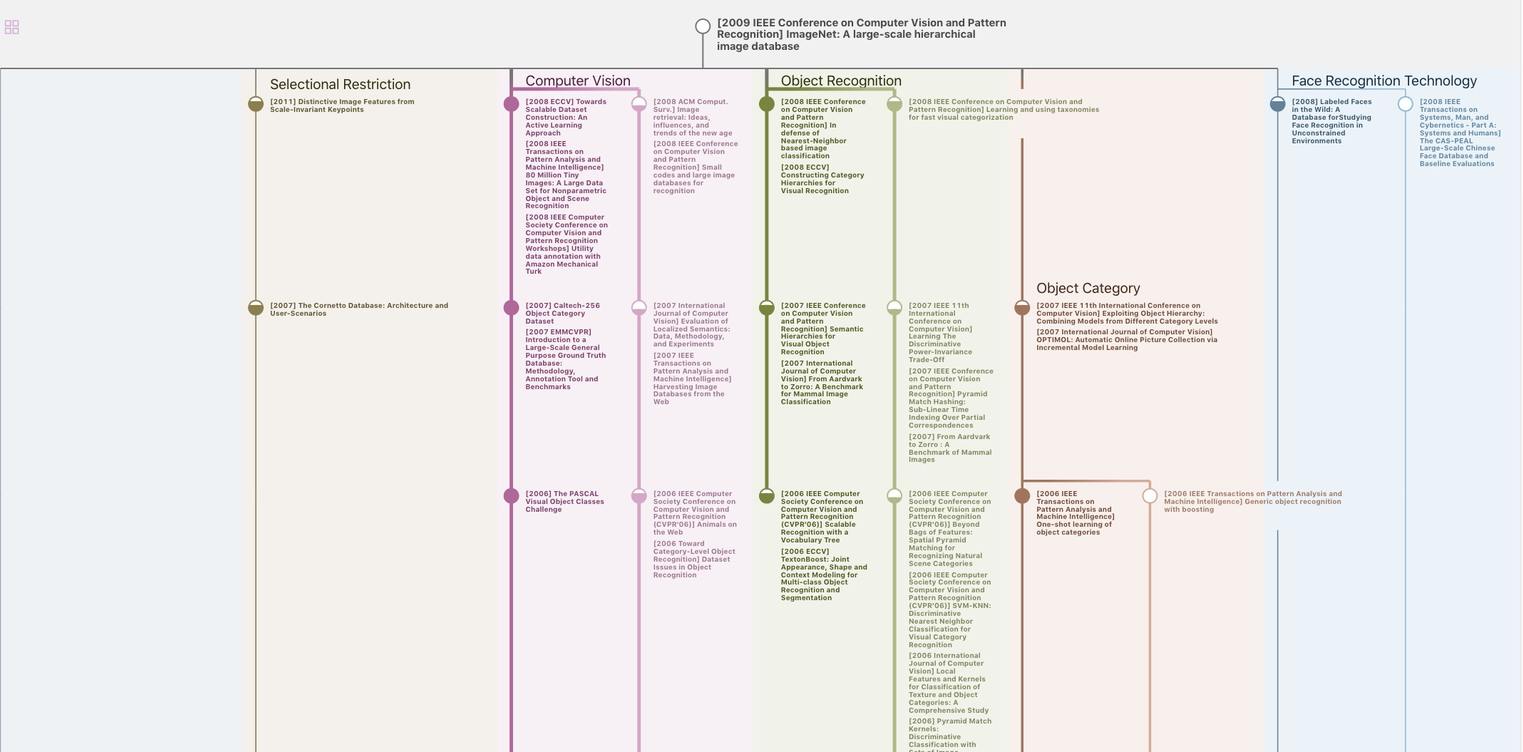
生成溯源树,研究论文发展脉络
Chat Paper
正在生成论文摘要