Optimal output regulation for unknown continuous-time linear systems by internal model and adaptive dynamic programming
Automatica(2022)
摘要
This paper addresses an optimal output regulation problem for linear time-invariant systems with unknown dynamics. First, a new augmented virtual system is designed to replace the original system and the internal model. Then, by incorporating the data from the augmented virtual system with adaptive dynamic programming (ADP) method, a new iterative learning equation without requiring integral operations or constructing internal model in advance is proposed for establishing the data-driven learning algorithm. Compared with existing ADP-based learning algorithms for linear continuous-time systems, the proposed learning algorithm relaxes both requirements on recording the complete continuous data and setting an initial stabilizing control policy. Finally, the effectiveness of the proposed algorithm is illustrated by an example.
更多查看译文
关键词
Data-driven learning method,Internal model,Optimal output regulation,Virtual system
AI 理解论文
溯源树
样例
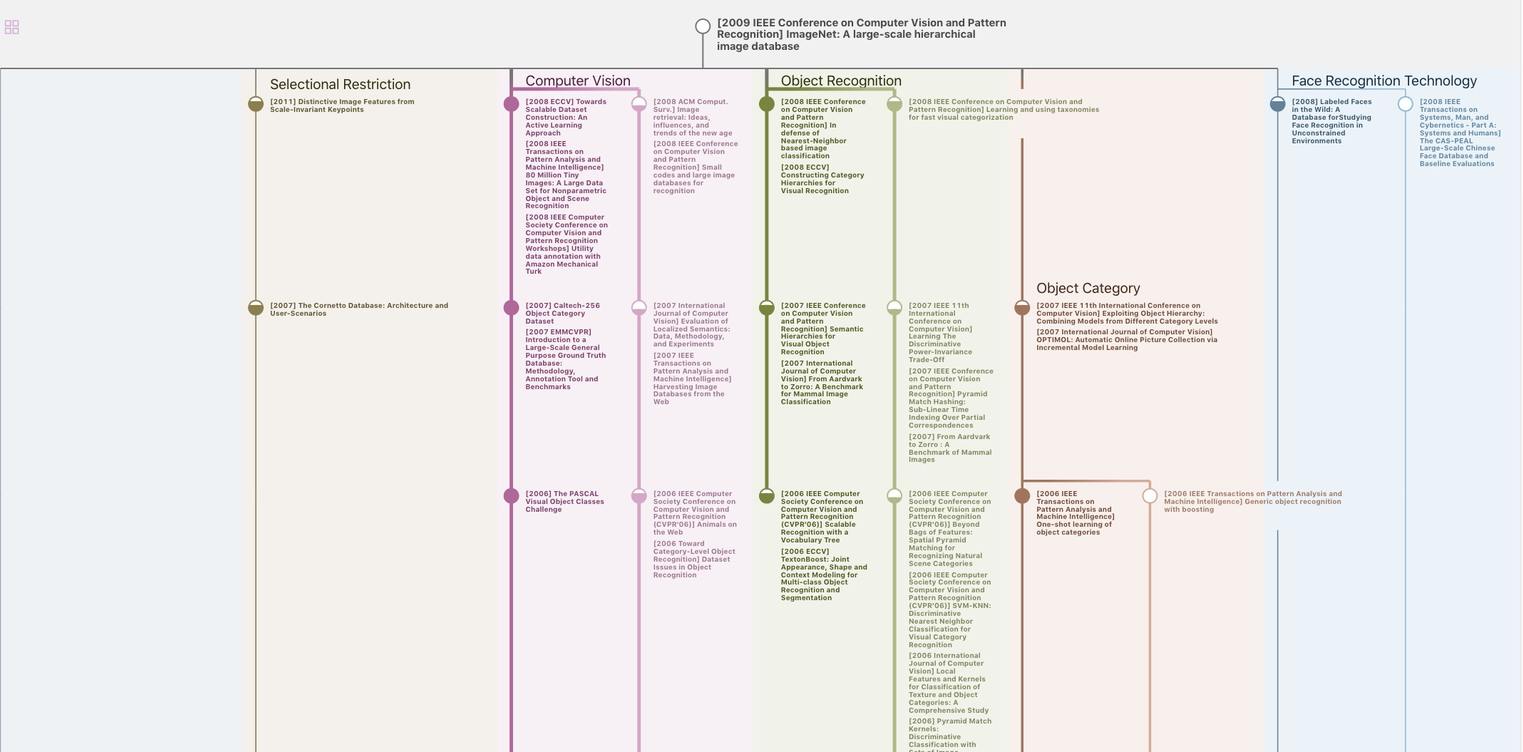
生成溯源树,研究论文发展脉络
Chat Paper
正在生成论文摘要