Towards bridging the distribution gap: Instance to Prototype Earth Mover’s Distance for distribution alignment
Medical Image Analysis(2022)
摘要
Despite remarkable success of deep learning, distribution divergence remains a challenge that hinders the performance of many tasks in medical image analysis. Large distribution gap may deteriorate the knowledge transfer across different domains or feature subspaces. To achieve better distribution alignment, we propose a novel module named Instance to Prototype Earth Mover’s Distance (I2PEMD), where shared class-specific prototypes are progressively learned to narrow the distribution gap across different domains or feature subspaces, and Earth Mover’s Distance (EMD) is calculated to take into consideration the cross-class relationships during embedding alignment. We validate the effectiveness of the proposed I2PEMD on two different tasks: multi-modal medical image segmentation and semi-supervised classification. Specifically, in multi-modal medical image segmentation, I2PEMD is explicitly utilized as a distribution alignment regularization term to supervise the model training process, while in semi-supervised classification, I2PEMD works as an alignment measure to sort and cherry-pick the unlabeled data for more accurate and robust pseudo-labeling. Results from comprehensive experiments demonstrate the efficacy of the present method.
更多查看译文
关键词
Distribution alignment,Instance to prototype matching,Earth Mover’s Distance,Unpaired multi-modal segmentation,Semi-supervised classification
AI 理解论文
溯源树
样例
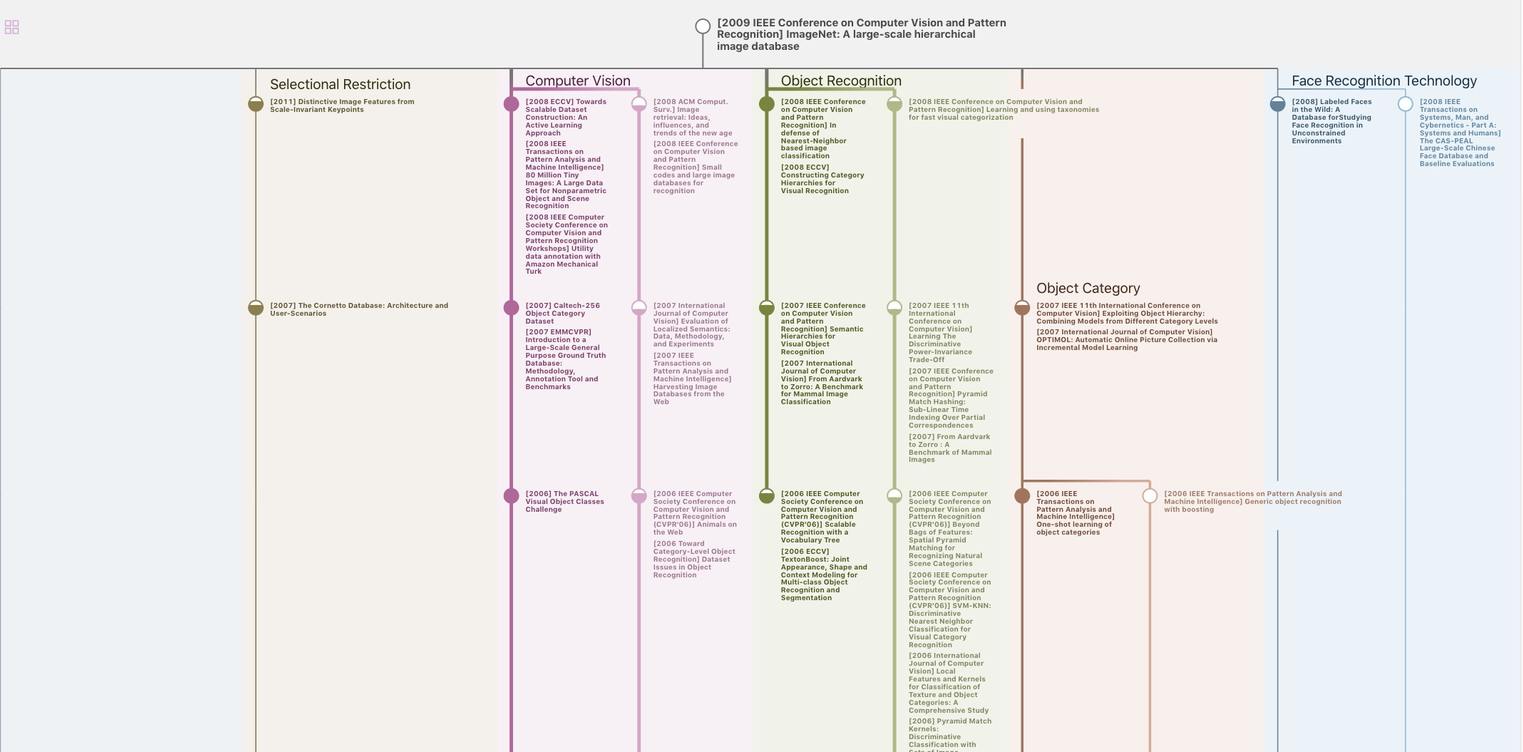
生成溯源树,研究论文发展脉络
Chat Paper
正在生成论文摘要