Learning All-In Collaborative Multiview Binary Representation for Clustering
IEEE TRANSACTIONS ON NEURAL NETWORKS AND LEARNING SYSTEMS(2024)
摘要
Multiview clustering via binary representation has attracted intensive attention due to its effectiveness in handling large-scale multiple view data. However, these kind of clustering approaches usually ignore a very important potential high-order correlation in discrete representation learning. In this article, we propose a novel all-in collaborative multiview binary representation for clustering (AC-MVBC) framework, where multiview collaborative binary representation and clustering structure are learned in a joint manner. Specifically, using a new type of tensor low-rank constraint, the high-order collaborations, i.e., cross-view and inner view collaborations, can be effectively captured in our model. Moreover, by incorporating the Bregman discrepancy, the projective consistency among different views can be guaranteed to achieve a more powerful binary representation. An efficient optimization algorithm is also proposed to solve the objective function with fast convergence empirically. Experimental results on several challenge datasets demonstrate that the proposed method has achieved highly competent performance compared with the state-of-the-art multiview clustering (MVC) methods while maintaining low computational and memory requirements.
更多查看译文
关键词
Collaboration,Tensors,Costs,Binary codes,Encoding,Task analysis,Optimization,All-in collaborative,binary representation,Bregman discrepancy,joint learning,multiview clustering (MVC),tensor low-rank constraint
AI 理解论文
溯源树
样例
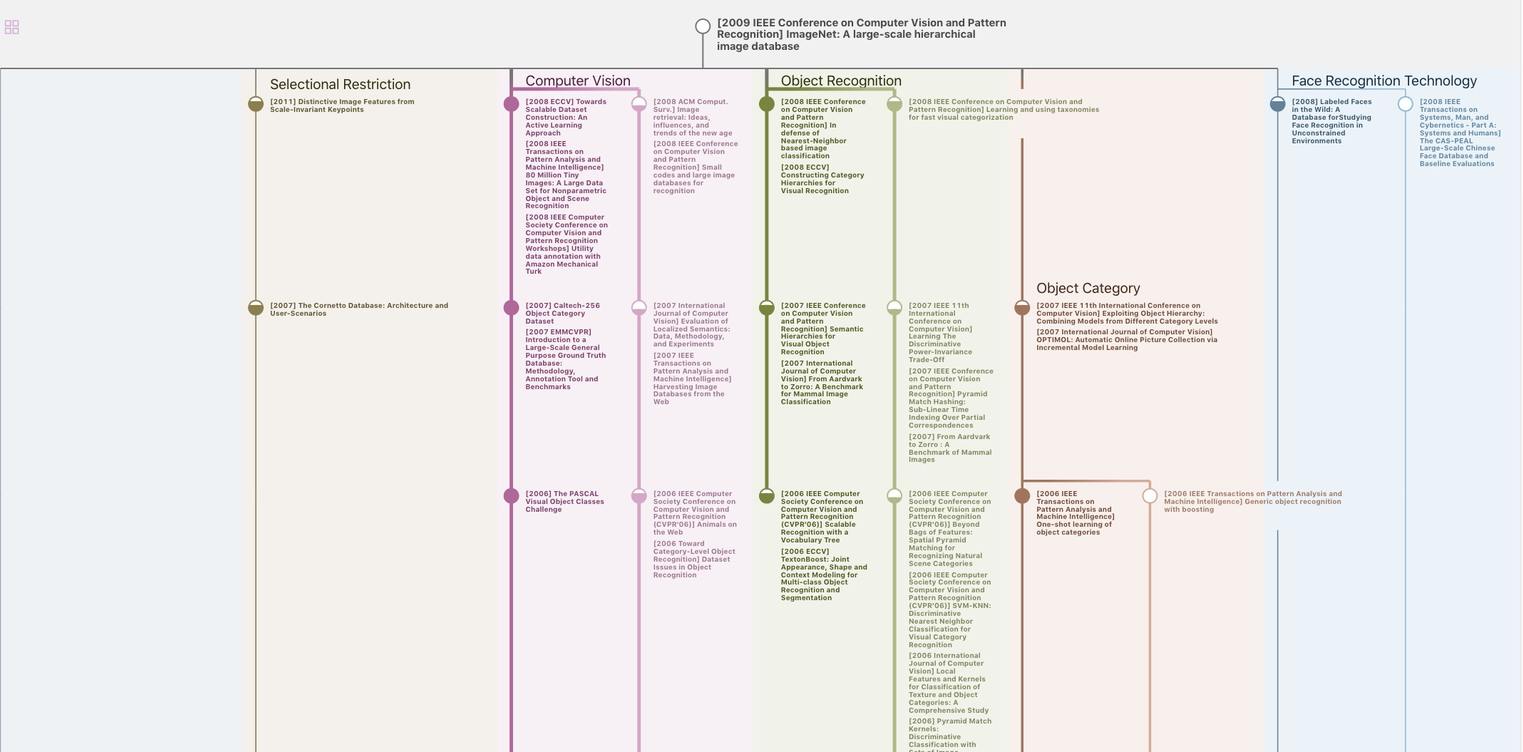
生成溯源树,研究论文发展脉络
Chat Paper
正在生成论文摘要