An Object Point Set Inductive Tracker for Multi-Object Tracking and Segmentation
IEEE TRANSACTIONS ON IMAGE PROCESSING(2022)
摘要
Multi-object tracking and segmentation (MOTS) is a derivative task of multi-object tracking (MOT). The new setting encourages the learning of more discriminative high-quality embeddings. In this paper, we focus on the problem of exploring the relationship between the segmenter and the tracker, and propose an efficient Object Point set Inductive Tracker (OPITrack) based on it. First, we discover that after a single attention layer, the high-dimensional, key point embedding will show feature averaging. To alleviate this phenomenon, we propose an embedding generalization training strategy for sparse training and dense testing. This strategy allows the network to increase randomness in training and encourages the tracker to learn more discriminative features. In addition, to learn the desired embedding space, we propose a general Trip-hard sample augmentation loss. The loss uses patches that are not distinguishable by the segmenter to join the feature learning and force the embedding network to learn the difference between false positives and true positives. Our method was validated on two MOTS benchmark datasets and achieved promising results. In addition, our OPITrack can achieve better performance for the raw model while costing less video memory (VRAM) at training time.
更多查看译文
关键词
Task analysis, Tracking, Image segmentation, Feature extraction, Training, Multitasking, Three-dimensional displays, Multi-object tracking and segmentation, tracking-by-segmentation, high-quality embeddings, robust, multi-object tracking
AI 理解论文
溯源树
样例
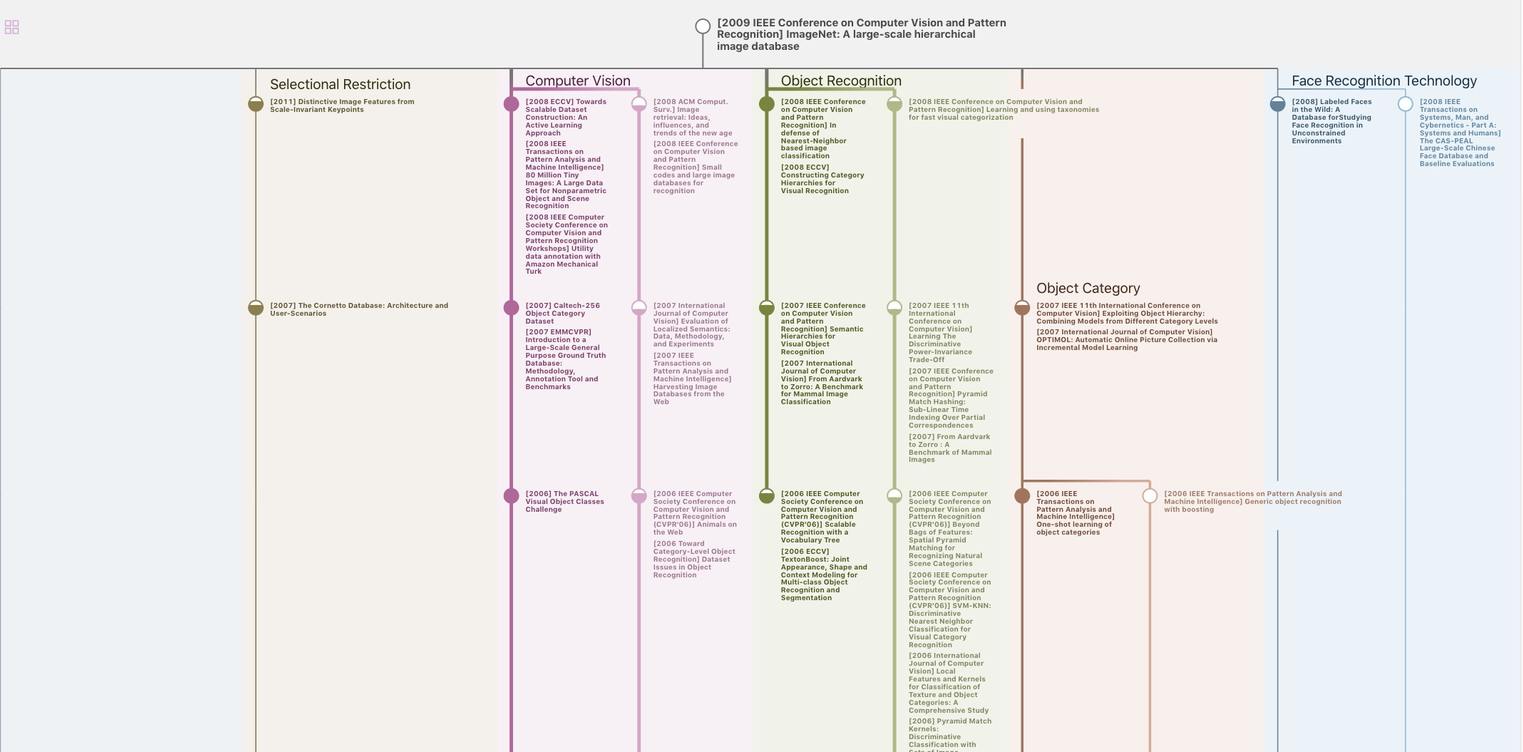
生成溯源树,研究论文发展脉络
Chat Paper
正在生成论文摘要