Systematic Improvement of the Performance of Machine Learning Scoring Functions by Incorporating Features of Protein-Bound Water Molecules
JOURNAL OF CHEMICAL INFORMATION AND MODELING(2022)
摘要
Water molecules at the ligand-protein interfaces play crucial roles in the binding of the ligands, but the behavior of protein-bound water is largely ignored in many currently used machine learning (ML)-based scoring functions (SFs). In an attempt to improve the prediction performance of existing ML-based SFs, we estimated the water distribution with a HydraMap (HM) method and then incorporated the features extracted from protein-bound waters obtained in this way into three ML-based SFs: RFScore, ECIF, and PLEC. It was found that a combination of HM-based features can consistently improve the performance of all three SFs, including their scoring, ranking, and docking power. HydraMap-based features show consistently good performance with both crystal structures and docked structures, demonstrating their robustness for SFs. Overall, HM-based features, which are a statistical representation of hydration sites at protein-ligand interfaces, are expected to improve the prediction performance for diverse SFs.
更多查看译文
关键词
machine learning scoring functions,machine learning,molecules,protein-bound
AI 理解论文
溯源树
样例
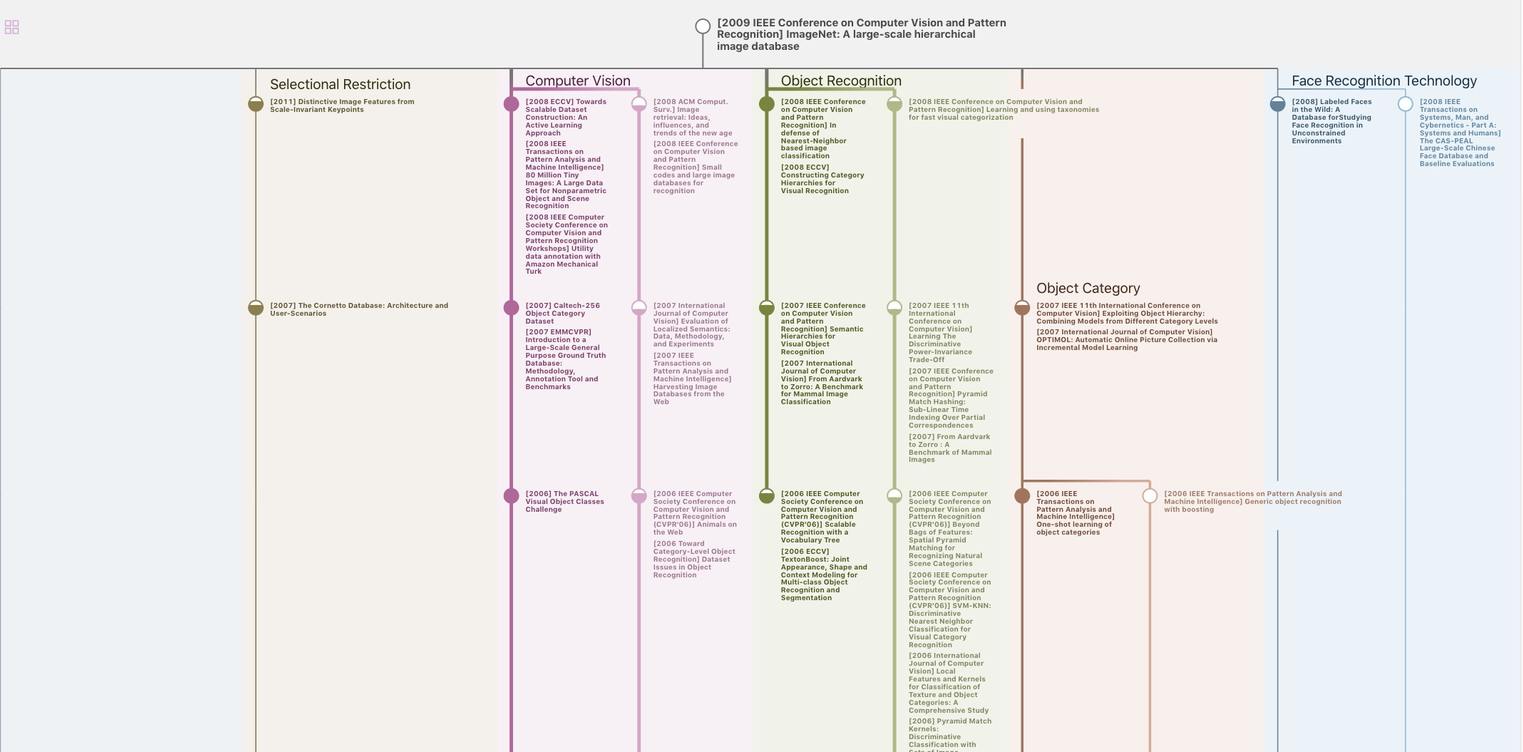
生成溯源树,研究论文发展脉络
Chat Paper
正在生成论文摘要