Small batch deep reinforcement learning
2022 8th International Conference on Big Data and Information Analytics (BigDIA)(2023)
摘要
In value-based deep reinforcement learning with replay memories, the batch size parameter specifies how many transitions to sample for each gradient update. Although critical to the learning process, this value is typically not adjusted when proposing new algorithms. In this work we present a broad empirical study that suggests {\em reducing} the batch size can result in a number of significant performance gains; this is surprising, as the general tendency when training neural networks is towards larger batch sizes for improved performance. We complement our experimental findings with a set of empirical analyses towards better understanding this phenomenon.
更多查看译文
关键词
Reinforcement learning,Deep learning,Strategy function,Cross entropy
AI 理解论文
溯源树
样例
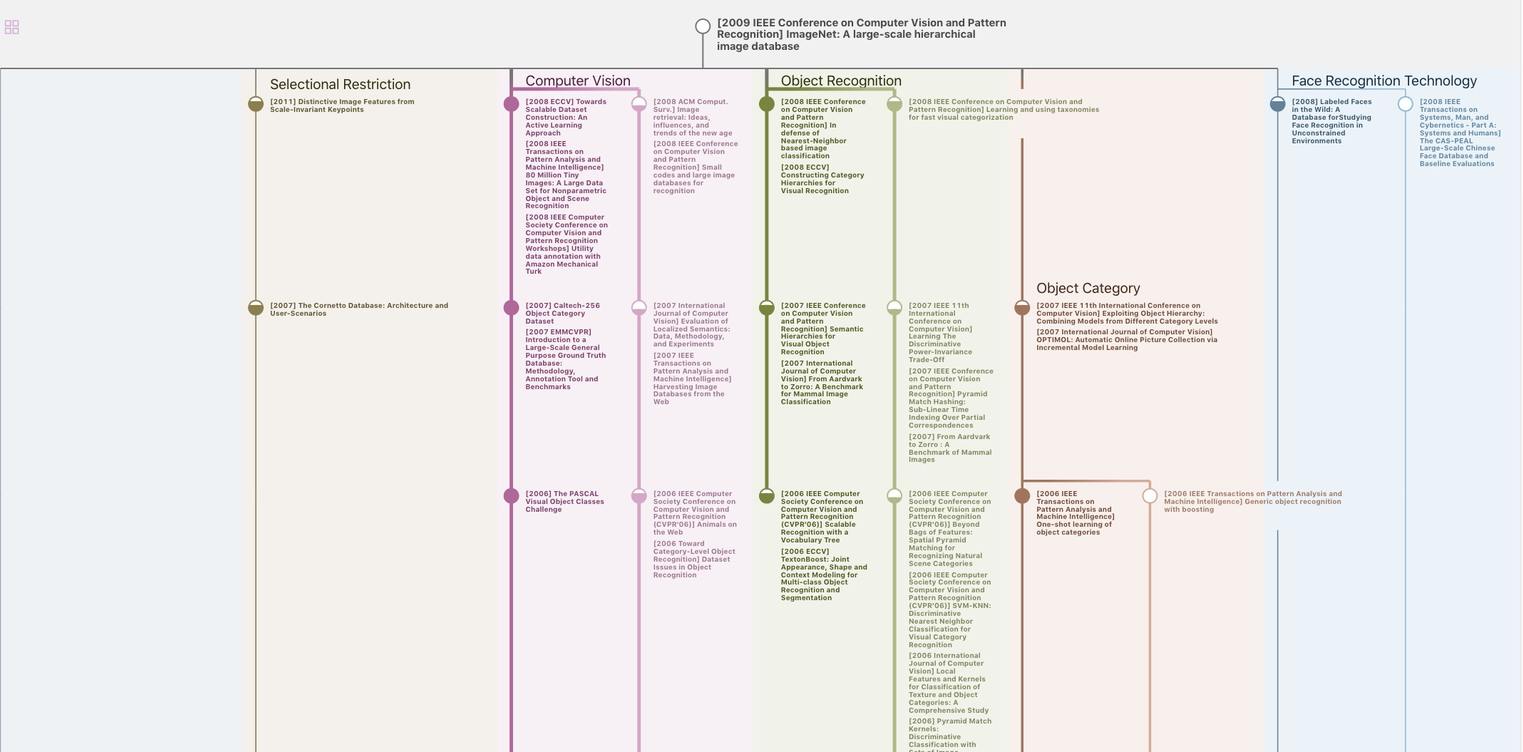
生成溯源树,研究论文发展脉络
Chat Paper
正在生成论文摘要