Data-driven for accelerated design strategy of photocatalytic degradation activity prediction of doped TiO2 photocatalyst
Journal of Water Process Engineering(2022)
摘要
TiO2 photocatalytic degradation, as an efficient, clean technology, is widely used in the treatment of contami-nated wastewater. To expand the absorption spectrum of TiO2 from UV to visible light, considerable effort has been put into the modification of TiO2. Current TiO2 studies still rely on experimental work, mainly focusing on the effects of experimental variables on photocatalytic degradation. However, multiple variables introduce the experimental complexity and increase the cost. As a result, the development of TiO2 could be time-consuming and less cost-effective. Herein, we use a machine learning (ML) approach to study the photocatalytic degrada-tion of doped TiO2. In this study, the degradation rate of pollutant solution in the presence of doped TiO2 is simulated under various experimental conditions using a LightGBM model. The training data from experiments comprised nine inputs, namely dopant, dopant/Ti molar ratio, calcination temperature, pollutant, catalyst/ pollutant mass ratio, pH, experimental temperature, light wavelength and illumination time. The predicted result from trained model shows good accuracy with a coefficient of determination (92.8 %). The importance ranking of these variables, this study may help a better design of TiO2 for wastewater treatment, thereby improving the purification efficiency and saving natural resources.
更多查看译文
关键词
Doped TiO2, Photodegradation of wastewater, Machine learning, LightGBM, Degradation rate
AI 理解论文
溯源树
样例
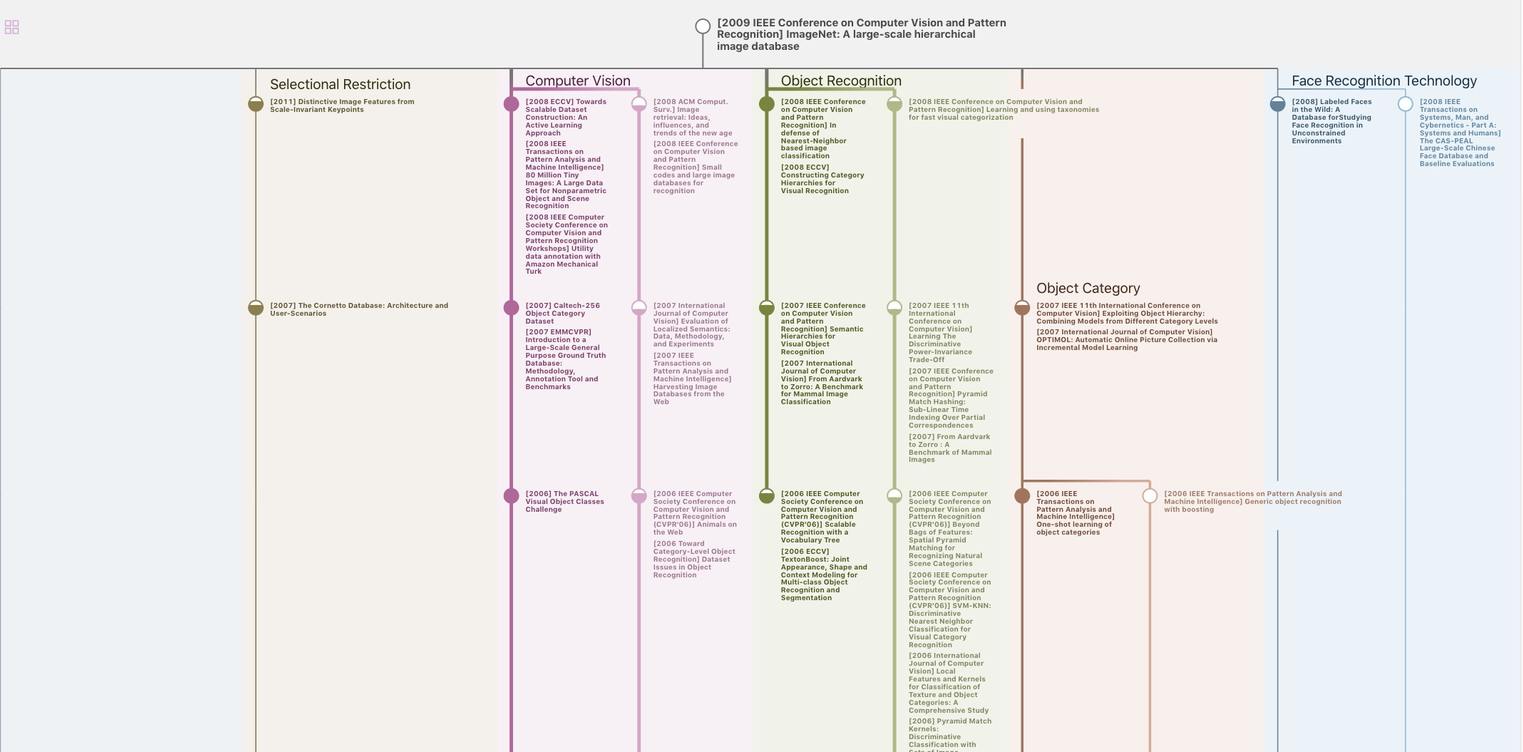
生成溯源树,研究论文发展脉络
Chat Paper
正在生成论文摘要